FDA-FFNet: A Feature-Distance Attention-Based Change Detection Network for Remote Sensing Image
IEEE JOURNAL OF SELECTED TOPICS IN APPLIED EARTH OBSERVATIONS AND REMOTE SENSING(2024)
摘要
Convolutional neural networks have demonstrated remarkable capability in extracting deep semantic features from images, leading to significant advancements in various image processing tasks. This success has also opened up new possibilities for change detection (CD) in remote sensing applications. But unlike the conventional image recognition tasks, the performance of AI models in CD heavily relies on the method used to fuse the features from two different phases of the image. The existing deep-learning-based methods for CD typically fuse features of bitemporal images using difference or concatenation techniques. However, these approaches often fail tails to prioritize potential change areas adequately and neglect the rich contextual information essential for discerning subtle changes, potentially leading to slower convergence speed and reduced accuracy. To tackle this challenge, we propose a novel feature fusion approach called feature-difference attention-based feature fusion CD network. This method aims to enhance feature fusion by incorporating a feature-difference attention-based feature fusion module, enabling a more focused analysis of change areas. Additionally, a deep-supervised attention module is implemented to leverage the deep surveillance module for cascading refinement of change areas. Furthermore, an atrous spatial pyramid pooling fast is employed to efficiently acquire multiscale object information. The proposed method is evaluated on two publicly available datasets, namely the WHU-CD and LEVIR-CD datasets. Compared with the state-of-the-art CD methods, the proposed method outperforms in all metrics, with an intersection over union of 92.49% and 85.56%, respectively.
更多查看译文
关键词
Feature extraction,Image segmentation,Remote sensing,Euclidean distance,Surveillance,Support vector machines,Standards,Attention-based,change detection (CD),deep learning,deep supervision,multiscale feature
AI 理解论文
溯源树
样例
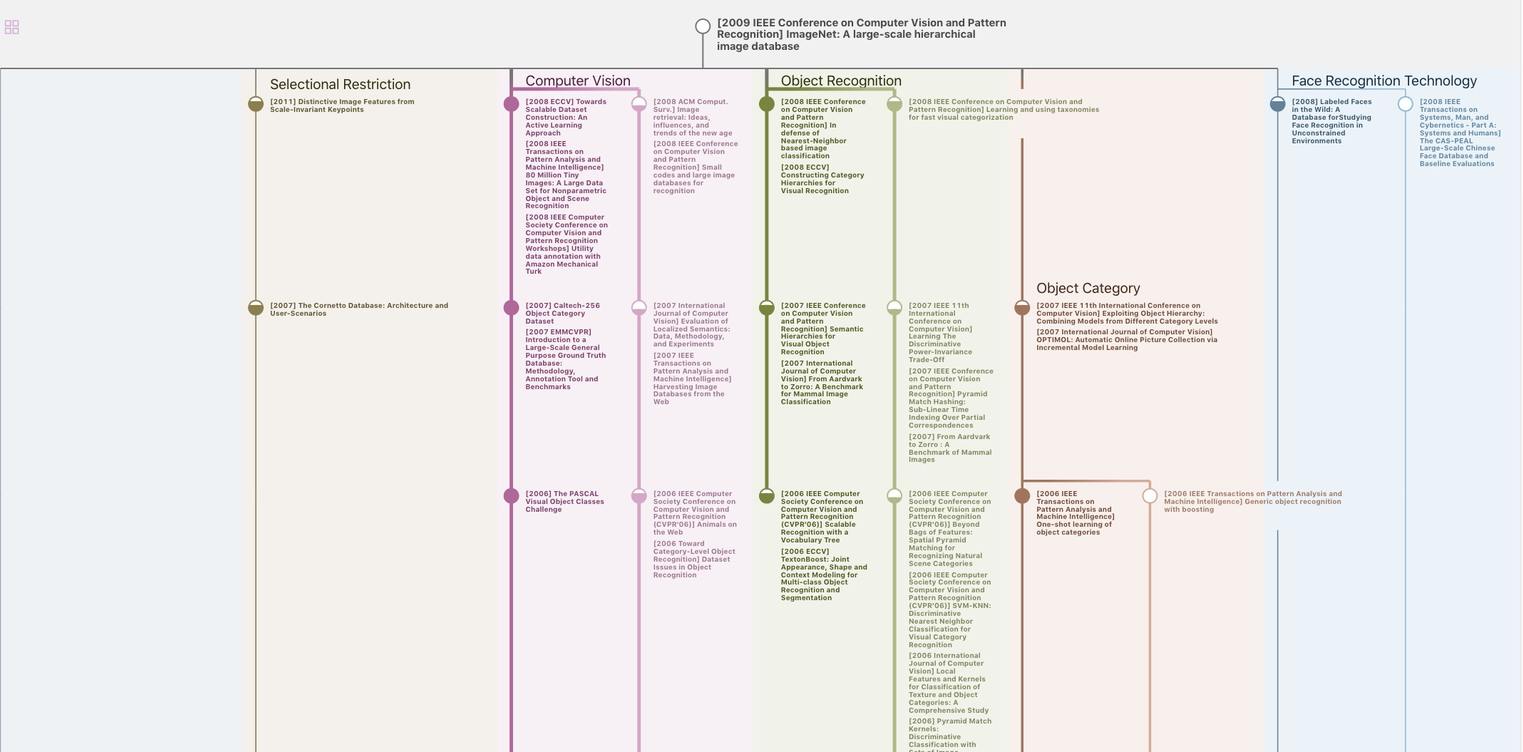
生成溯源树,研究论文发展脉络
Chat Paper
正在生成论文摘要