Directional-Guided Motion Sensitive Descriptor for Automated Detection of Hypertension Using Ultrasound Images
IEEE ACCESS(2024)
摘要
The current work proposes an efficient assessment of hypertension (HTN) using a Directional-Guided Motion Sensitive (DGMS) descriptor and Machine Learning (ML) algorithm. The main objective of the proposed work is to automate the detection of HTN using ultrasound (US) images. The four-chamber US images from 70 healthy subjects and 70 HTN patients are collected. A novel pipelined architecture has been developed in two stages with four phases: preprocessing, feature extraction using DGMS descriptor, feature ranking and selection, and classification using shallow K-Nearest Neighbor classifier. The proposed model has achieved a classification accuracy of 98% using a set of prominent features, predominating the performance attained by other approaches. This study suggests US contains predictive signals even when standard measures are normal and lays the groundwork for artificial intelligence-assisted cardiac assessment to aid quicker, more objective diagnosis and earlier treatment. If further validated on additional diverse patient data, the technology could be integrated into clinics to enhance HTN detection through automated, early discernment of subtle manifestations missed by human eyes and traditional metrics.
更多查看译文
关键词
Computer aided diagnostic tool,directional-guided descriptor,hypertension,shallow classifier,ultrasound images
AI 理解论文
溯源树
样例
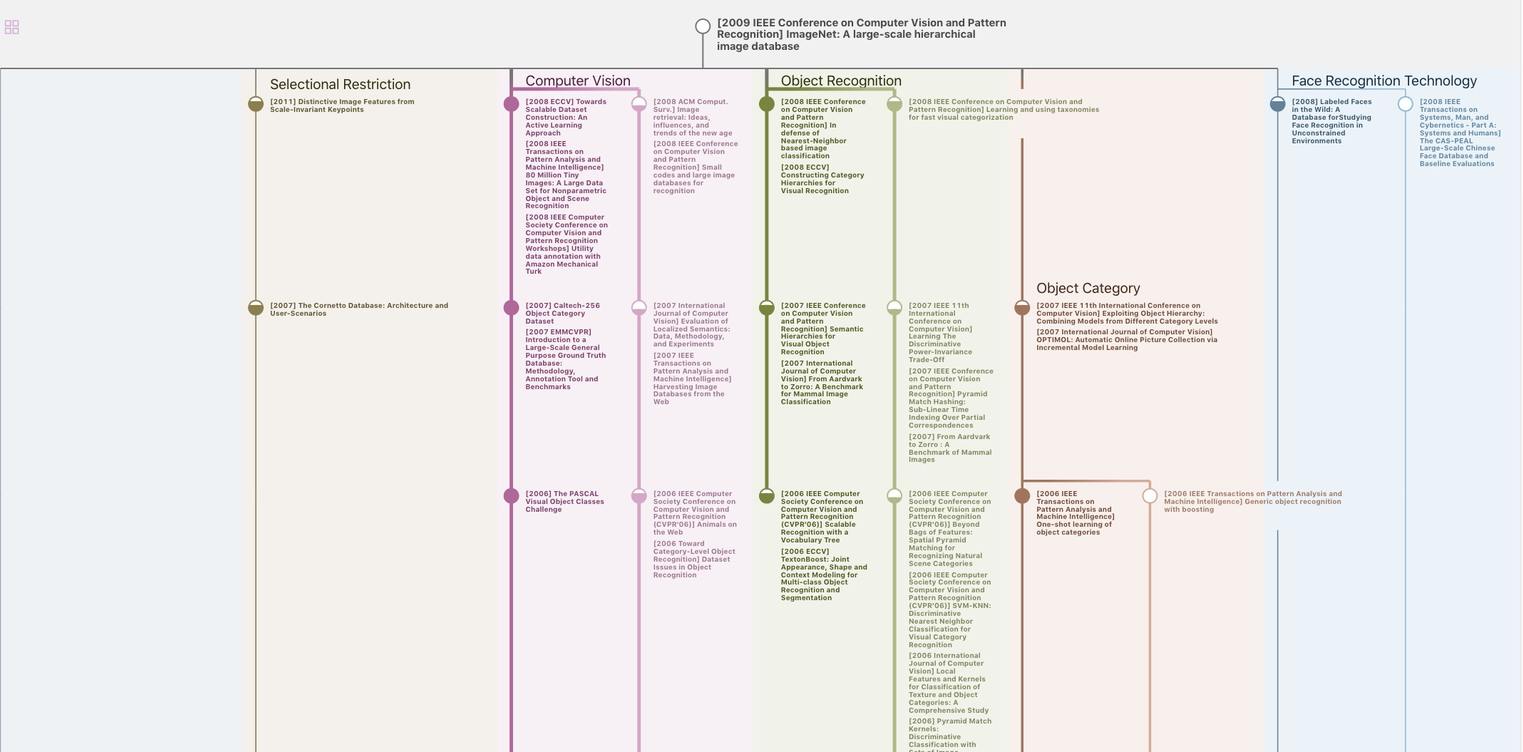
生成溯源树,研究论文发展脉络
Chat Paper
正在生成论文摘要