CLUE: Contrastive language-guided learning for referring video object segmentation
PATTERN RECOGNITION LETTERS(2024)
摘要
Referring video object segmentation (R-VOS), the task of separating the object described by a natural language query from the video frames, has become increasingly critical with recent advances in multi-modal understanding. Existing approaches are mainly visual-dominant in both representation-learning and decision-making process, and are less sensitive to fine-grained clues in text description. To address this, we propose a language-guided contrastive learning and data augmentation framework to enhance the model sensitivity to the fine-grained textual clues (i.e., color, location, subject) in the text that relate heavily to the video information. By substituting key information of the original sentences and paraphrasing them with a text-based generation model, our approach conducts contrastive learning through automatically building diverse and fluent contrastive samples. We further enhance the multi-modal alignment with a sparse attention mechanism, which can find the most relevant video information by optimal transport. Experiments on a large-scale R-VOS benchmark show that our method significantly improves strong Transformer-based baselines, and further analysis demonstrates the better ability of our model in identifying textual semantics.
更多查看译文
关键词
Video object segmentation,Multi-modal,Contrastive learning,Deep learning
AI 理解论文
溯源树
样例
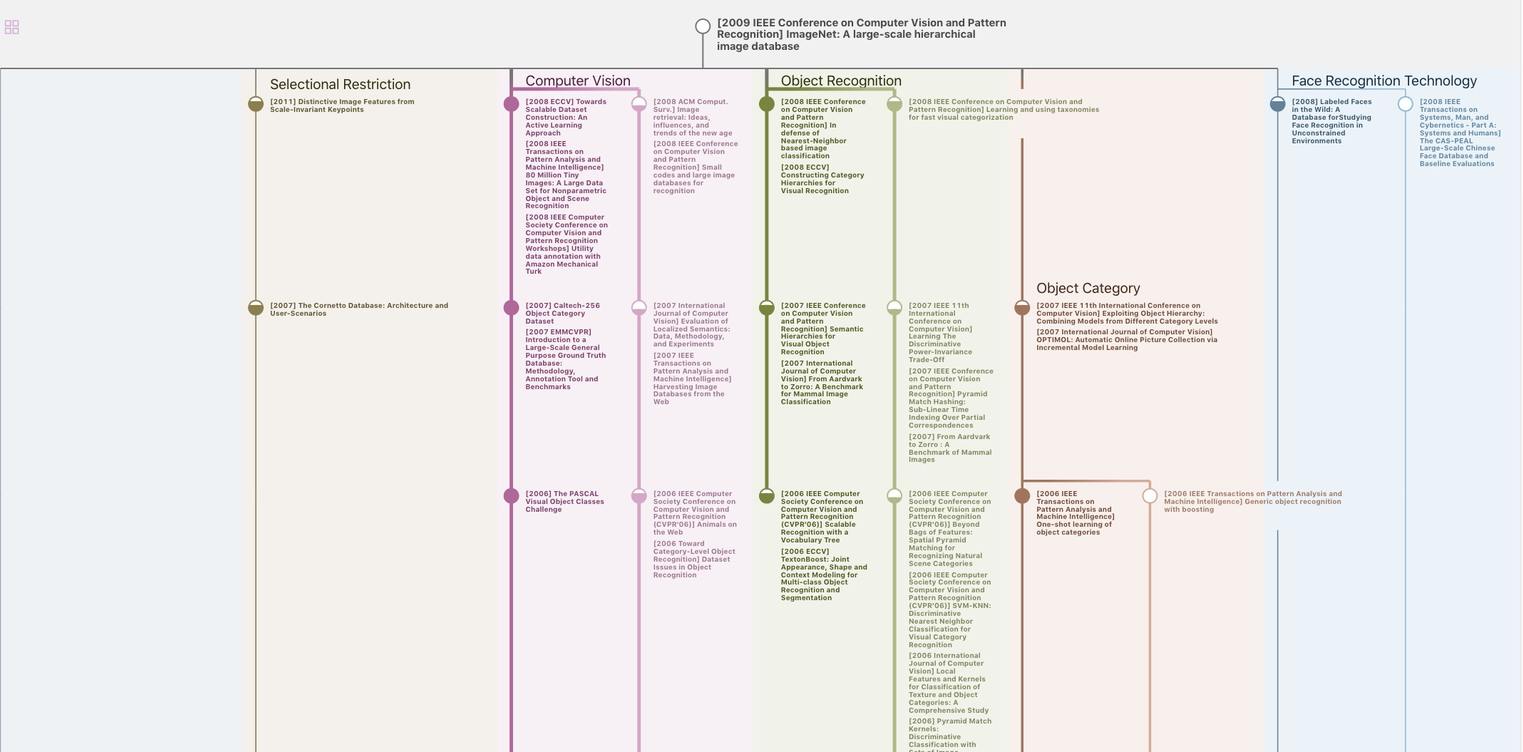
生成溯源树,研究论文发展脉络
Chat Paper
正在生成论文摘要