An ensemble neural network model for predicting the energy utility in individual houses
COMPUTERS & ELECTRICAL ENGINEERING(2024)
摘要
With the rapid increase in the world's human population and the advancement of technology, energy utilization has substantially increased. To sustain a stable stream of energy, it is vital to anticipate power utilization beforehand. This issue makes predicting short-term electrical time series use difficult. In this research, the user proposed a neural network with a deep learning prototype that performs energy prediction by combining. Long Short-Term Memory (LSTM) and Feed Forward Neural Networks (FFNN). In order to enhance the performance, the ensemble FFNN-LSTM network and the Stationary Wavelet Transform (SWT) method are combined to create a hybrid deep learning algorithm. The SWT's reduction of instability and expansion of data dimensionality may improve the FFNN-LSTM forecasting accuracy. The suggested technique's predicting effectiveness is even further improved by the ensemble Long short-term neural network. On the basis of a real-world household energy usage database gathered by the "UKDALE" project, validation studies were carried out. Researchers determined that the LSTM-FFNN based SWT produced a better result in terms of accuracy and energy utilization than other existing results after contrasting the experimental findings to the baseline, which is the conventional LSTM and Moving Average (MA) based upon the Root Mean Squared Error (RMSE) score.
更多查看译文
关键词
LSTM-FFNN,Stationary wavelet transform,UK-DALE,Energy utilization
AI 理解论文
溯源树
样例
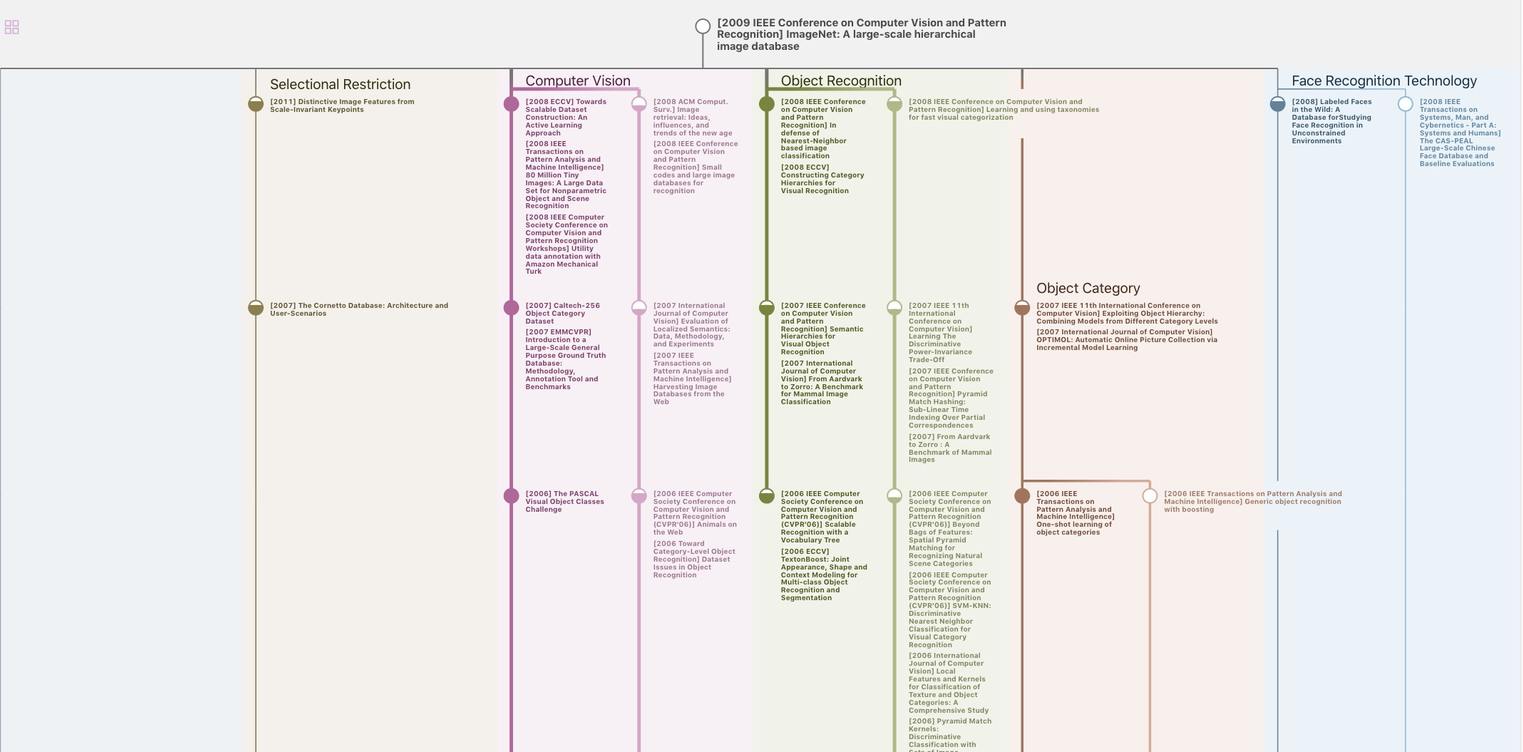
生成溯源树,研究论文发展脉络
Chat Paper
正在生成论文摘要