End-to-end comparative opinion quintuple extraction as bipartite set prediction with dynamic structure pruning
EXPERT SYSTEMS WITH APPLICATIONS(2024)
摘要
Comparative Opinion Quintuple Extraction (COQE) is an emerging task focused on identifying comparative relationships within sentence-level comments. COQE involves extracting a quintuple that encapsulates a specific comparative relation, encompassing a subject, object, comparative aspect, opinion, and preference. Previous approaches to COQE relied on pipeline methods, which unfortunately suffered from error propagation during the sequential process. In our work, we present an efficient and highly effective end-to-end solution for COQE, improving three key aspects: encoding, decoding, and learning. First, in terms of feature encoding, we introduce external syntactic dependency structural features to enhance the modeling of opinion elements. We also propose a novel dynamic structural pruning (DSP) mechanism to refine and optimize the raw syntax structure, ensuring alignment with the end task. Second, regarding decoding, we address the challenge of non-coherent relationships among quintuple constituents by formulating COQE as a set prediction problem. We employ a non-autoregressive decoding scheme to simultaneously generate all possible quintuples in parallel, increasing decoding speed while retaining the advantages of a generative approach. Finally, from a learning perspective, we introduce three advanced training targets: bipartite matching learning loss, cross-element contrastive loss, and mention boundary learning loss. These targets promote interaction learning between quadruples and elements. Through extensive experiments on three benchmark datasets, our end-to-end COQE system demonstrates significant performance improvements over both current pipeline baselines and state-of-the-art joint systems by 2 to 3 points. We also demonstrate that our proposed dynamic pruning strategy over syntactic dependency features is important to the overall performance, and meanwhile our system is capable of producing explainable predictions. Furthermore, the non-autoregressive decoding greatly enhances the inference efficiency, and multiple advanced training losses help achieve better learning process.
更多查看译文
关键词
Natural language processing,Opinion mining,Sentiment analysis,Information extraction,Syntactic dependency,Non-autoregressive generation
AI 理解论文
溯源树
样例
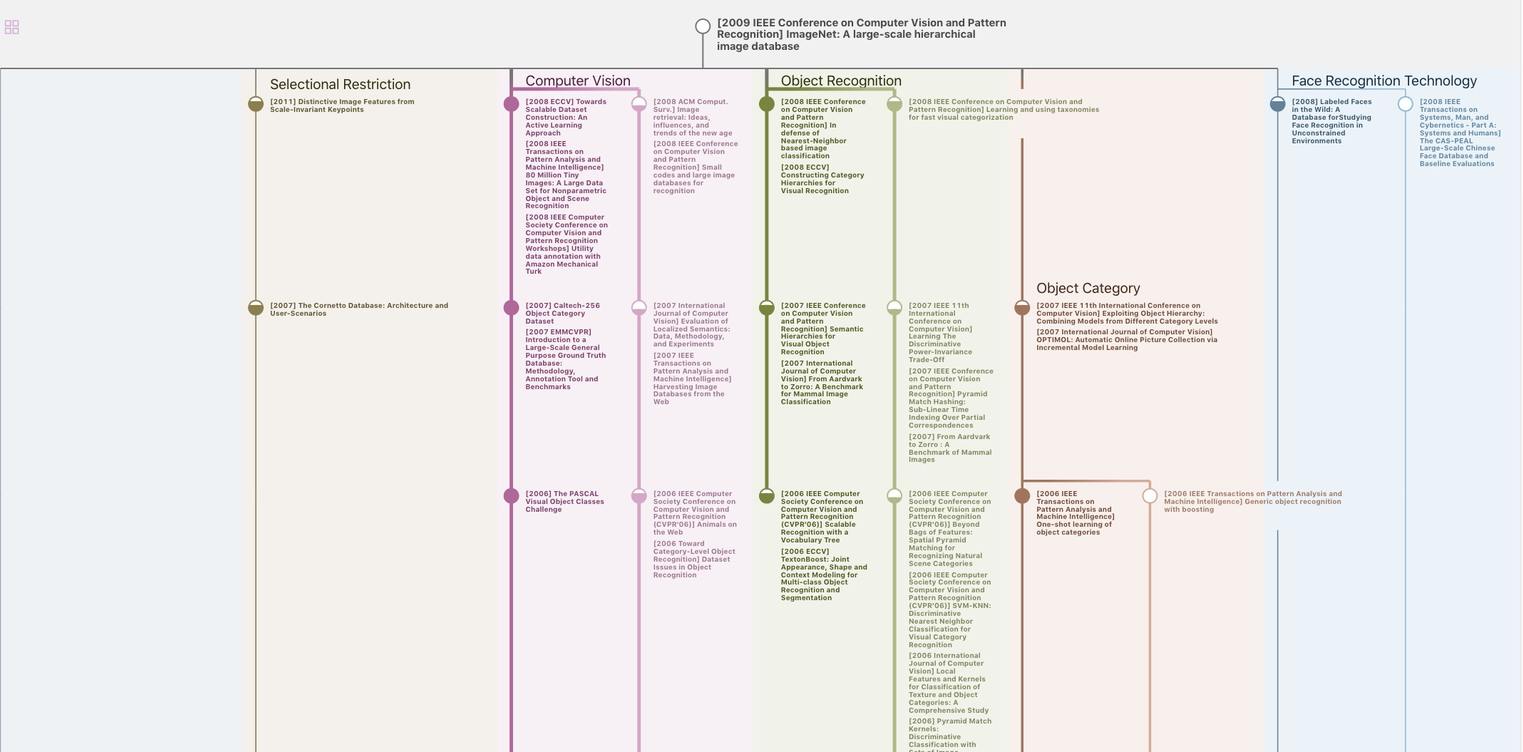
生成溯源树,研究论文发展脉络
Chat Paper
正在生成论文摘要