Universum driven cost-sensitive learning method with asymmetric loss function
ENGINEERING APPLICATIONS OF ARTIFICIAL INTELLIGENCE(2024)
摘要
Class imbalance learning (CIL) has been a critical yet challenging topic for a long time. To address the issue, this paper proposes a cost-sensitive learning method based on the linear-exponential (LINEX) loss function and the Universum (UCSSVM). On one hand, the asymmetry of LINEX loss function is leveraged to realize costsensitive learning, by treating each instance discriminately. On the other hand, to enhance the classification performance, a novel and effective strategy is put forward to generate Universum with the assistance of hardto-classify samples (small margin distance). The mini-batch gradient descent (MBGD) approach is adopted to optimize UCSSVM. Meanwhile, extensive experiments demonstrate its superiority in CIL and the necessity of using Universum.
更多查看译文
关键词
Class imbalance learning,Cost-sensitive learning,LINEX loss function,Universum generation,Margin distance,Support vector machine
AI 理解论文
溯源树
样例
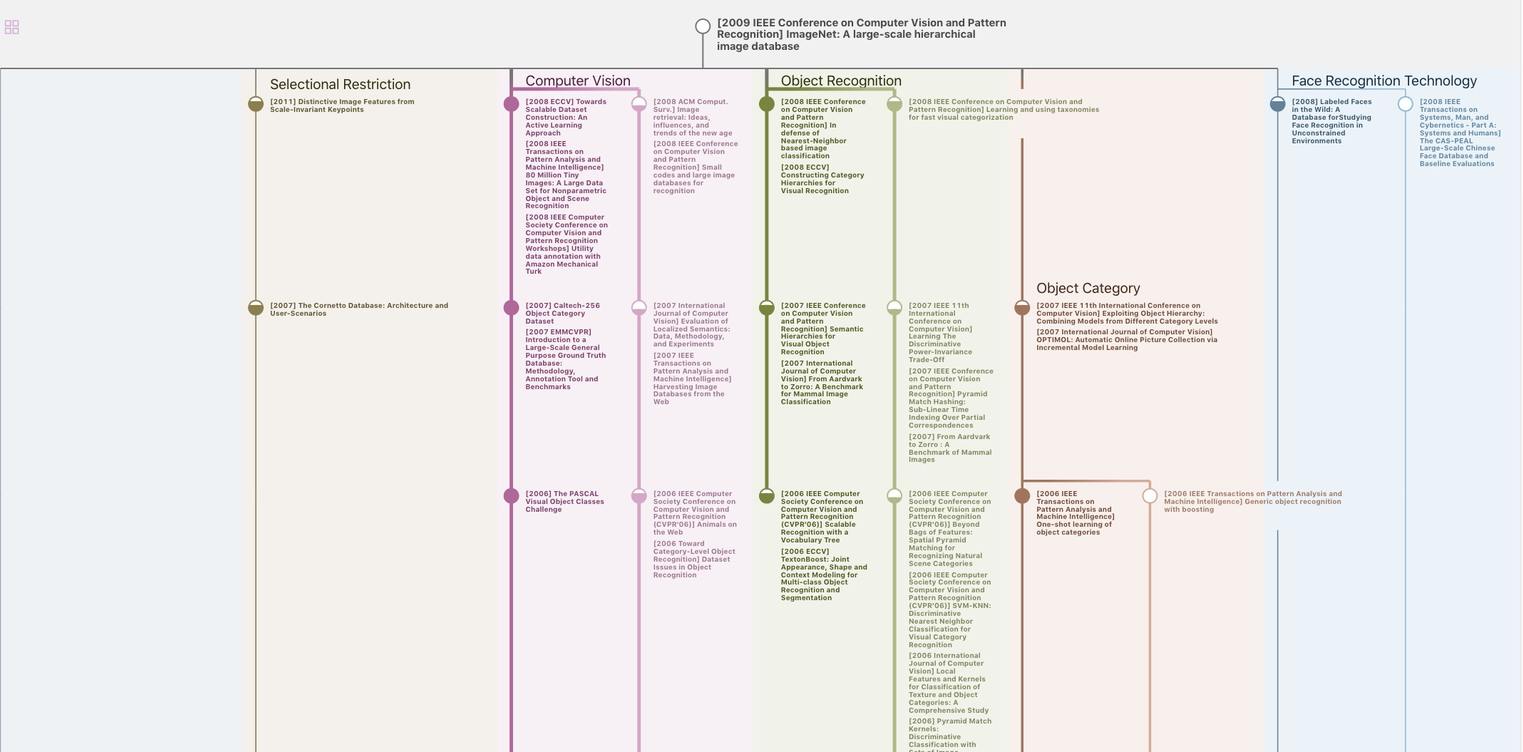
生成溯源树,研究论文发展脉络
Chat Paper
正在生成论文摘要