OBiLSTM-Based Intrusion Detection Method for Industrial Internet of Things.
2023 8th International Conference on Data Science in Cyberspace (DSC)(2023)
摘要
Industrial Internet of Things (IIoT), is the integration of the latest scientific technology and traditional industrial networks developed on the General Electric platform. Although plenty of advantages, IIoT also suffers from many serious security challenges. As a result, IIoT would be frequently attacked by malicious adversaries. Therefore, it is necessary to design some mechanisms (such as intrusion detection) to protect the security of IIoT. Recently, a lot of intrusion detection schemes have been proposed. However, due to the large scale and temporality of network traffic data in IIoT, the detection accuracy of the existing intrusion detection schemes is not attractive. In order to improve the detection accuracy, we propose a new intrusion detection method for IIoT based on Optimized Bi-Directional Long and Short-term Memory (OBiLSTM). We firstly improve BiLSTM by replacing the reverse long and short-term memory network (LSTM) with gated recurrent unit (GRU), then design a new deep neural network named OBiLSTM. Subsequently, we utilize OBiLSTM to design a new intrusion detection method, which can greatly improve detection accuracy in IIoT environment. Finally, implement the proposed intrusion detection method and test the performance based on UNSW-NB15 dataset. The experimental results demonstrate that the accuracy, recall, and F1_score of our proposed method are much more attractive than the previous methods.
更多查看译文
关键词
Industrial Internet of Things,Intrusion Detection,Neural Network
AI 理解论文
溯源树
样例
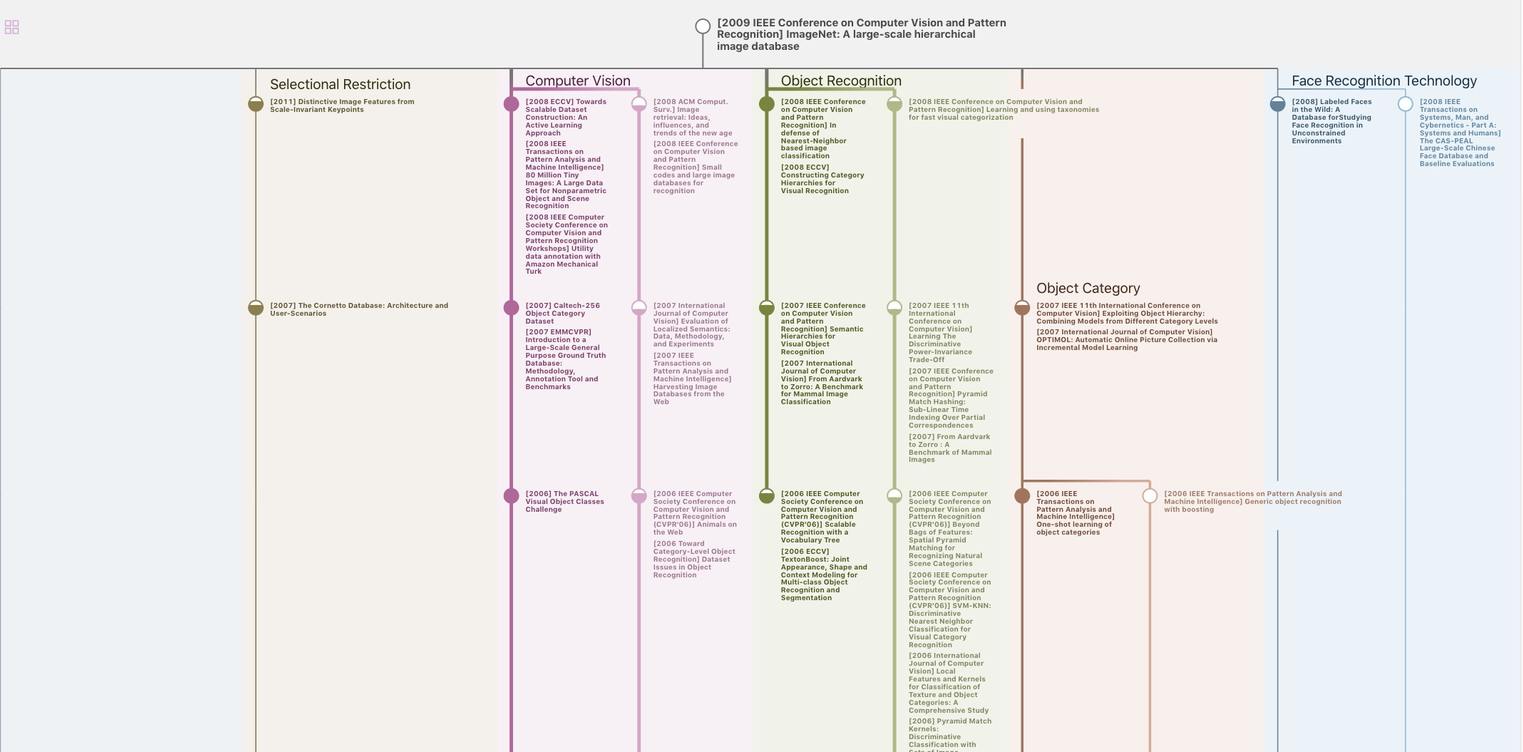
生成溯源树,研究论文发展脉络
Chat Paper
正在生成论文摘要