Knowledge Graph Embedding via Adaptive Negative Subsampling.
2023 8th International Conference on Data Science in Cyberspace (DSC)(2023)
摘要
Knowledge graph embedding is an important approach for addressing the task of knowledge graph completion, which has received extensive attention and research in recent years. However, some existing methods, such as non-Euclidean space embedding models, suffer from high computational complexity and time costs, as well as high embedding dimensions, without significant improvements over classical models. In this study, we apply adaptive negative sampling to the classical knowledge graph embedding model transE. We have performed optimization on the loss function while preserving the fundamental principles of embedding. As a result, our model is referred to as ANStransE. We also experimentally investigate the impact of different dimensions and evaluate our approach on three commonly used datasets (WN18RR, FB15k-237, and YAGO3-10). The experimental results demonstrate a significant performance improvement achieved by our method, enabling a simple model to achieve results close to the state-of-the-art. Through adaptive negative sampling, we are able to effectively utilize negative samples for model training, thereby enhancing the accuracy and efficiency of knowledge graph representation learning.
1
1
The relevant code has been made publicly available at https://github.com/xiaoluoElon/ANStransE.
更多查看译文
关键词
KG Embedding,Negative Sample,TransE
AI 理解论文
溯源树
样例
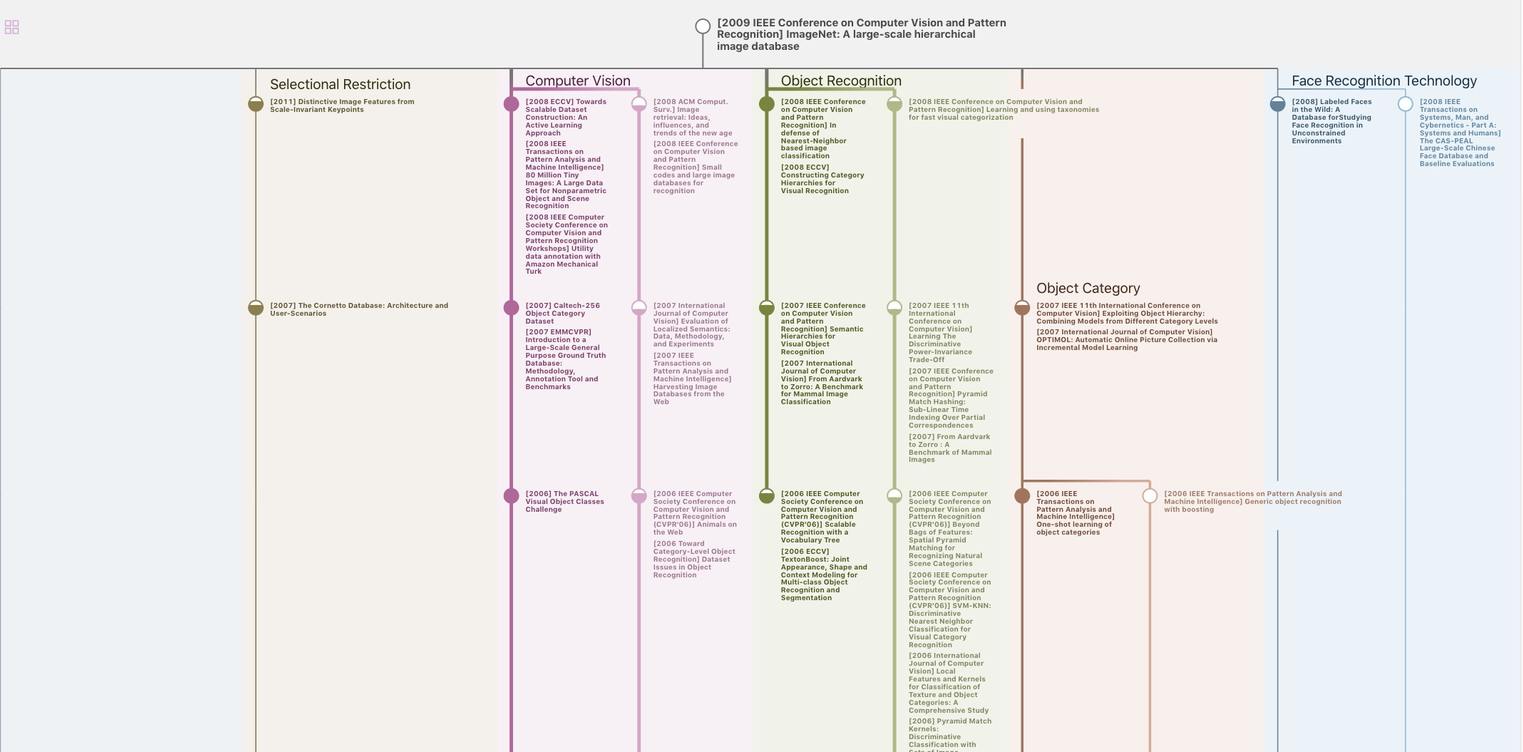
生成溯源树,研究论文发展脉络
Chat Paper
正在生成论文摘要