A Data-Distillation-Enhanced Autoencoder for Detecting Anomalous Gas Consumption
IEEE INTERNET OF THINGS JOURNAL(2024)
摘要
The number of natural gas users has been growing rapidly in China due to the promotion of clean energy and the economic benefits of natural gas, especially in businesses and industries. Though the infrastructures for gas supplies have been highly improved, gas providers are still suffering from various problems, such as malfunctioning gas meters, gas leakage, gas theft, etc. With the development of the Internet of Things, smart gas meters have been widely adopted by gas providers to collect real-time gas consumption data for billing purposes which can also serve as a basis for anomaly detection. One challenge of using such data for anomaly detection is that it is difficult to obtain sufficient labeled data for model training. To address this challenge, we propose D(2)AE, a data-distillation-enhanced autoencoder for detecting anomalous gas consumption, which consists of three modules. The first module preprocesses the raw meter readings and carries out a rule-based anomaly detection. The second module extracts the normal gas usage patterns via an integration of correlation and clustering-based consistency evaluation methods. The extracted normal usage patterns are then used in the third module to train an autoencoder for anomaly detection. D(2)AE intends to provide a method to detect anomalous gas consumption induced by various causes such that manual inspection can be largely reduced. Moreover, D(2)AE does not require user-specific information and can be applied to different types of gas users. Based on a real-world gas consumption data set, we carry out a set of experiments and show that D(2)AE outperforms the existing anomaly detection methods in the literature and improves the F-1 score by an average of 7.4% for restaurant users and 5.7% for canteen users.
更多查看译文
关键词
Anomaly detection,gas consumption,time series,unsupervised learning,urban computing
AI 理解论文
溯源树
样例
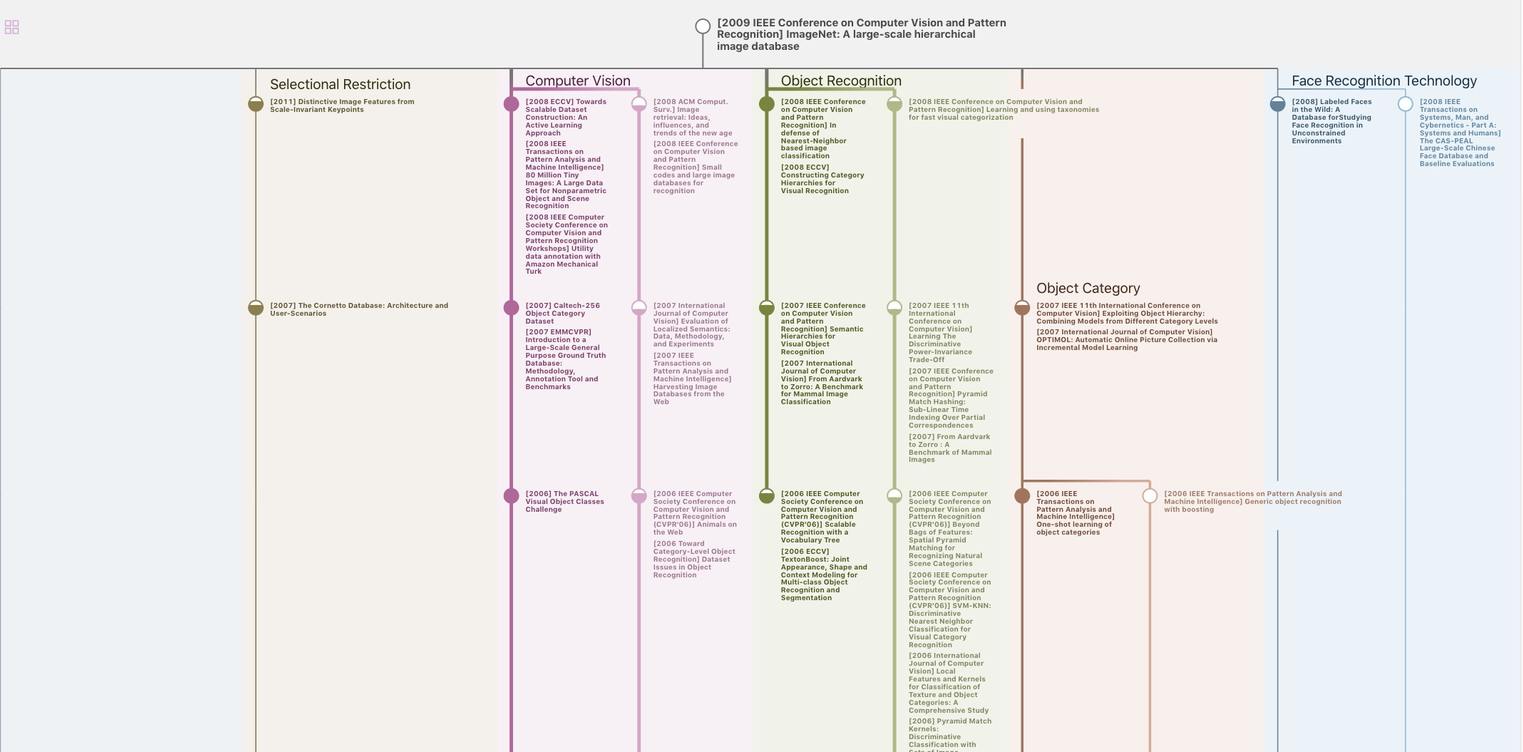
生成溯源树,研究论文发展脉络
Chat Paper
正在生成论文摘要