From Signals to Insights: Uncovering Latent Degradation with Deep Learning as a Stepping Stone Towards Digital Twins of Failures (DTFs).
2023 7th International Conference on System Reliability and Safety (ICSRS)(2023)
摘要
In the study, we investigate the application of deep learning using discretised hydraulic power signals for delineating healthy from non-healthy states on a hydraulic system of a rubber mixing machine. Using the concept of functional-productiveness, the study uses class labels determined as semi-supervised learning by relying on discretised signal behaviour and manually inputted target labels. The feature extraction process is performed through extensive data wrangling to extract discrete-domain features for training the neural networks. The study compares five deep neural network models, in which case the most suitable model is determined by the area under the receiver operator curve (AUC) and the time to train the network. The neural network architecture optimises via Genetic Algorithm (GA). The final result shows that GLobally convergent Resilient PROPagation with Smallest Absolute Gradient (GLPROP-SAG) outperforms other models regarding AUC and time to train the network.
更多查看译文
关键词
industry 5.0,digital twins of failures,deep neural network,deep learning,energy-based maintenance,sustainable maintenance
AI 理解论文
溯源树
样例
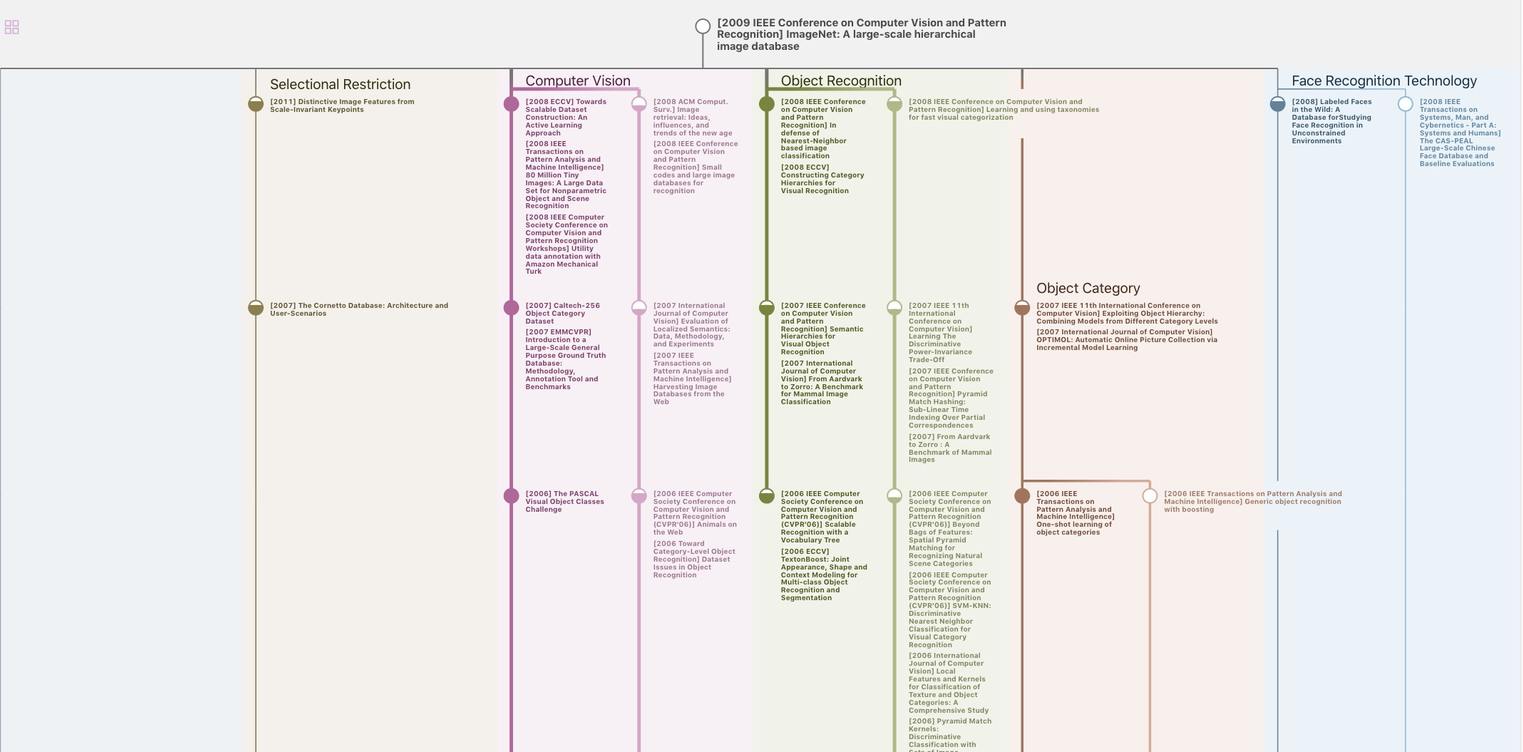
生成溯源树,研究论文发展脉络
Chat Paper
正在生成论文摘要