Dual Sparse Structured Subspaces and Graph Regularisation for Particle Swarm Optimisation-Based Multi-Label Feature Selection
IEEE COMPUTATIONAL INTELLIGENCE MAGAZINE(2024)
摘要
Many real-world classification problems are becoming multi-label in nature, i.e., multiple class labels are assigned to an instance simultaneously. Multi-label classification is a challenging problem due to the involvement of three forms of interactions, i.e., feature-to-feature, feature-to-label, and label-to-label interactions. What further complicates the problem is that not all features are useful, and some can deteriorate the classification performance. Sparsity-based methods have been widely used to address multi-label feature selection due to their efficiency and effectiveness. However, most (if not all) existing methods do not consider the three forms of interactions simultaneously, which could hinder their ability to achieve good performance. Moreover, most existing methods are gradient-based, which are prone to getting stuck at local optima. This paper proposes a new sparsity-based feature selection approach that can simultaneously consider all three forms of interactions. Furthermore, this paper develops a novel sparse learning method based on particle swarm optimisation that can avoid local optima. The proposed method is compared against the state-of-the-art multi-label feature selection methods in terms of multi-label classification performance. The results show that our method performed significantly better in selecting high-quality feature subsets with respect to various feature subset sizes.
更多查看译文
关键词
Learning systems,Feature extraction,Particle swarm optimization,Optimization,Labeling
AI 理解论文
溯源树
样例
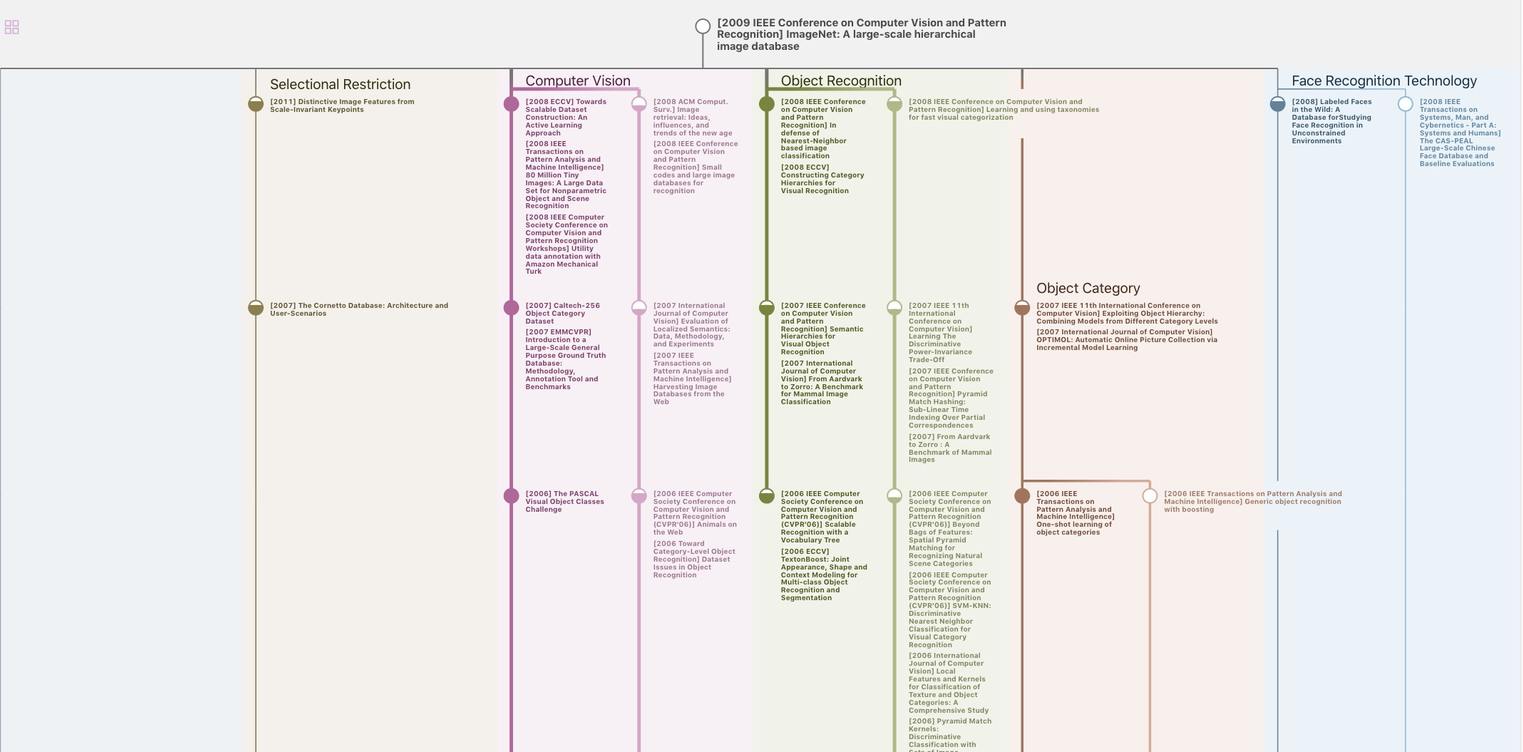
生成溯源树,研究论文发展脉络
Chat Paper
正在生成论文摘要