Reinforcement Learning for Optimizing Delay-Sensitive Task Offloading in Vehicular Edge-Cloud Computing
IEEE INTERNET OF THINGS JOURNAL(2024)
摘要
With the appearance of more and more devices connected to the Internet, the world has witnessed an ever-growing number of data to be processed. Among those, many tasks require swift execution time, while the storage and computation capability of Internet of Things (IoT) devices are limited. To address the demands of delay-sensitive tasks, we present a vehicular edge-cloud computing (VECC) network that leverages powerful computation capabilities through the deployment of servers in proximity to task-generated devices, as well as the utilization of idle resources from smart vehicles to share the workload. Because these limited resources are vulnerable to sudden data arising, it is imperative to incorporate cloud servers to prevent system overload. The challenge now is to find a task offloading strategy that collaborates both edges and cloud resources to minimize the total time surpassing the quality baseline of each task (tolerance time) and make all tasks meet their soft deadlines of quality. To reach this goal, we first model the task offloading problem in VECC as a Markov decision process (MDP). Then, we propose advantage-oriented task offloading with a dueling actor-insulator network scheme to solve the problem. This value-based reinforcement learning (RL) method helps the agent find an effective policy when not knowing all the state attributes changes. The effectiveness of our method is demonstrated by performance evaluations based on real-world bus traces in Rio de Janeiro (Brazil). The experimental results show that our proposal reduces the tolerance time by at least 8.81% compared to other RL algorithms and 75% compared to greedy approaches.
更多查看译文
关键词
Cloud computing,deep Q-learning,delay-sensitive task,task offloading,vehicular edge computing (VEC)
AI 理解论文
溯源树
样例
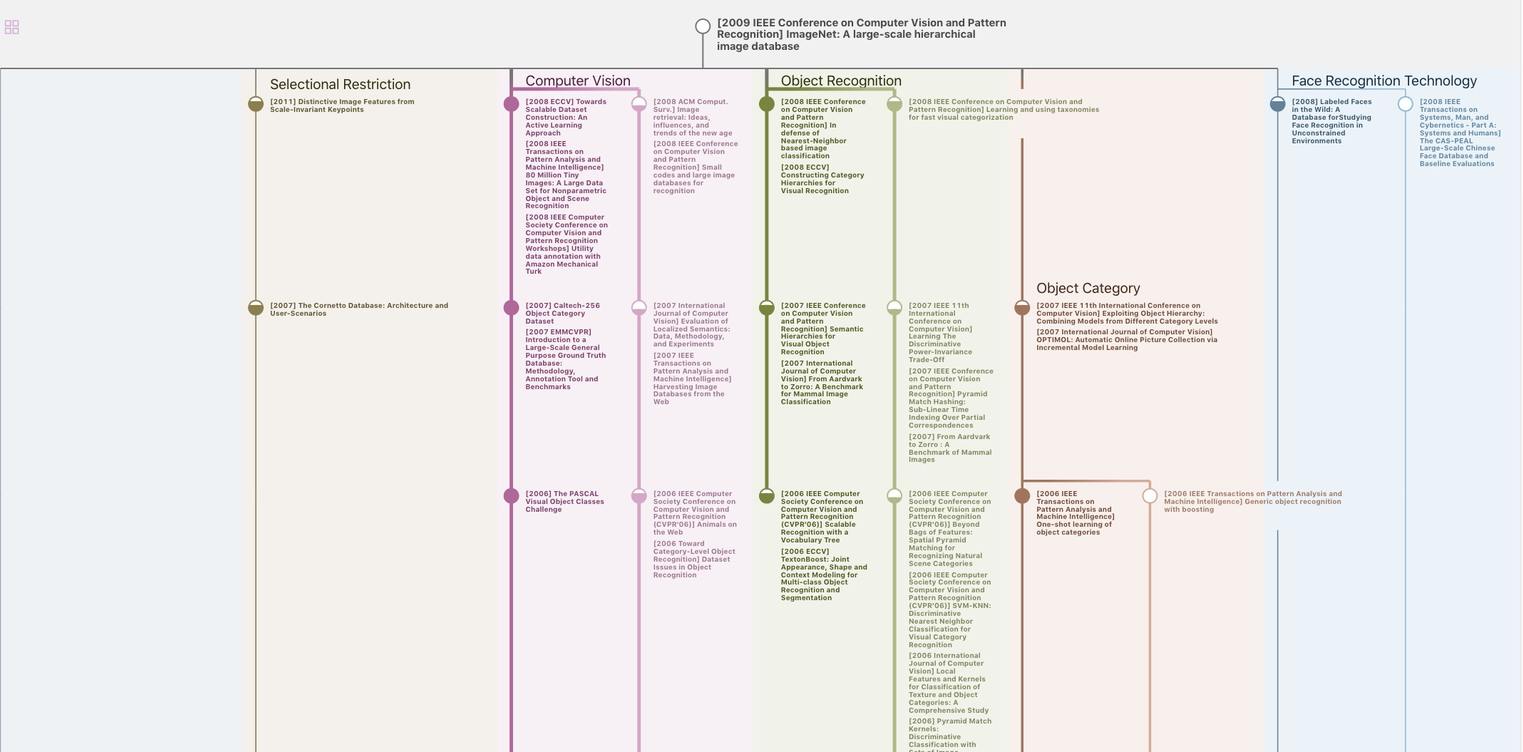
生成溯源树,研究论文发展脉络
Chat Paper
正在生成论文摘要