Sum Rate Maximization in Multi-Cell Multi-User Networks: An Inverse Reinforcement Learning-Based Approach
IEEE WIRELESS COMMUNICATIONS LETTERS(2024)
摘要
Currently, reinforcement learning (RL) is widely used in wireless network optimization, where the key factor is to design the efficient reward functions manually. However, the limitations of the man-made reward function are mainly that it is subject to human subjective factors and requires extensive simulations to select appropriate parameters. To cope with this, the letter proposes an inverse reinforcement learning (IRL)-based approach to optimize the transmit power control in multi-cell multi-user networks, which does not require any prior knowledge about the system and the wireless environment. An optimization problem is formulated to maximize the achievable sum information rate subject to the minimal required information rate of users. To tackle the NP-hard non-convex problem, our IRL-based approach is able to obtain rewards automatically rather than through a man-made reward function. In particular, the successive convex approximation (SCA)-based approach is adopted as the expert-policy to generate the expert data to train the automatic rewards. Simulation results support that the proposed IRL-based approach outperforms the traditional RL-based ones.
更多查看译文
关键词
Inverse reinforcement learning,power allocation,QoS constraints,cellular network
AI 理解论文
溯源树
样例
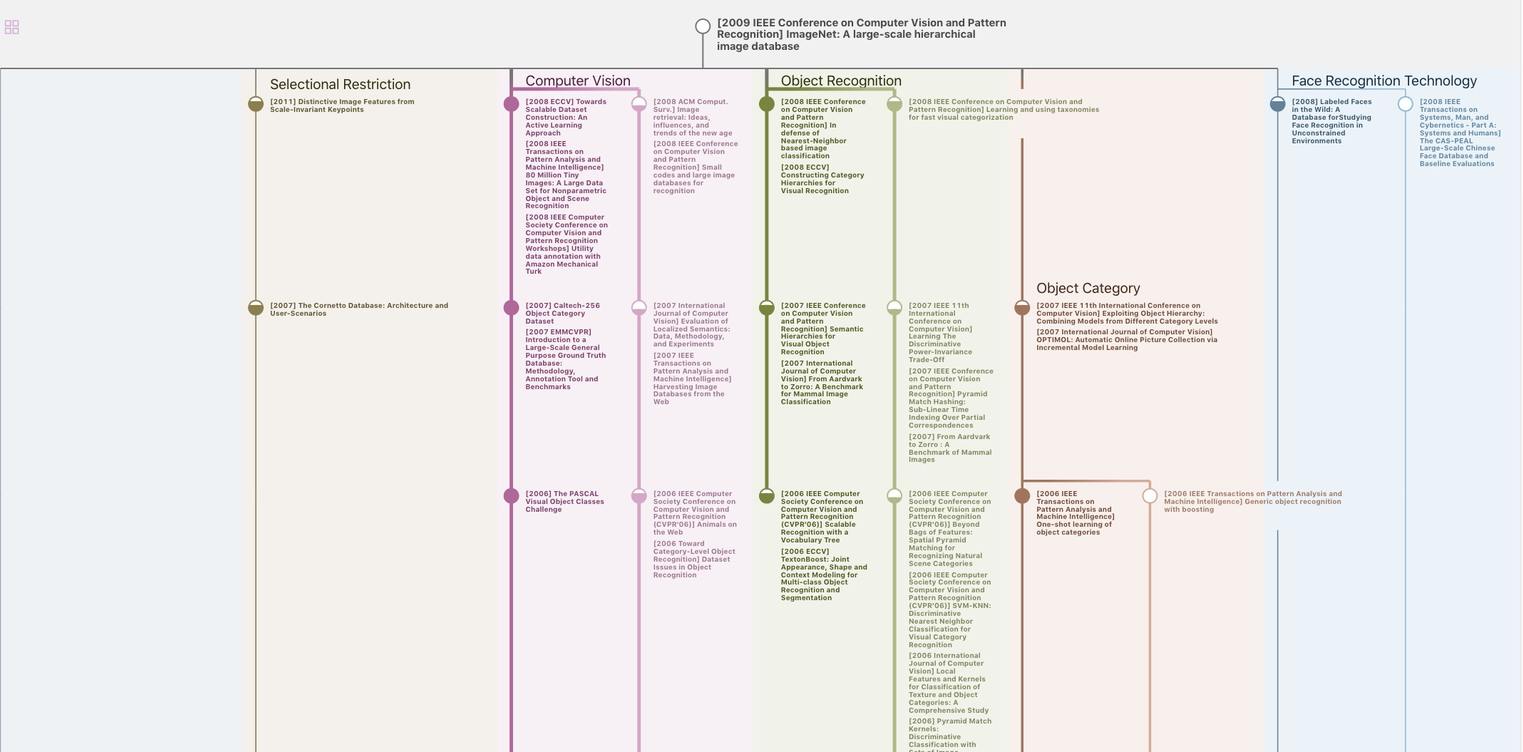
生成溯源树,研究论文发展脉络
Chat Paper
正在生成论文摘要