Process parameter effects estimation and surface quality prediction for selective laser melting empowered by Bayes optimized soft attention mechanism-enhanced transfer learning
COMPUTERS IN INDUSTRY(2024)
摘要
Additive Manufacturing (AM), particularly Selective Laser Melting (SLM), has revolutionized the industrial manufacturing sector owing to its remarkable design flexibility and precision. However, it is well known that slight changes in SLM process parameters may highly affect the surface quality of the as-built product. In this paper, we investigate the influence of SLM printing parameters (laser power, laser scanning speed, layer thickness, and hatch distance) on surface quality and develop a predictive model for surface quality based on the given printing parameters. The developed model is constructed by a Bayesian Optimization and soft Attention mechanism-enhanced Transfer learning (BOAT) framework with superior domain adaptability and generalization capability. Through experimental validation, the effectiveness of the BOAT approach in estimating printing parameters and correlating them with surface quality has been verified. The comprehensive methodology, experimental configurations, prediction results, and ensuing discussions are all presented. This study contributes to providing valuable insights and practical implications for improving the competitiveness and impact of SLM in advanced manufacturing by accurately predicting surface quality with specified printing parameters.
更多查看译文
关键词
additive manufacturing,selective laser melting,bayesian optimization,transfer learning,attention mechanism
AI 理解论文
溯源树
样例
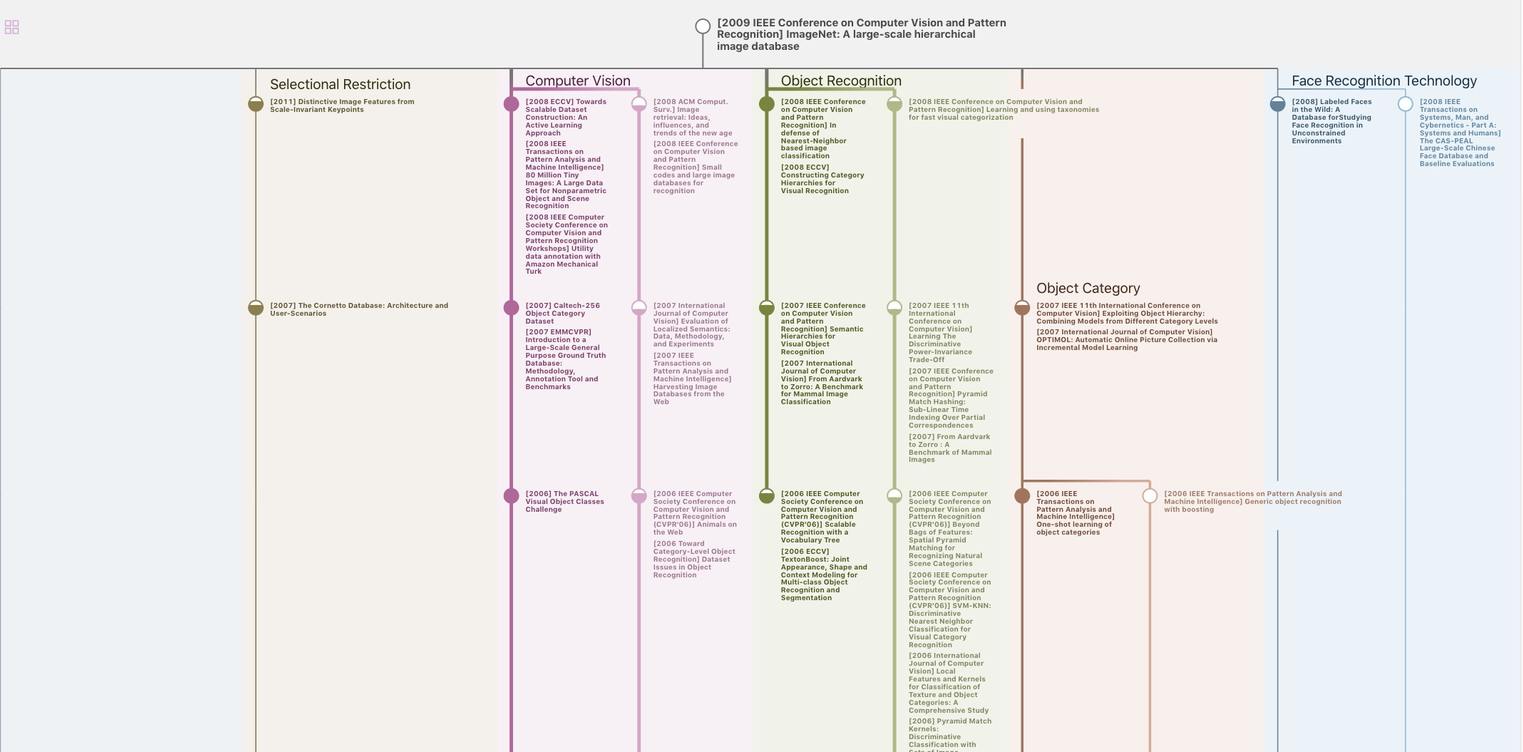
生成溯源树,研究论文发展脉络
Chat Paper
正在生成论文摘要