eSeiz 2.0: An Optimized Pulse Exclusion Mechanism for Accurate and Energy-Efficient Seizure Detection in the IoMT
SN Computer Science(2024)
摘要
Approximately, 50 million people worldwide are impacted by epilepsy, necessitating the development of a seizure detection system that is low power, low latency, and capable of providing accurate and real-time monitoring to address the issue. In this paper, a low-power wearable device for epilepsy has been presented that uses a novel pulse exclusion (PEM) algorithm to characterize seizure and normal activities. PEM resolves the issue of heavy computational burden by reducing the number of channels or features. The feature vectors of reduced size become input to an optimized deep neural network (DNN) classifier for seizure identification. The proposed PEM-based approach has been extensively validated with 10 epileptic subjects obtained from the CHB-MIT Scalp datasets. PEM in combination with DNN classifier shows huge potential in eliminating false detections, and the average specificity of the specified subjects is recorded as 100%, which may be useful for seizure detection and subsequent epilepsy treatment.
更多查看译文
关键词
Electroencephalography (EEG),Pulse exclusion mechanism (PEM),Internet-of-medical-things (IoMT),Deep neural network,Energy efficiency,Feature vector
AI 理解论文
溯源树
样例
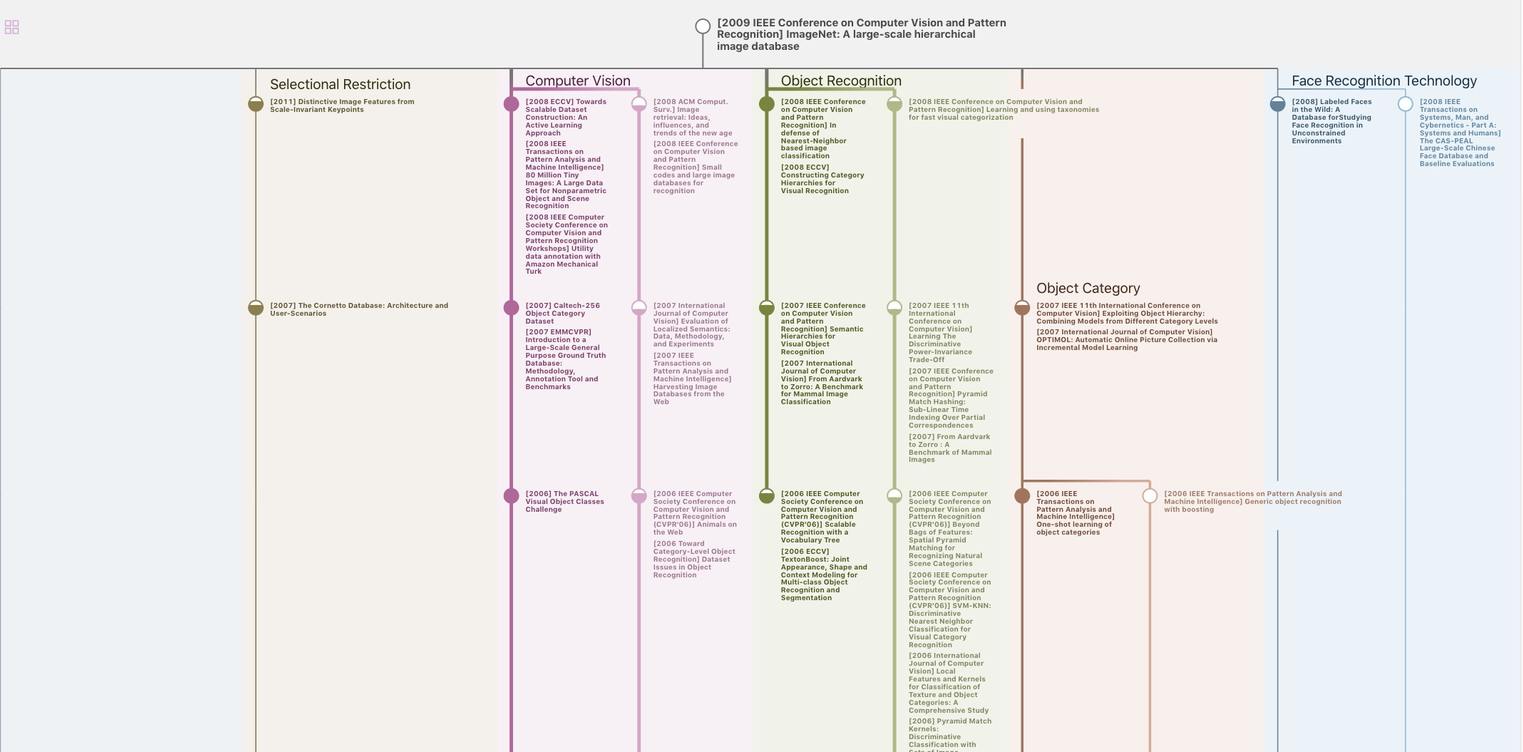
生成溯源树,研究论文发展脉络
Chat Paper
正在生成论文摘要