A fuzzy rough set-based undersampling approach for imbalanced data
International Journal of Machine Learning and Cybernetics(2024)
摘要
How to effectively handle imbalanced data is one of the hot issues in the fields of machine learning and data mining. Undersampling is a popular technique of dealing with imbalanced data. The aim of undersampling is to select an instance subset from the majority class of an imbalanced dataset and then make the dataset balanced. However, the traditional undersampling approaches may lead to the information loss of majority class instances. Therefore, on the basis of the concept of the importance degree of a fuzzy granule, a measure criterion of selecting representative instances from the majority class is presented in this paper by considering the fuzzy relations between the k -nearest neighbors of a majority class instance and the minority class instances. Then, we put forward an undersampling approach based on fuzzy rough sets (USFRS). With the proposed USFRS, the representativeness of the selected majority class instances can be guaranteed and the information loss due to undersampling can be reduced to the utmost extent. Furthermore, USFRS is compared with the relative undersampling methods, and the difference of the experimental results is analyzed by the statistic test. The experimental results demonstrate that USFRS performs well in classification for imbalanced data.
更多查看译文
关键词
Imbalanced data,Fuzzy rough sets,Undersampling,Instance selection
AI 理解论文
溯源树
样例
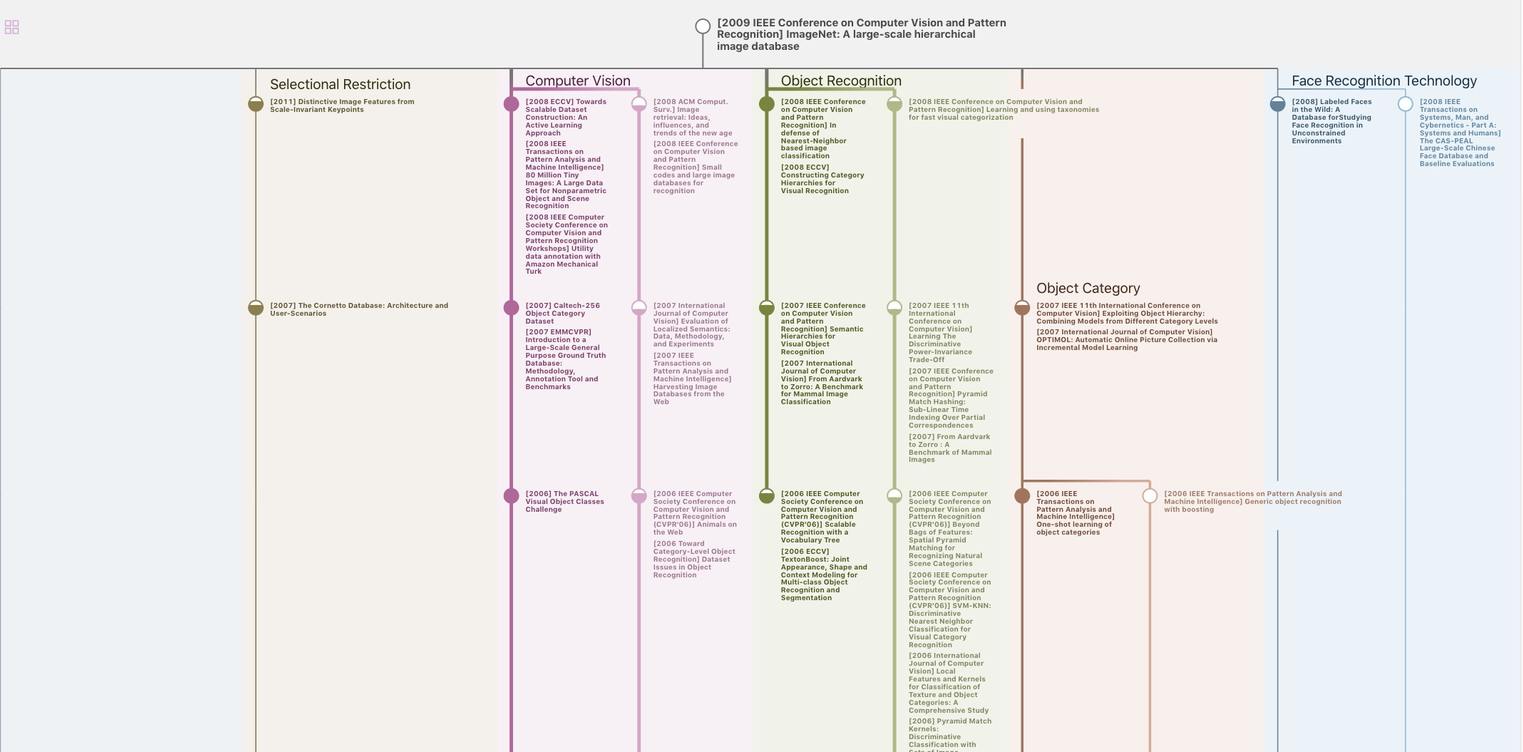
生成溯源树,研究论文发展脉络
Chat Paper
正在生成论文摘要