Deep learning-based immunohistochemical estimation of breast cancer via ultrasound image applications
FRONTIERS IN ONCOLOGY(2024)
摘要
Background Breast cancer is the key global menace to women's health, which ranks first by mortality rate. The rate reduction and early diagnostics of breast cancer are the mainstream of medical research. Immunohistochemical examination is the most important link in the process of breast cancer treatment, and its results directly affect physicians' decision-making on follow-up medical treatment.Purpose This study aims to develop a computer-aided diagnosis (CAD) method based on deep learning to classify breast ultrasound (BUS) images according to immunohistochemical results.Methods A new depth learning framework guided by BUS image data analysis was proposed for the classification of breast cancer nodes in BUS images. The proposed CAD classification network mainly comprised three innovation points. First, a multilevel feature distillation network (MFD-Net) based on CNN, which could extract feature layers of different scales, was designed. Then, the image features extracted at different depths were fused to achieve multilevel feature distillation using depth separable convolution and reverse depth separable convolution to increase convolution depths. Finally, a new attention module containing two independent submodules, the channel attention module (CAM) and the spatial attention module (SAM), was introduced to improve the model classification ability in channel and space.Results A total of 500 axial BUS images were retrieved from 294 patients who underwent BUS examination, and these images were detected and cropped, resulting in breast cancer node BUS image datasets, which were classified according to immunohistochemical findings, and the datasets were randomly subdivided into a training set (70%) and a test set (30%) in the classification process, with the results of the four immune indices output simultaneously from training and testing, in the model comparison experiment. Taking ER immune indicators as an example, the proposed model achieved a precision of 0.8933, a recall of 0.7563, an F1 score of 0.8191, and an accuracy of 0.8386, significantly outperforming the other models. The results of the designed ablation experiment also showed that the proposed multistage characteristic distillation structure and attention module were key in improving the accuracy rate.Conclusion The extensive experiments verify the high efficiency of the proposed method. It is considered the first classification of breast cancer by immunohistochemical results in breast cancer image processing, and it provides an effective aid for postoperative breast cancer treatment, greatly reduces the difficulty of diagnosis for doctors, and improves work efficiency.
更多查看译文
关键词
computer-aided diagnosis,deep learning,neural network,immunohistochemistry,node of breast cancer
AI 理解论文
溯源树
样例
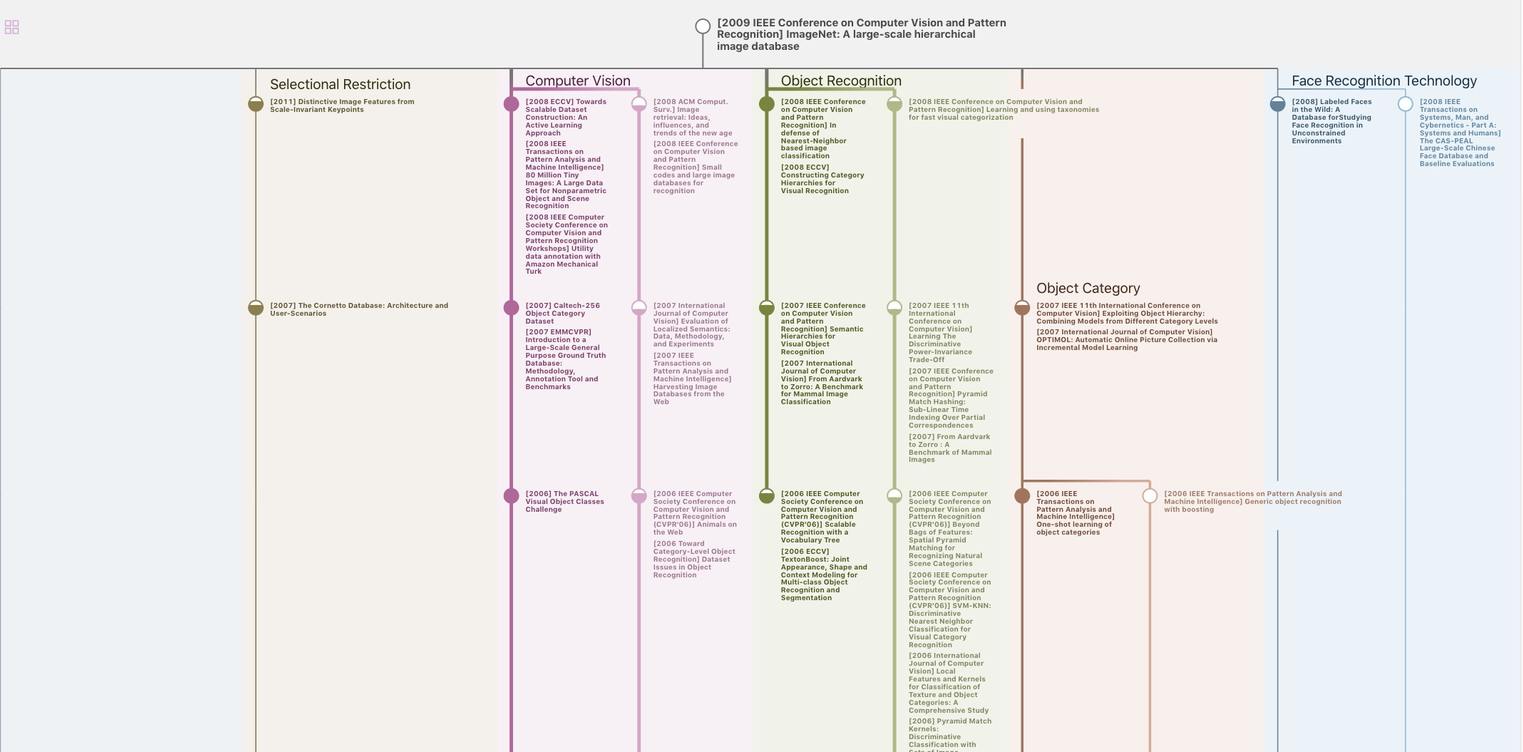
生成溯源树,研究论文发展脉络
Chat Paper
正在生成论文摘要