InvariantOODG: Learning Invariant Features of Point Clouds for Out-of-Distribution Generalization
CoRR(2024)
摘要
The convenience of 3D sensors has led to an increase in the use of 3D point
clouds in various applications. However, the differences in acquisition devices
or scenarios lead to divergence in the data distribution of point clouds, which
requires good generalization of point cloud representation learning methods.
While most previous methods rely on domain adaptation, which involves
fine-tuning pre-trained models on target domain data, this may not always be
feasible in real-world scenarios where target domain data may be unavailable.
To address this issue, we propose InvariantOODG, which learns invariability
between point clouds with different distributions using a two-branch network to
extract local-to-global features from original and augmented point clouds.
Specifically, to enhance local feature learning of point clouds, we define a
set of learnable anchor points that locate the most useful local regions and
two types of transformations to augment the input point clouds. The
experimental results demonstrate the effectiveness of the proposed model on 3D
domain generalization benchmarks.
更多查看译文
关键词
Point clouds,out of distribution generalization,invariance learning
AI 理解论文
溯源树
样例
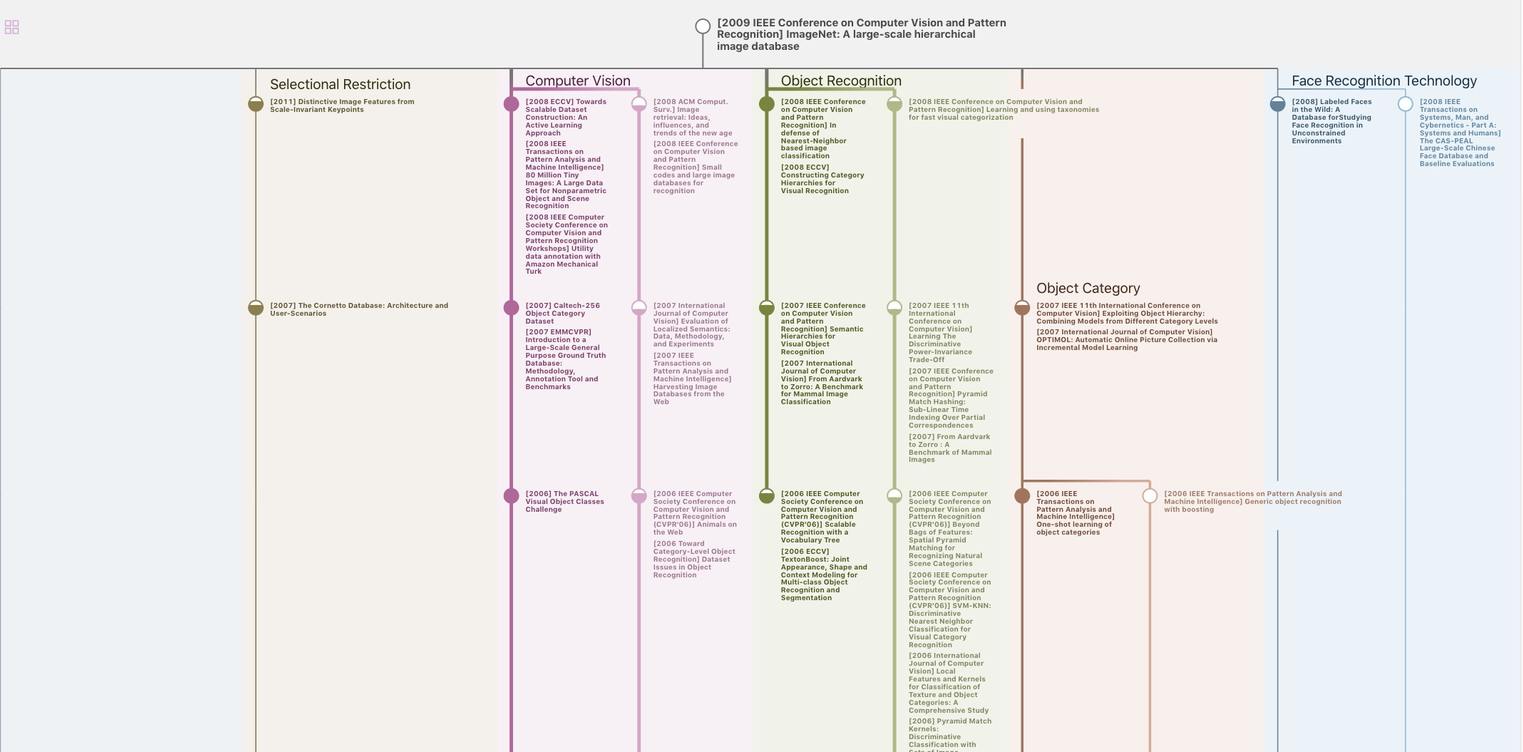
生成溯源树,研究论文发展脉络
Chat Paper
正在生成论文摘要