On Leveraging Large Language Models for Enhancing Entity Resolution
CoRR(2024)
摘要
Entity resolution, the task of identifying and consolidating records that
pertain to the same real-world entity, plays a pivotal role in various sectors
such as e-commerce, healthcare, and law enforcement. The emergence of Large
Language Models (LLMs) like GPT-4 has introduced a new dimension to this task,
leveraging their advanced linguistic capabilities. This paper explores the
potential of LLMs in the entity resolution process, shedding light on both
their advantages and the computational complexities associated with large-scale
matching. We introduce strategies for the efficient utilization of LLMs,
including the selection of an optimal set of matching questions, namely MQsSP,
which is proved to be a NP-hard problem. Our approach optimally chooses the
most effective matching questions while keep consumption limited to your budget
. Additionally, we propose a method to adjust the distribution of possible
partitions after receiving responses from LLMs, with the goal of reducing the
uncertainty of entity resolution. We evaluate the effectiveness of our approach
using entropy as a metric, and our experimental results demonstrate the
efficiency and effectiveness of our proposed methods, offering promising
prospects for real-world applications.
更多查看译文
AI 理解论文
溯源树
样例
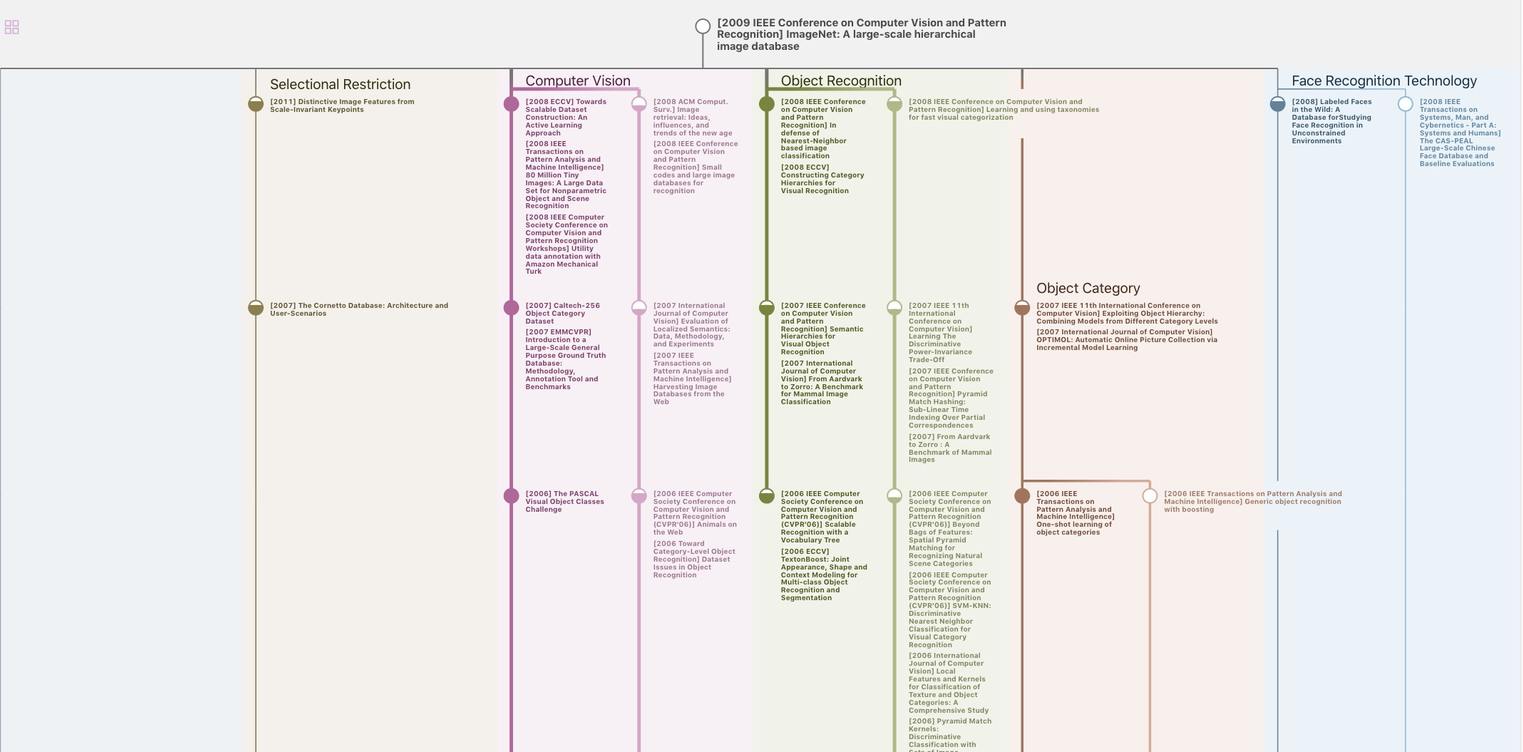
生成溯源树,研究论文发展脉络
Chat Paper
正在生成论文摘要