Physics-based vs. data-driven 24-hour probabilistic forecasts of precipitation for northern tropical Africa
arxiv(2024)
摘要
Numerical weather prediction (NWP) models struggle to skillfully predict
tropical precipitation occurrence and amount, calling for alternative
approaches. For instance, it has been shown that fairly simple, purely
data-driven logistic regression models for 24-hour precipitation occurrence
outperform both climatological and NWP forecasts for the West African summer
monsoon. More complex neural network based approaches, however, remain
underdeveloped due to the non-Gaussian character of precipitation. In this
study, we develop, apply and evaluate a novel two-stage approach, where we
train a U-Net convolutional neural network (CNN) model on gridded rainfall data
to obtain a deterministic forecast and then apply the recently developed,
nonparametric Easy Uncertainty Quantification (EasyUQ) approach to convert it
into a probabilistic forecast. We evaluate CNN+EasyUQ for one-day ahead 24-hour
accumulated precipitation forecasts over northern tropical Africa for
2011–2019, with the Integrated Multi-satellitE Retrievals for GPM (IMERG) data
serving as ground truth. In the most comprehensive assessment to date we
compare CNN+EasyUQ to state-of-the-art physics-based and data-driven approaches
such as a monthly probabilistic climatology, raw and postprocessed ensemble
forecasts from the European Centre for Medium-Range Weather Forecasts (ECMWF),
and traditional statistical approaches that use up to 25 predictor variables
from IMERG and the ERA5 reanalysis.Generally, statistical approaches perform
about en par with post-processed ECMWF ensemble forecasts. The CNN+EasyUQ
approach, however, clearly outperforms all competitors for both occurrence and
amount. Hybrid methods that merge CNN+EasyUQ and physics-based forecasts show
slight further improvement. Thus, the CNN+EasyUQ approach can likely improve
operational probabilistic forecasts of rainfall in the tropics, and potentially
even beyond.
更多查看译文
AI 理解论文
溯源树
样例
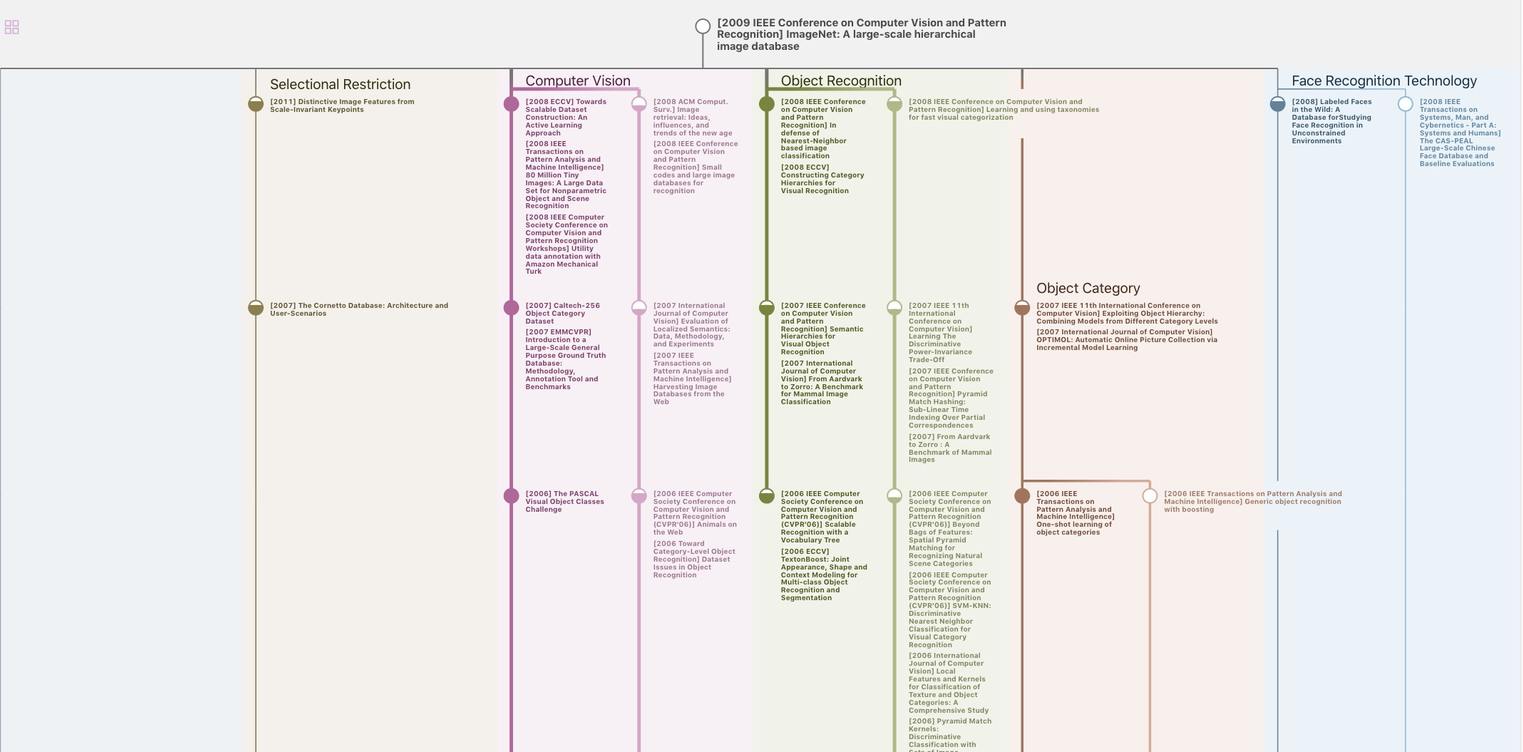
生成溯源树,研究论文发展脉络
Chat Paper
正在生成论文摘要