A non-asymptotic distributional theory of approximate message passing for sparse and robust regression
CoRR(2024)
摘要
Characterizing the distribution of high-dimensional statistical estimators is
a challenging task, due to the breakdown of classical asymptotic theory in high
dimension. This paper makes progress towards this by developing non-asymptotic
distributional characterizations for approximate message passing (AMP) – a
family of iterative algorithms that prove effective as both fast estimators and
powerful theoretical machinery – for both sparse and robust regression. Prior
AMP theory, which focused on high-dimensional asymptotics for the most part,
failed to describe the behavior of AMP when the number of iterations exceeds
o(log n/loglog n) (with n the sample size). We establish
the first finite-sample non-asymptotic distributional theory of AMP for both
sparse and robust regression that accommodates a polynomial number of
iterations. Our results derive approximate accuracy of Gaussian approximation
of the AMP iterates, which improves upon all prior results and implies enhanced
distributional characterizations for both optimally tuned Lasso and robust
M-estimator.
更多查看译文
AI 理解论文
溯源树
样例
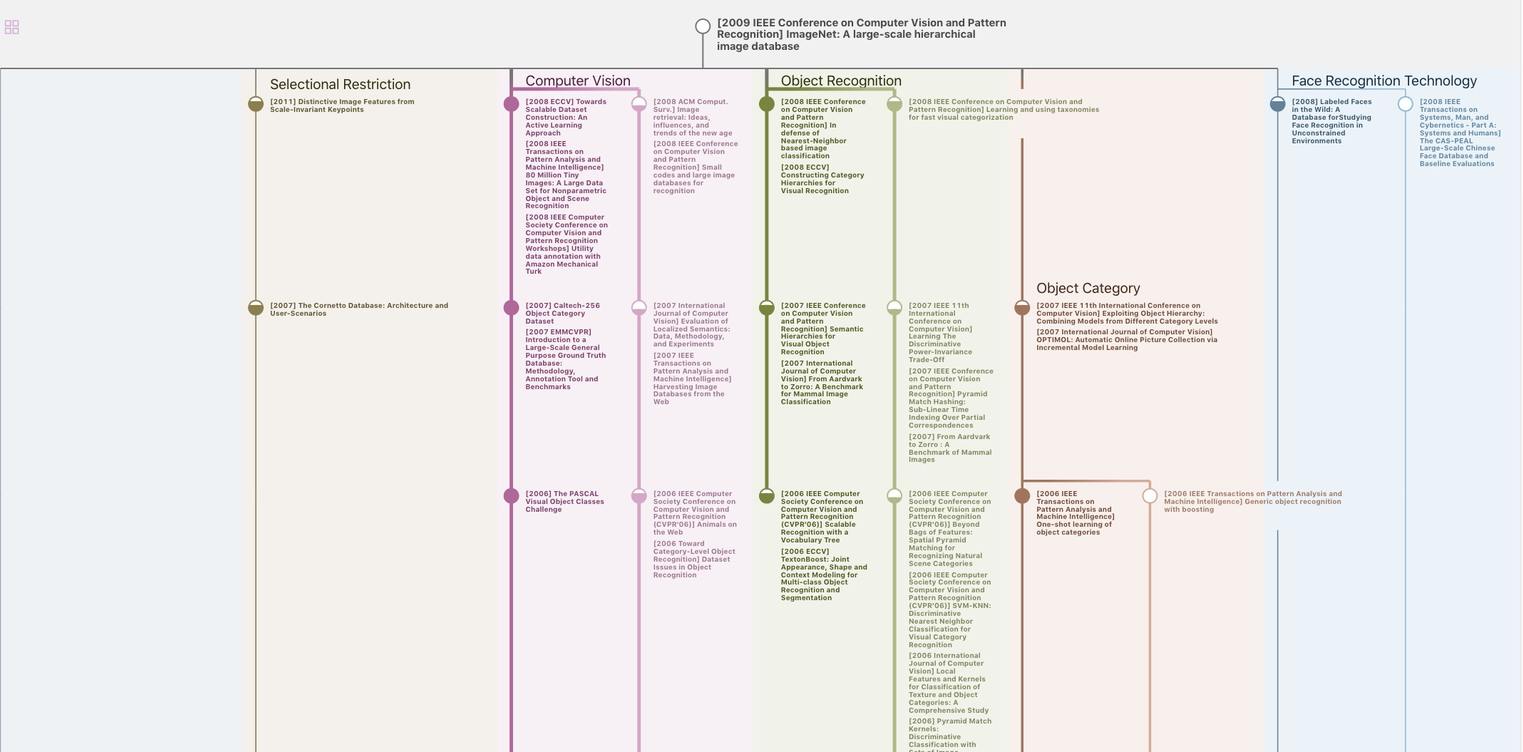
生成溯源树,研究论文发展脉络
Chat Paper
正在生成论文摘要