Joint item recommendation and trust prediction with graph neural networks
KNOWLEDGE-BASED SYSTEMS(2024)
摘要
Item recommendation and trust prediction are desired by users on social network platforms since they can help users find their favourite items or friends faster. Existing methods usually utilize users' social relationships to facilitate the item recommendation task and leverage users' preferences to assist the trust prediction task. While sociological researchers have shown that users' preferences and users' social relationships are not isolated but influenced by each other, there are a few studies that model these two tasks jointly. However, in these studies, the incorporation of distrust relations and the dynamic item-specific mutual influence between users' preferences and users' social relationships are ignored. In this paper, we propose a joint learning method using graph neural networks for item recommendation and trust prediction tasks, named JoRTGNN. First, users' distrust relations are incorporated along with trust relations to extract more potential user social relationships, which can benefit both the item recommendation and trust prediction tasks. In addition, a dynamic item-specific mutual influence between users' preferences and users' social relationships is highlighted with attention mechanisms for the joint learning tasks. Experiments have been conducted on the Epinions datasets, and the experimental results show a superior performance of JoRTGNN over baselines in item recommendation and trust prediction tasks, which demonstrates that item recommendation and trust prediction can be effectively improved in the process of the mutual influence between users' preferences and users' social relationships.
更多查看译文
关键词
Recommendation systems,Social relationships,Graph neural networks
AI 理解论文
溯源树
样例
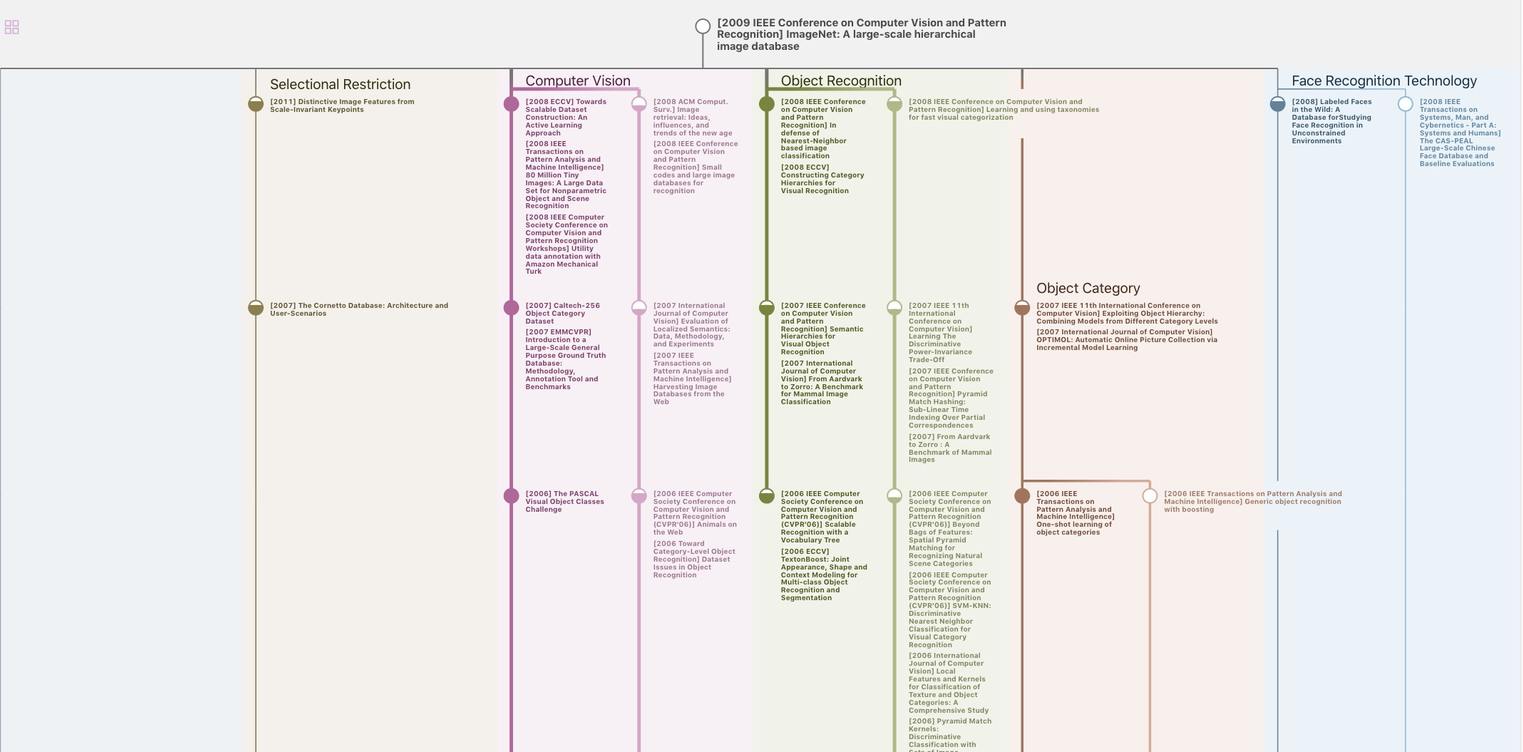
生成溯源树,研究论文发展脉络
Chat Paper
正在生成论文摘要