CVTStego-Net: A convolutional vision transformer architecture for spatial image steganalysis
JOURNAL OF INFORMATION SECURITY AND APPLICATIONS(2024)
摘要
The principal investigations in image steganalysis in the spatial domain have concentrated on convolutional neural network (CNN) designs. However, existing CNNs increase the local receptive field of steganographic noise without considering global steganographic noise. This study introduces CVTStego-Net, a convolutional vision transformer for spatial domain image steganalysis that merges the strengths of convolutions and the advantages of attention mechanisms to capture both local and global dependencies. CVTStego-Net is composed of three stages: preprocessing stage, noise extraction, and analysis stage, and classification stage. The preprocessing stage involves a bifurcation with trainable and untrainable 30 SRM (Spatial Rich Models) filters to enhance steganographic noise. The noise extraction and analysis stage combines the SE-Block (Squeezeand-Excitation) with residual operations to increase the sensitivity to steganographic noise and suppressing the influence of redundant information, and the classification stage combines SE-Block with a convolutional vision transformer to connect the local and global spatial relationships of the steganographic noise. This work enhanced the classification accuracies for steganographic algorithms compared to YEDROUDJ-Net, SR-Net, ZHU-Net, GBRAS-Net, and SNMC-Net. Specifically, the accuracy of CVTStego-Net for WOW at 0.2 bpp was 86.58%, and 0.4 bpp was 93.80%. Moreover, for S-UNIWARD at 0.2 and 0.4 bpp, the accuracies were 80.70% and 90.45%, respectively. For MiPOD at 0.2 and 0.4 bpp, the accuracies were 74.70% and 81.48%, respectively. For HILL at 0.2 and 0.4 bpp, the accuracies were 76.70% and 85.80%, respectively, and for HUGO at 0.2 and 0.4 bpp, the accuracies were 78.20% and 86.98%, respectively, using test data from the BOSSbase 1.01. The results demonstrate that convolutional vision transformers can classify steganographic images in the spatial domain.
更多查看译文
关键词
Convolutional vision transformer,CVTStego-net,Convolutional neural network,Deep learning,Steganalysis,Steganography
AI 理解论文
溯源树
样例
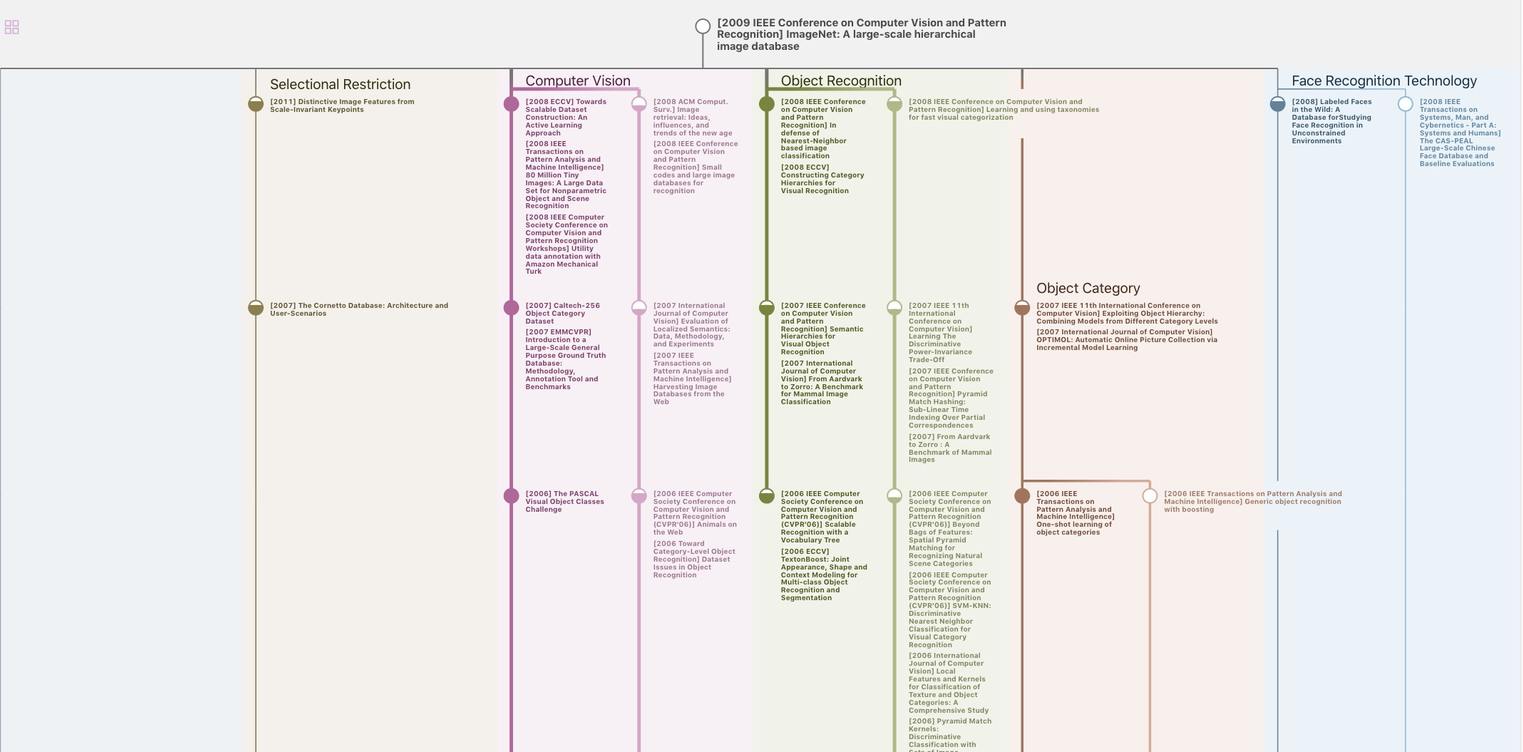
生成溯源树,研究论文发展脉络
Chat Paper
正在生成论文摘要