Preoperative 3-dimensional computed tomography bone density measures provide objective bone quality classifications for stemless anatomic total shoulder arthroplasty.
Journal of shoulder and elbow surgery(2024)
摘要
BACKGROUND:Reproducible methods for determining adequate bone densities for stemless anatomic total shoulder arthroplasty (aTSA) are currently lacking. The purpose of this study was to evaluate the utility of preoperative computed tomography (CT) imaging for assessing the bone density of the proximal humerus for supportive differentiation in the decision making for stemless humeral component implantation. It was hypothesized that preoperative 3-dimensional (3-D) CT bone density measures provide objective classifications of the bone quality for stemless aTSA.
METHODS:A 3-part study was performed that included the analysis of cadaveric humerus CT scans followed by retrospective application to a clinical cohort and classification with a machine learning model. Thirty cadaveric humeri were evaluated with clinical CT and micro-CT (μCT) imaging. Phantom-calibrated CT data were used to extract 3-D regions of interest and defined radiographic scores. The final image processing script was applied retrospectively to a clinical cohort (n = 150) that had a preoperative CT and intraoperative bone density assessment using the "thumb test," followed by placement of an anatomic stemmed or stemless humeral component. Postscan patient-specific calibration was used to improve the functionality and accuracy of the density analysis. A machine learning model (Support vector machine [SVM]) was utilized to improve the classification of bone densities for a stemless humeral component.
RESULTS:The image processing of clinical CT images demonstrated good to excellent accuracy for cylindrical cancellous bone densities (metaphysis [ICC = 0.986] and epiphysis [ICC = 0.883]). Patient-specific internal calibration significantly reduced biases and unwanted variance compared with standard HU CT scans (P < .0001). The SVM showed optimized prediction accuracy compared with conventional statistics with an accuracy of 73.9% and an AUC of 0.83 based on the intraoperative decision of the surgeon. The SVM model based on density clusters increased the accuracy of the bone quality classification to 87.3% with an AUC of 0.93.
CONCLUSIONS:Preoperative CT imaging allows accurate evaluation of the bone densities in the proximal humerus. Three-dimensional regions of interest, rescaling using patient-specific calibration, and a machine learning model resulted in good to excellent prediction for objective bone quality classification. This approach may provide an objective tool extending preoperative selection criteria for stemless humeral component implantation.
更多查看译文
AI 理解论文
溯源树
样例
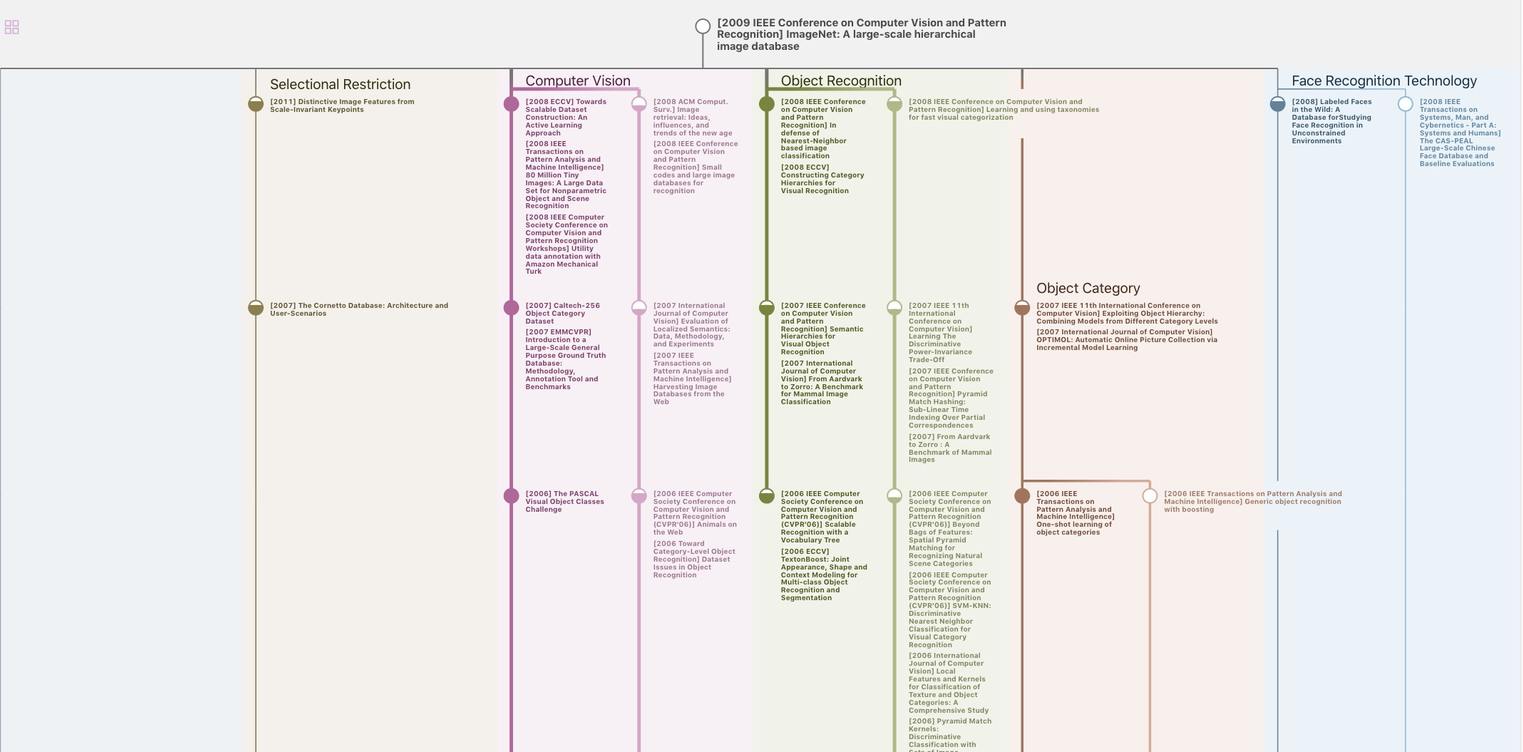
生成溯源树,研究论文发展脉络
Chat Paper
正在生成论文摘要