A dynamic nomogram for predicting intraoperative brain bulge during decompressive craniectomy in patients with traumatic brain injury: a retrospective study
INTERNATIONAL JOURNAL OF SURGERY(2024)
摘要
Objective:The aim of this paper is to investigate the risk factors associated with intraoperative brain bulge (IOBB), especially the computed tomography (CT) value of the diseased lateral transverse sinus, and to develop a reliable predictive model to alert neurosurgeons to the possibility of IOBB.Methods:A retrospective analysis was performed on 937 patients undergoing traumatic decompressive craniectomy. A total of 644 patients from Fuzong Clinical Medical College of Fujian Medical University were included in the development cohort, and 293 patients from the First Affiliated Hospital of Shantou University Medical College were included in the external validation cohort. Univariate and multifactorial logistic regression analyses identified independent risk factors associated with IOBB. The logistic regression models consisted of independent risk factors, and receiver operating characteristic curves, calibration, and decision curve analyses were used to assess the performance of the models. Various machine learning models were used to compare with the logistic regression model and analyze the importance of the factors, which were eventually jointly developed into a dynamic nomogram for predicting IOBB and published online in the form of a simple calculator.Results:IOBB occurred in 93/644 (14.4%) patients in the developmental cohort and 47/293 (16.0%) in the validation cohort. Univariate and multifactorial regression analyses showed that age, subdural hematoma, contralateral fracture, brain contusion, and CT value of the diseased lateral transverse sinus were associated with IOBB. A logistic regression model (full model) consisting of the above risk factors had excellent predictive power in both the development cohort [area under the curve (AUC)=0.930] and the validation cohort (AUC=0.913). Among the four machine learning models, the AdaBoost model showed the best predictive value (AUC=0.998). Factors in the AdaBoost model were ranked by importance and combined with the full model to create a dynamic nomogram for clinical application, which was published online as a practical and easy-to-use calculator.Conclusions:The CT value of the diseased lateral transverse is an independent risk factor and a reliable predictor of IOBB. The online dynamic nomogram formed by combining logistic regression analysis models and machine learning models can more accurately predict the possibility of IOBBs in patients undergoing traumatic decompressive craniectomy.
更多查看译文
关键词
decompressive craniectomy,intraoperative brain bulge,machine learning,nomogram,traumatic brain injury
AI 理解论文
溯源树
样例
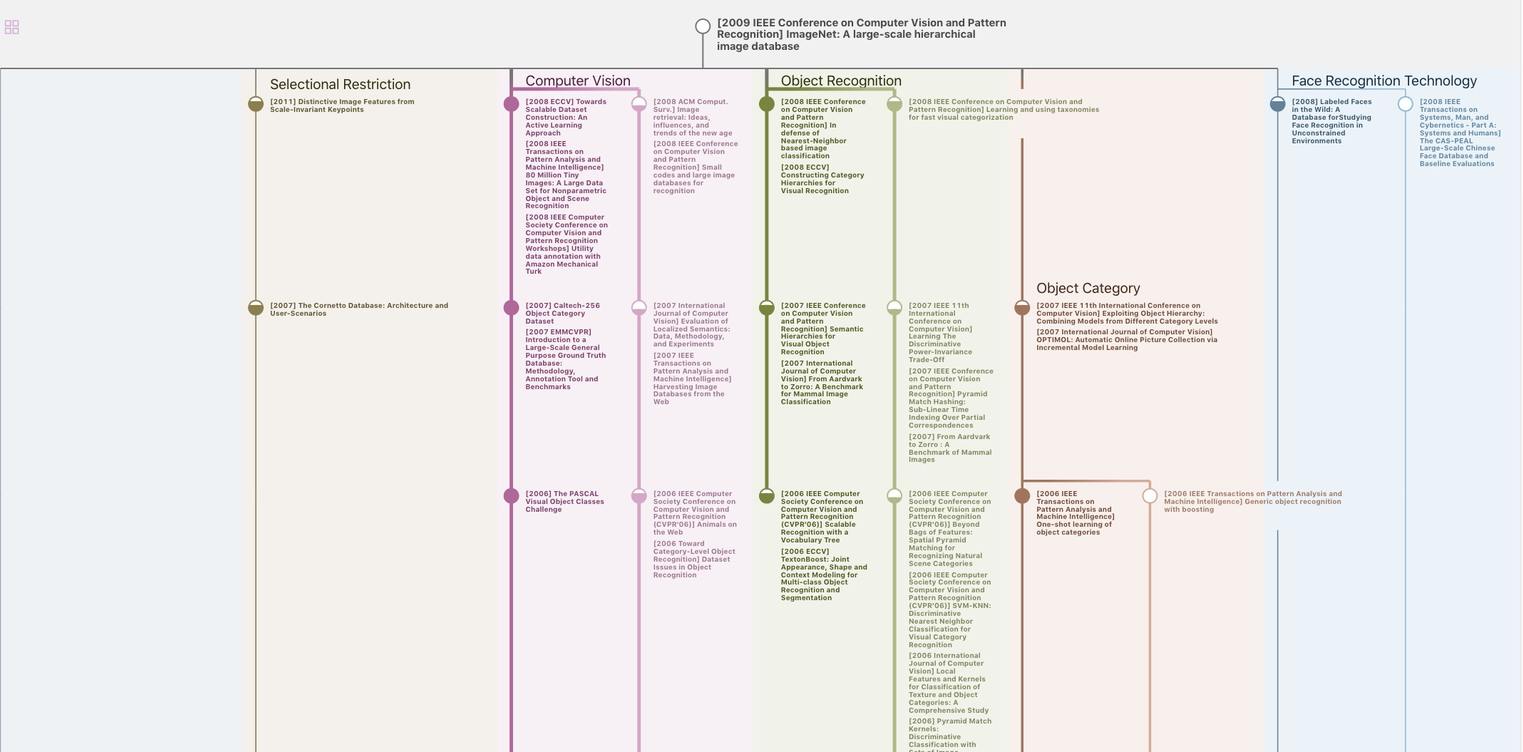
生成溯源树,研究论文发展脉络
Chat Paper
正在生成论文摘要