Heuristic Search Optimisation Using Planning and Curriculum Learning Techniques
PROGRESS IN ARTIFICIAL INTELLIGENCE, EPIA 2023, PT I(2023)
摘要
Learning a well-informed heuristic function for hard planning domains is an elusive problem. Although there are known neural network architectures to represent such heuristic knowledge, it is not obvious what concrete information is learned and whether techniques aimed at understanding the structure help in improving the quality of the heuristics. This paper presents a network model that learns a heuristic function capable of relating distant parts of the state space via optimal plan imitation using the attention mechanism which drastically improves the learning of a good heuristic function. The learning of this heuristic function is further improved by the use of curriculum learning, where newly solved problem instances are added to the training set, which, in turn, helps to solve problems of higher complexities and train from harder problem instances. The methodologies used in this paper far exceed the performances of all existing baselines including known deep learning approaches and classical planning heuristics. We demonstrate its effectiveness and success on grid-type PDDL domains, namely Sokoban, maze-with-teleports and sliding tile puzzles.
更多查看译文
关键词
Planning,Optimizing heuristic functions,Deep learning
AI 理解论文
溯源树
样例
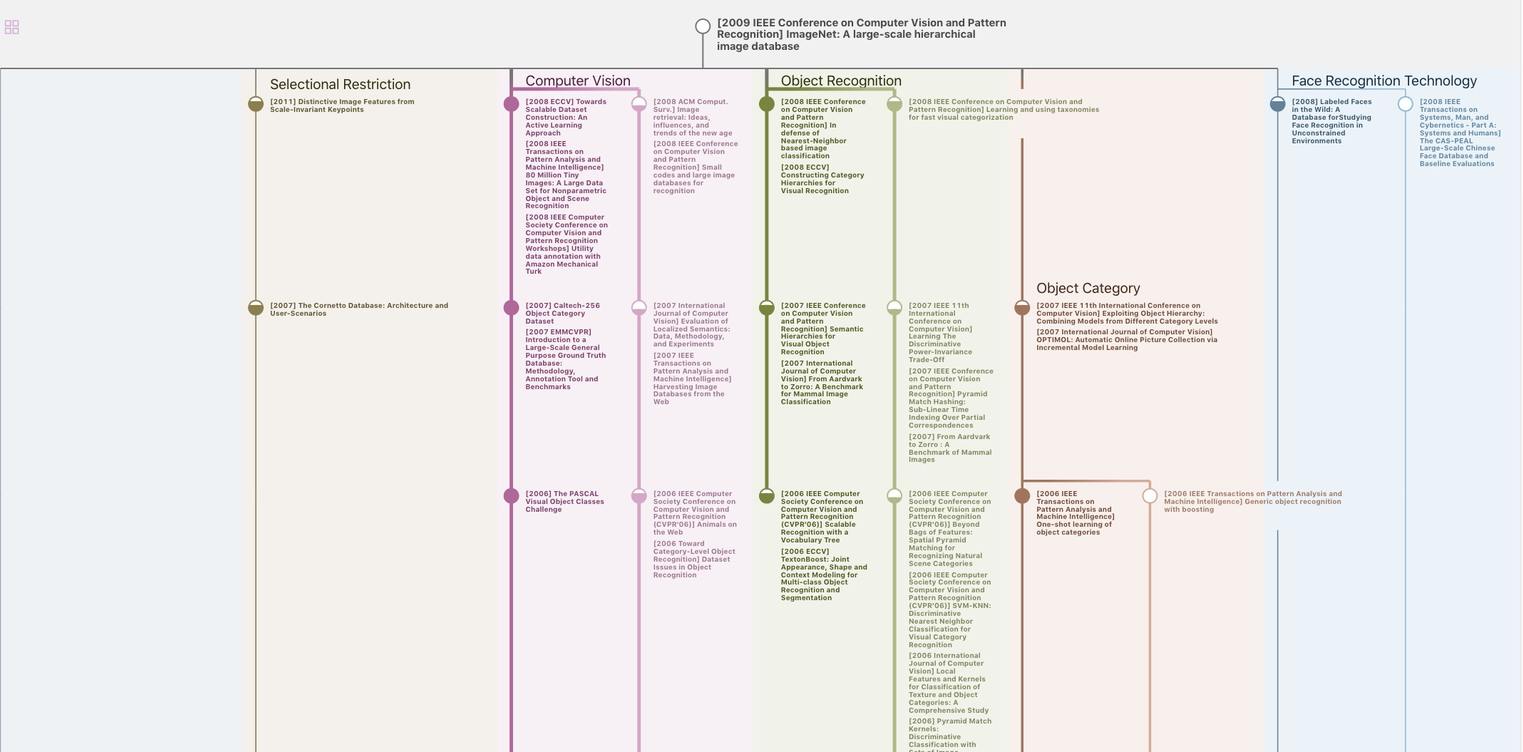
生成溯源树,研究论文发展脉络
Chat Paper
正在生成论文摘要