Imbalanced Regression Evaluation Under Uncertain Domain Preferences
PROGRESS IN ARTIFICIAL INTELLIGENCE, EPIA 2023, PT I(2023)
摘要
In natural phenomena, data distributions often deviate from normality. One can think of cataclysms as a self-explanatory example: rarely occurring events differ considerably from common outcomes. In real-world domains, such tail events are often the most relevant to anticipate, allowing us to take adequate measures to prevent or attenuate their impact on society. However, mapping target values to particular relevance judgements is challenging and existing methods do not consider the impact of bias in reaching such mappings-relevance functions. In this paper, we tackle the issue of uncertainty in non-uniform domain preferences and its impact on imbalanced regression evaluation. Specifically, we develop two methods for assessing the volatility of model performance when dealing with uncertainty regarding the range of target values that are more important to the underlying problem. We demonstrate the importance of our proposed methods in capturing the impact of small changes in relevance assessments of target values and how they may impact experimental conclusions.
更多查看译文
AI 理解论文
溯源树
样例
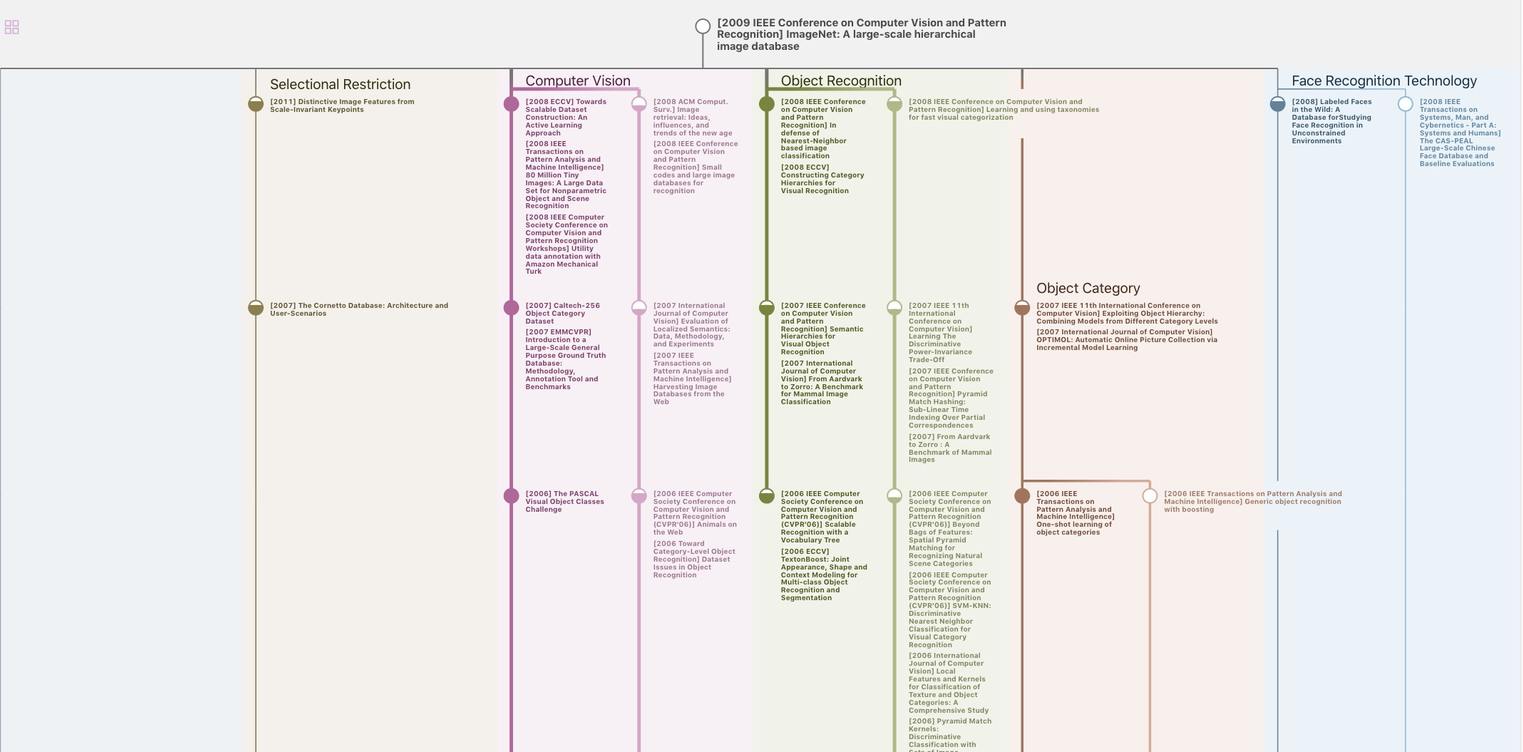
生成溯源树,研究论文发展脉络
Chat Paper
正在生成论文摘要