Multi-Task Self-Blended Images for Face Forgery Detection.
ACM Multimedia Asia(2023)
摘要
Deepfake detection has attracted extensive attention due to widespread forged images on social media. Recently, self-supervised learning (SSL) based Deepfake detection approaches have outperformed supervised methods in terms of model generalization. However, we notice that most SSL-based methods do not take the manipulation strength levels of synthesized forgery samples into consideration according to different synthesis parameters and result in suboptimal detection performances. To address this issue, we introduce several auxiliary losses to the state-of-the-art SSL-based method based on different synthesis sub-tasks during data generation by inferring their synthesis parameters where the ground-truth labels are obtained from the synthesis pipeline for free. With comprehensive evaluations on various benchmarks, our approach has achieved noticeable performance improvement. Specifically, for the cross-dataset evaluation, the proposed approach outperforms the state-of-the-art method in terms of AUC on various datasets with improvements of 3.4%, 1.47%, 1.56%, and 1.3% on the CDF, DFDC, DFDCP, and FFIW datasets and achieves competitive performance on the DFD dataset. This further demonstrates the effectiveness of the proposed approach in its generalization ability.
更多查看译文
AI 理解论文
溯源树
样例
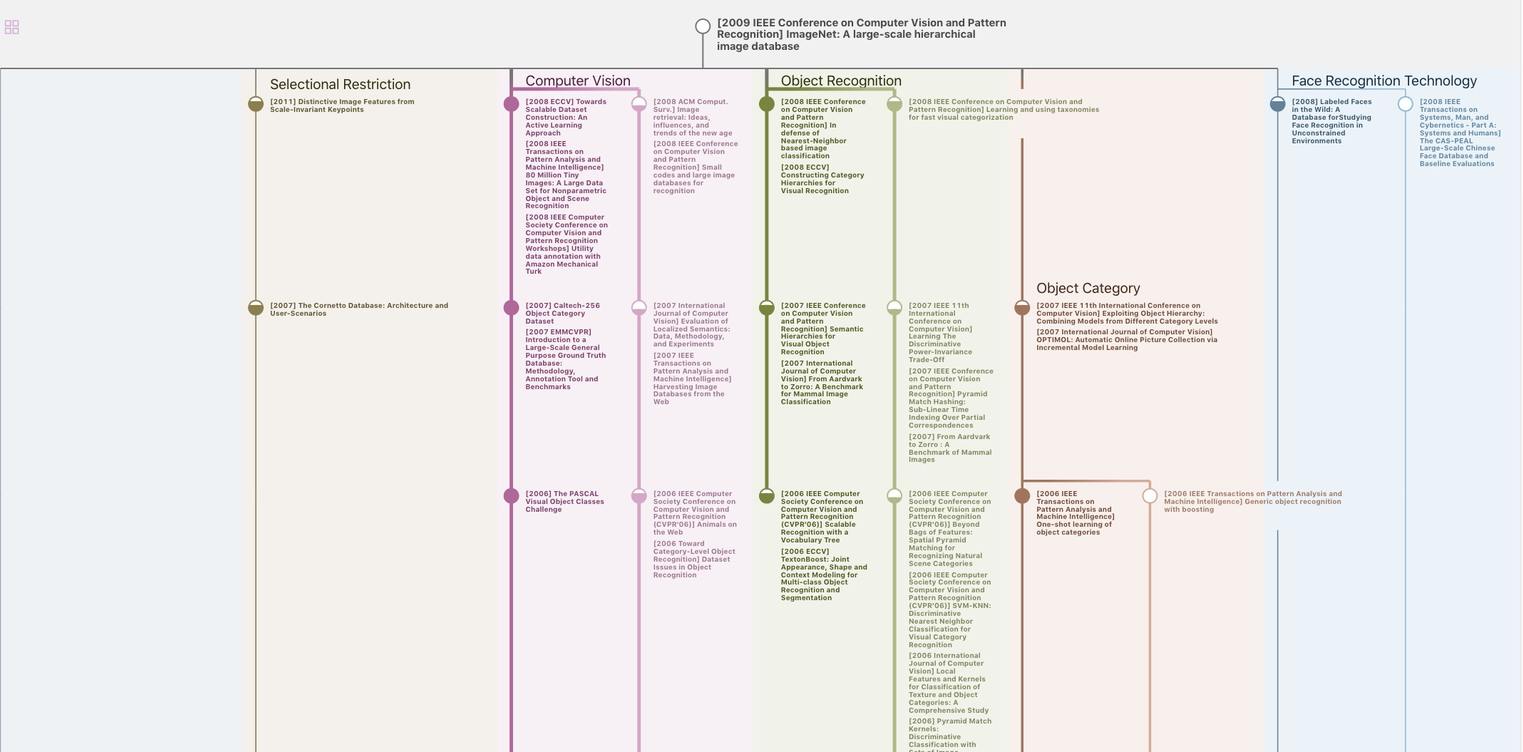
生成溯源树,研究论文发展脉络
Chat Paper
正在生成论文摘要