A city-agnostic framework to determine optimal location and sizing of electric vehicle charge stations
PROCEEDINGS OF 7TH JOINT INTERNATIONAL CONFERENCE ON DATA SCIENCE AND MANAGEMENT OF DATA, CODS-COMAD 2024(2024)
摘要
In this paper, we demonstrate a generalizable city-agnostic optimization framework and a supporting user interface to identify and visualize optimal locations and sizing of electric vehicle (EV) charge stations, with the following components: a data retrieval module, an EV demand projection module and an optimizer module. The data retrieval module retrieves city-specific data from open-source data repositories like OpenChargeMap, OpenStreetMap etc., while the EV demand projection module employs graph analysis and machine learning techniques to predict the spatial spread of EV charging demand in the target city. The optimizer module formulates and solves an MILP (mixed integer linear program) to identify the optimal location and sizing of EV charge stations. The supporting UI allows a business user to rapidly visualize and analyse multiple what-if scenarios with minimal intervention, thus enabling faster understanding and effective implementation.
更多查看译文
关键词
electric vehicles (EVs),optimization,optimal planning,siting and sizing,city-agnostic
AI 理解论文
溯源树
样例
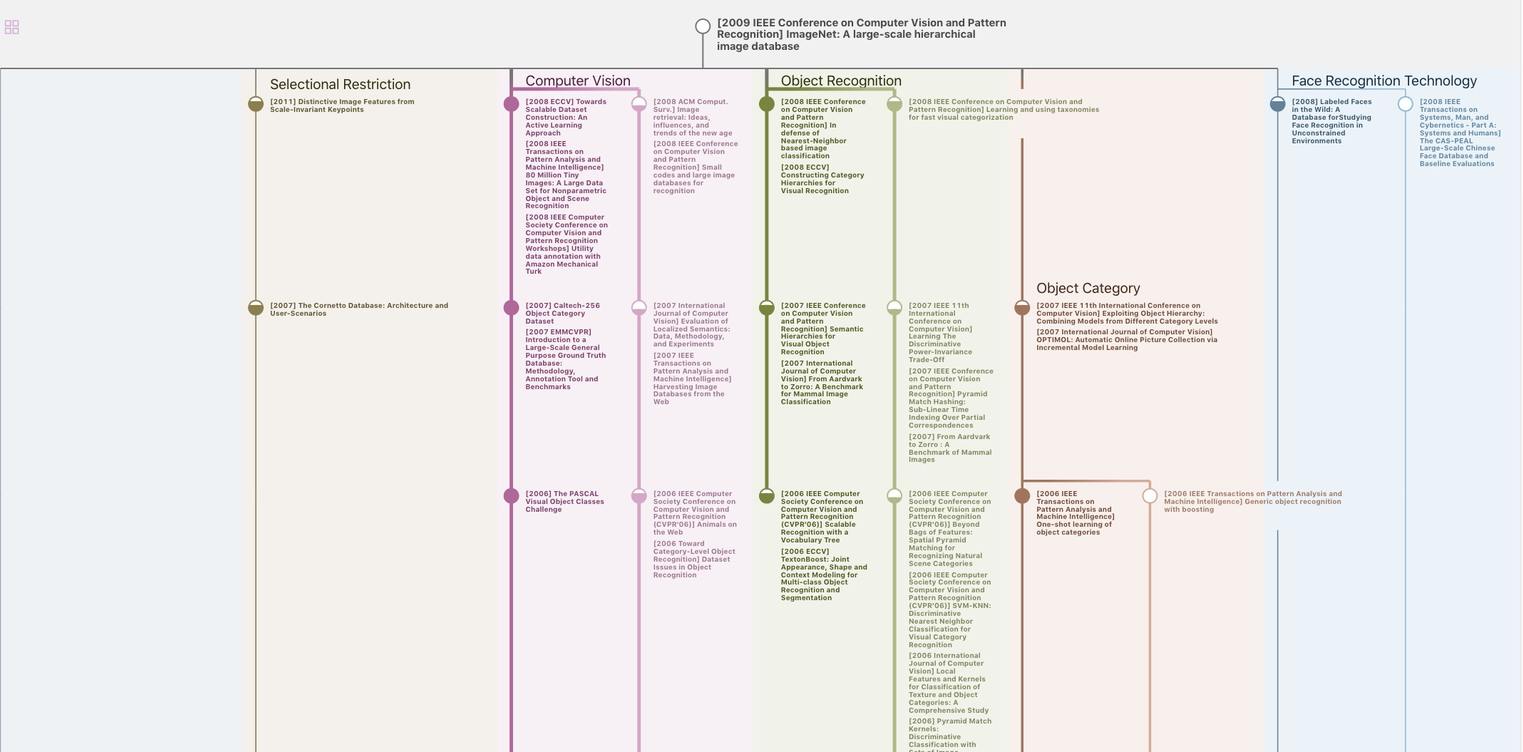
生成溯源树,研究论文发展脉络
Chat Paper
正在生成论文摘要