ARGO - An AI Based Responsible Gamification Framework for Online Skill Gaming Platform
PROCEEDINGS OF 7TH JOINT INTERNATIONAL CONFERENCE ON DATA SCIENCE AND MANAGEMENT OF DATA, CODS-COMAD 2024(2024)
摘要
Games of skill provide an excellent source of entertainment to realize self-esteem, relaxation and social gratification. Engagement in online skill gaming platforms is however heavily dependent on the outcomes and experience (e.g., wins/losses). There is an inherent tension between being: (i) demotivated (and hence dis-engaged) and ultra-motivated (to chase wins) in response to losses; or (ii) positively engaged and over-indulged under a winning streak. These extreme transient intents make any gamification effort non-trivial in outcome-oriented platforms because of the inherent trade-off between engagement and prudence (avoiding over-indulgence). Providing long challenges and high milestones can generate immediate engagement bump while adversely impacting its sustenance (resulting in possible churn eventually) depending on the players' intent. Unlike in most consumer internet platforms, the intent and relevancy (challenge completion) become intrinsically orthogonal. This paper presents ARGO, a deep learning based responsible gamification framework, which: (i) learns players' long term "game behaviours" (as psychological imprints emanating from game play evolution), via SOTA collaborative neural network, CognitionNet; (ii) succinctly combines these game behaviours to estimate players' inherent "intent" towards a challenge, via an engagement guided regressor; and (iii) addresses the issue in building an imbalanced classification model for challenge completion via quantile regression with a novel data augmentation trick. Using online Rummy as a case-study, we show that the player engagement can be maximized based on both intent and challenge completion likelihood. Personalizing the challenge milestones accordingly results in-15% lift in milestone completion, 14% decline in unsuccessful target chasing with 4% improvements in target completion rates for the target chasers and 7% reduction in loss chasing and 5% improvement in win rates for the over-indulging players. We contend that ARGO is first-of-a-kind framework for outcome-oriented platforms that-performs personalized gamification contingent to both: (a) players' transient intent and (b) challenge relevancy-thus balancing the trade-off between engagement and over-indulgence.
更多查看译文
关键词
Time Series,Clustering,Gamification,Neural Networks
AI 理解论文
溯源树
样例
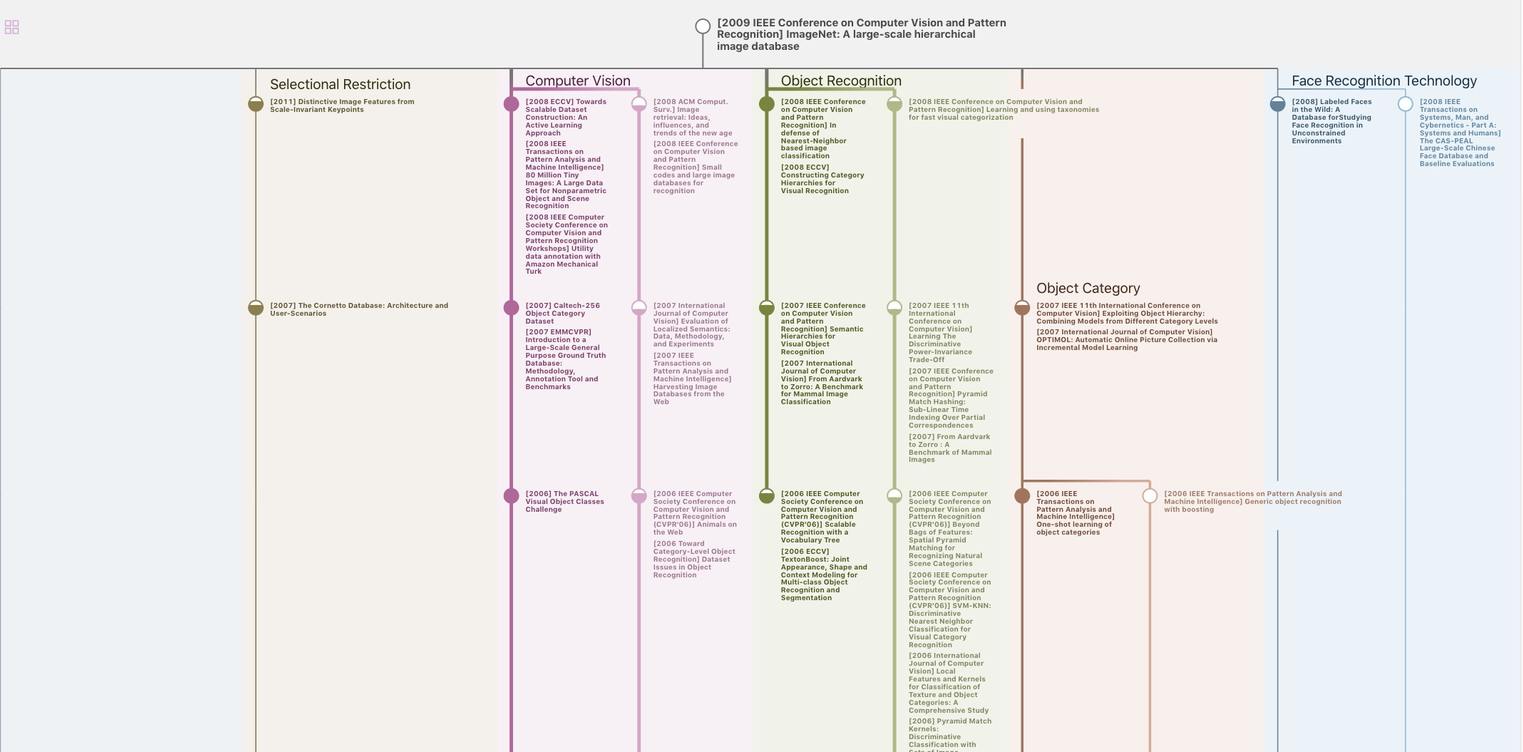
生成溯源树,研究论文发展脉络
Chat Paper
正在生成论文摘要