Multiforecast-based Early Anomaly Detection for Spacecraft Health Monitoring
PROCEEDINGS OF 7TH JOINT INTERNATIONAL CONFERENCE ON DATA SCIENCE AND MANAGEMENT OF DATA, CODS-COMAD 2024(2024)
摘要
Early detection of impending anomalies is a strong desirable for spacecraft operation as it can allow preemptive action to safeguard the mission objectives. Methods abound for just-in-time anomaly detection but early detection is a much more sought after goal. In this paper, we present MEND, a simple-yet-powerful model for early anomaly detection for spacecraft health monitoring. In experiments, MEND was able to provide strong alerts for impending anomalies as much as 10-15 minutes before the onset of the anomaly which could give a system admin valuable time to perform curative action. It is notable that none of the other models considered, including state-of-the-art zero-shot time series prediction models, were able to achieve this. MEND is based on simple, explainable elements such as self-supervised operation-mode detection and self-disagreement-based anomaly detection which, as a side effect, offer insights that may aid root cause analysis and may be of independent interest. Code for MEND is available at https://github.com/purushottamkar/mend
更多查看译文
关键词
spacecraft health monitoring,time series learning,anomaly detection,self-disagreement,clustering,regression analysis
AI 理解论文
溯源树
样例
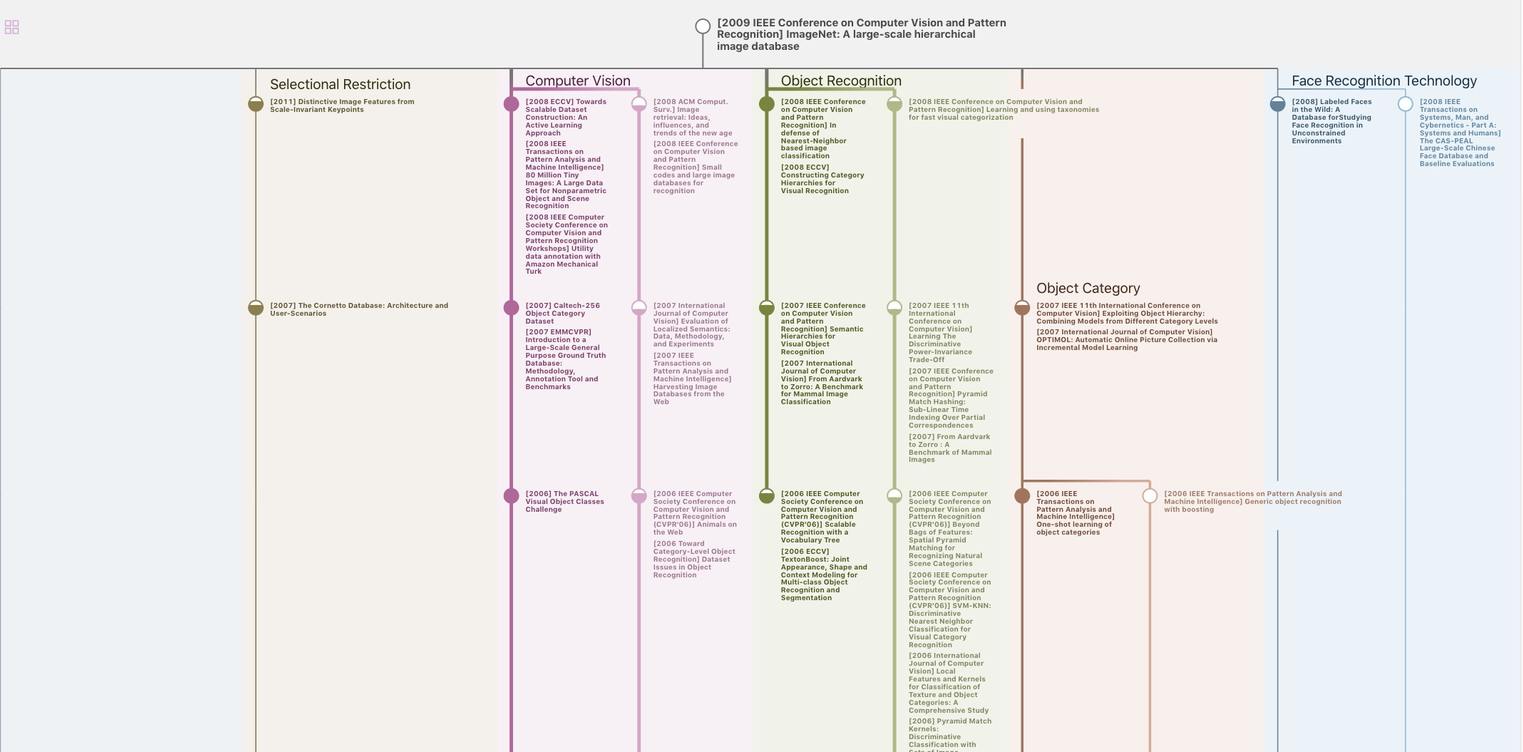
生成溯源树,研究论文发展脉络
Chat Paper
正在生成论文摘要