Robust Shape-regularized Non-negative Matrix Factorization for Real-time Source Apportionment
PROCEEDINGS OF 7TH JOINT INTERNATIONAL CONFERENCE ON DATA SCIENCE AND MANAGEMENT OF DATA, CODS-COMAD 2024(2024)
摘要
Discovering the anthropogenic sources of pollution is a key step in air quality monitoring and management. Real-time source apportionment (RTSA) is the globally accepted standard for this task and critically uses non-negative matrix factorization (NMF) that is made challenging by the non-convexity of the problem and noisy data. In this work, we develop a technique ROSE-NMF offering improvements over state-of-the-art RTSA and NMF solvers. ROSE-NMF offers provable convergence guarantees, allows diurnal patterns to to be specified to guide the solver to an optimal solution, and is robust to row and column outliers. In multiple experiments, ROSE-NMF offered markedly improved performance over standard NMF solvers as well as commercial RTSA solvers. Code for ROSE-NMF is available at https://github.com/purushottamkar/rose-nmf.
更多查看译文
关键词
non-negative matrix factorization,shape regularization,robust learning,source apportionment,air-quality monitoring
AI 理解论文
溯源树
样例
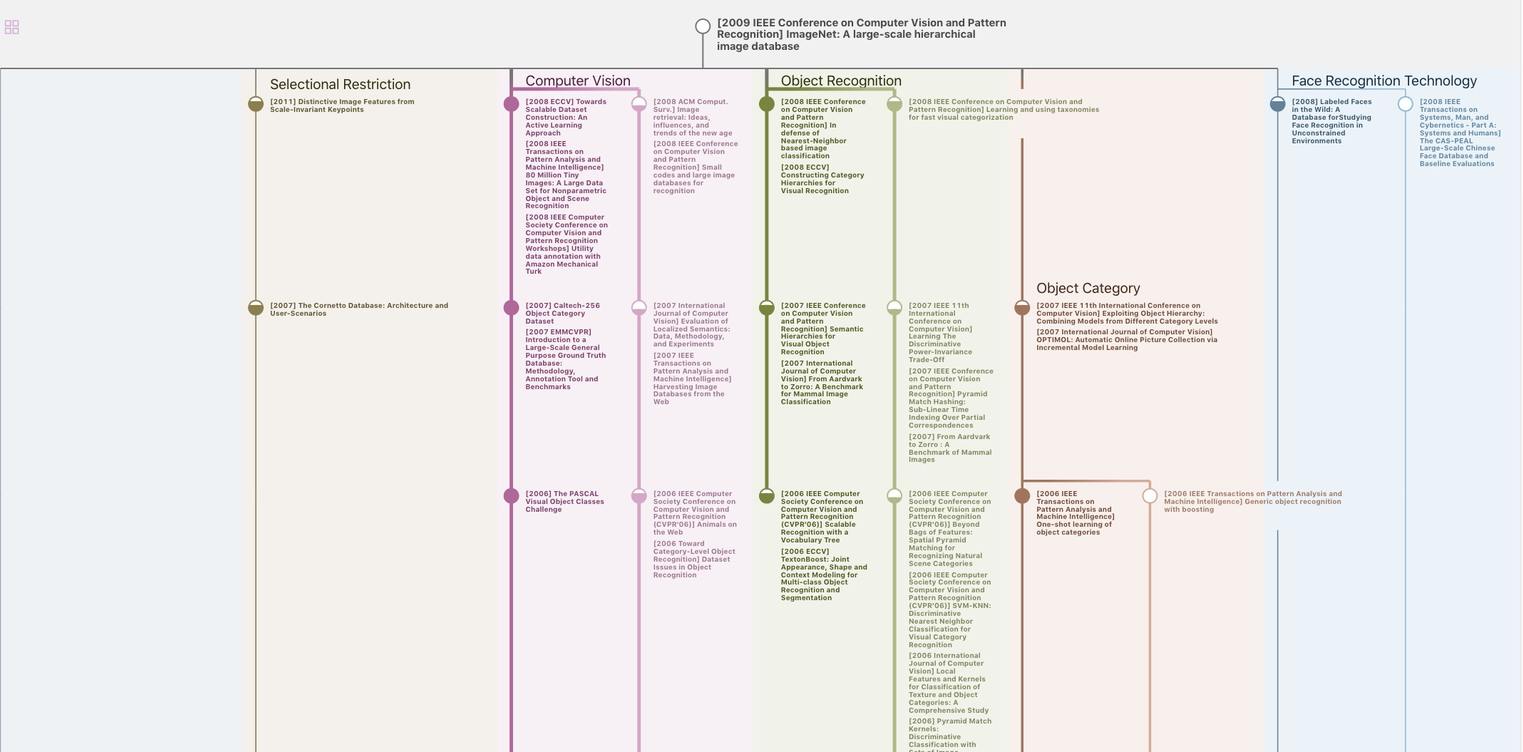
生成溯源树,研究论文发展脉络
Chat Paper
正在生成论文摘要