Tackling Electrode Shift In Gesture Recognition with HD-EMG Electrode Subsets
CoRR(2024)
摘要
sEMG pattern recognition algorithms have been explored extensively in
decoding movement intent, yet are known to be vulnerable to changing recording
conditions, exhibiting significant drops in performance across subjects, and
even across sessions. Multi-channel surface EMG, also referred to as
high-density sEMG (HD-sEMG) systems, have been used to improve performance with
the information collected through the use of additional electrodes. However, a
lack of robustness is ever present due to limited datasets and the difficulties
in addressing sources of variability, such as electrode placement. In this
study, we propose training on a collection of input channel subsets and
augmenting our training distribution with data from different electrode
locations, simultaneously targeting electrode shift and reducing input
dimensionality. Our method increases robustness against electrode shift and
results in significantly higher intersession performance across subjects and
classification algorithms.
更多查看译文
关键词
electromyography,gesture recognition,generalization,electrode shift
AI 理解论文
溯源树
样例
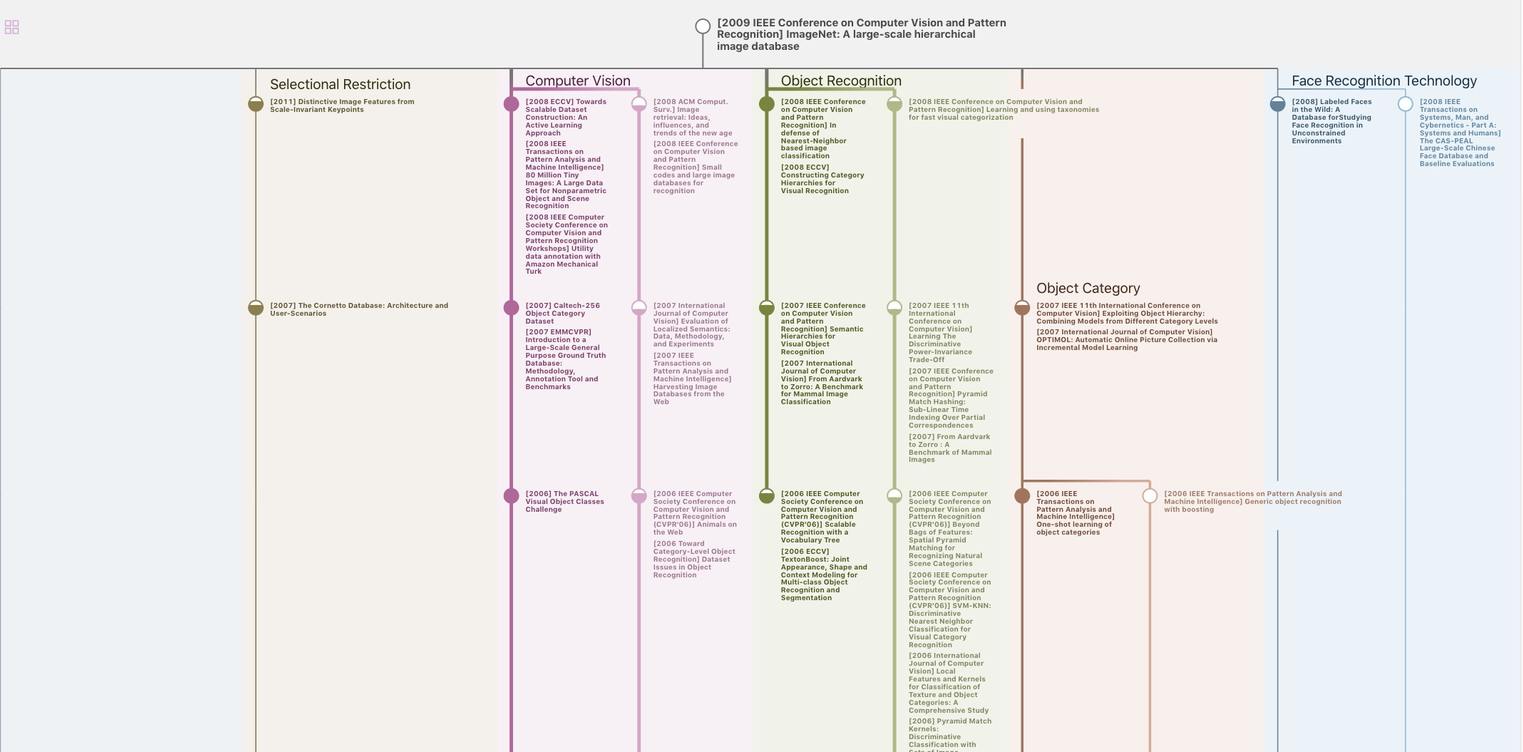
生成溯源树,研究论文发展脉络
Chat Paper
正在生成论文摘要