Spatial Patterning Analysis of Cellular Ensembles (SPACE) enables statistically robust discovery of complex spatial organization at the cell and tissue level.
bioRxiv : the preprint server for biology(2023)
摘要
Spatial patterns of cells and other entities drive both physiologic and pathologic processes within tissues. While many imaging and transcriptomic methods document tissue organization, discerning these patterns is challenging, especially when they involve multiple entities in complex arrangements. To address this challenge, we present Spatial Patterning Analysis of Cellular Ensembles (SPACE), an R package for analysis of high-plex tissue images generated using any collection modality. Unlike existing platforms, SPACE detects context-dependent associations, quantitative gradients and orientations, and other organizational complexities. Via a robust information theoretic framework, SPACE explores all possible ensembles - single entities, pairs, triplets, and so on - and ranks the strongest patterns of tissue organization. Using lymph node images for which ground truth has been defined, we validate SPACE and demonstrate its advantages. We then use SPACE to reanalyze a public dataset of human tuberculosis granulomas, verifying known patterns and discovering new patterns with possible insights into disease progression.
更多查看译文
AI 理解论文
溯源树
样例
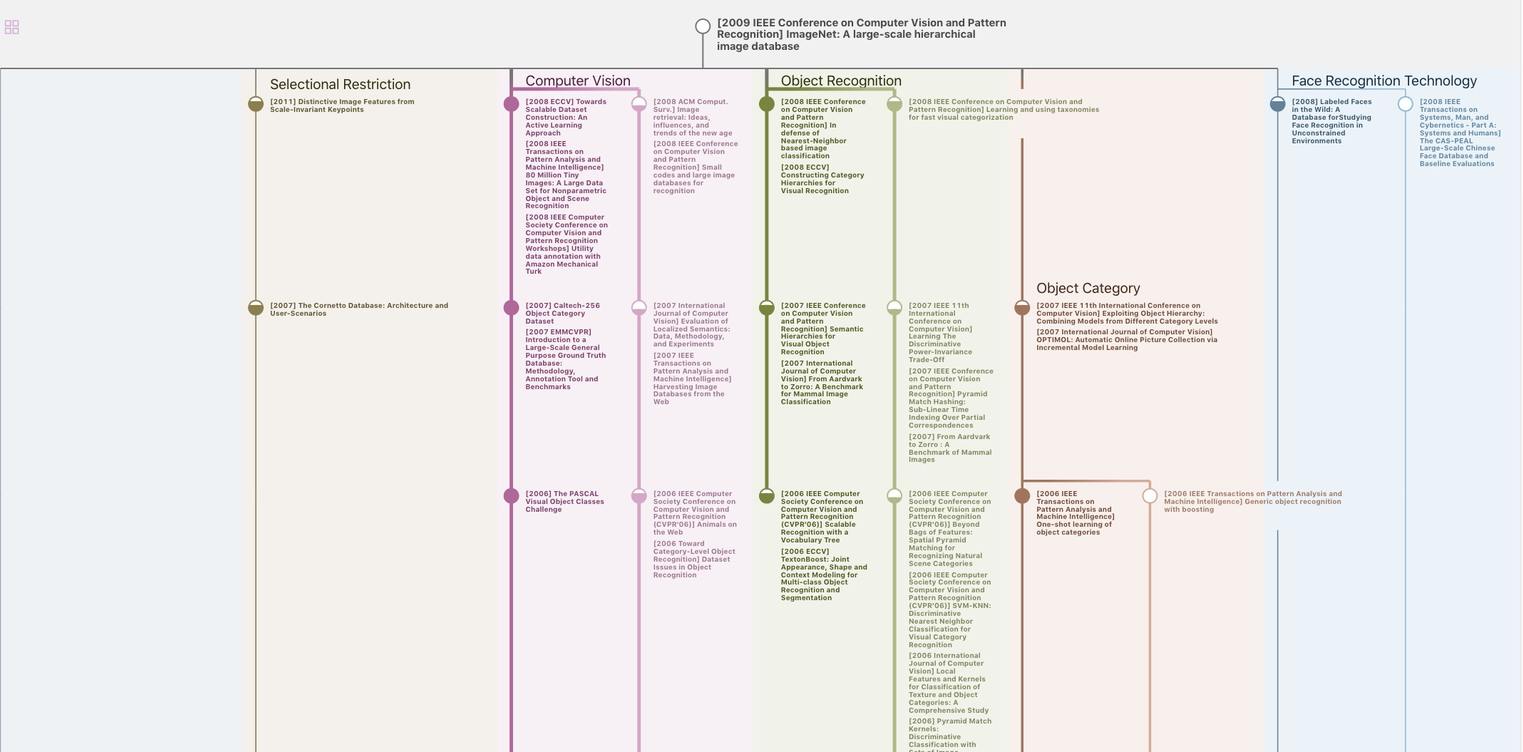
生成溯源树,研究论文发展脉络
Chat Paper
正在生成论文摘要