Microscopic parasite malaria classification using best feature selection based on generalized normal distribution optimization
PEERJ COMPUTER SCIENCE(2024)
摘要
Malaria disease can indeed be fatal if not identified and treated promptly. Due to advancements in the malaria diagnostic process, microscopy techniques are employed for blood cell analysis. Unfortunately, the diagnostic process of malaria via microscopy depends on microscopic skills. To overcome such issues, machine/deep learning algorithms can be proposed for more accurate and efficient detection of malaria. Therefore, a method is proposed for classifying malaria parasites that consist of three phases. The bilateral filter is applied to enhance image quality. After that shape-based and deep features are extracted. In shape-based pyramid histograms of oriented gradients (PHOG) features are derived with the dimension of N x 300. Deep features are derived from the residual network (ResNet)-50, and ResNet-18 at fully connected layers having the dimension of N x 1,000 respectively. The features obtained are fused serially, resulting in a dimensionality of N x 2,300. From this set, N x 498 features are chosen using the generalized normal distribution optimization (GNDO) method. The proposed method is accessed on a microscopic malarial parasite imaging dataset providing 99% classification accuracy which is better than as compared to recently published work.
更多查看译文
关键词
PHOG,GNDO,SVM,KNN,Ensemble,Malaria
AI 理解论文
溯源树
样例
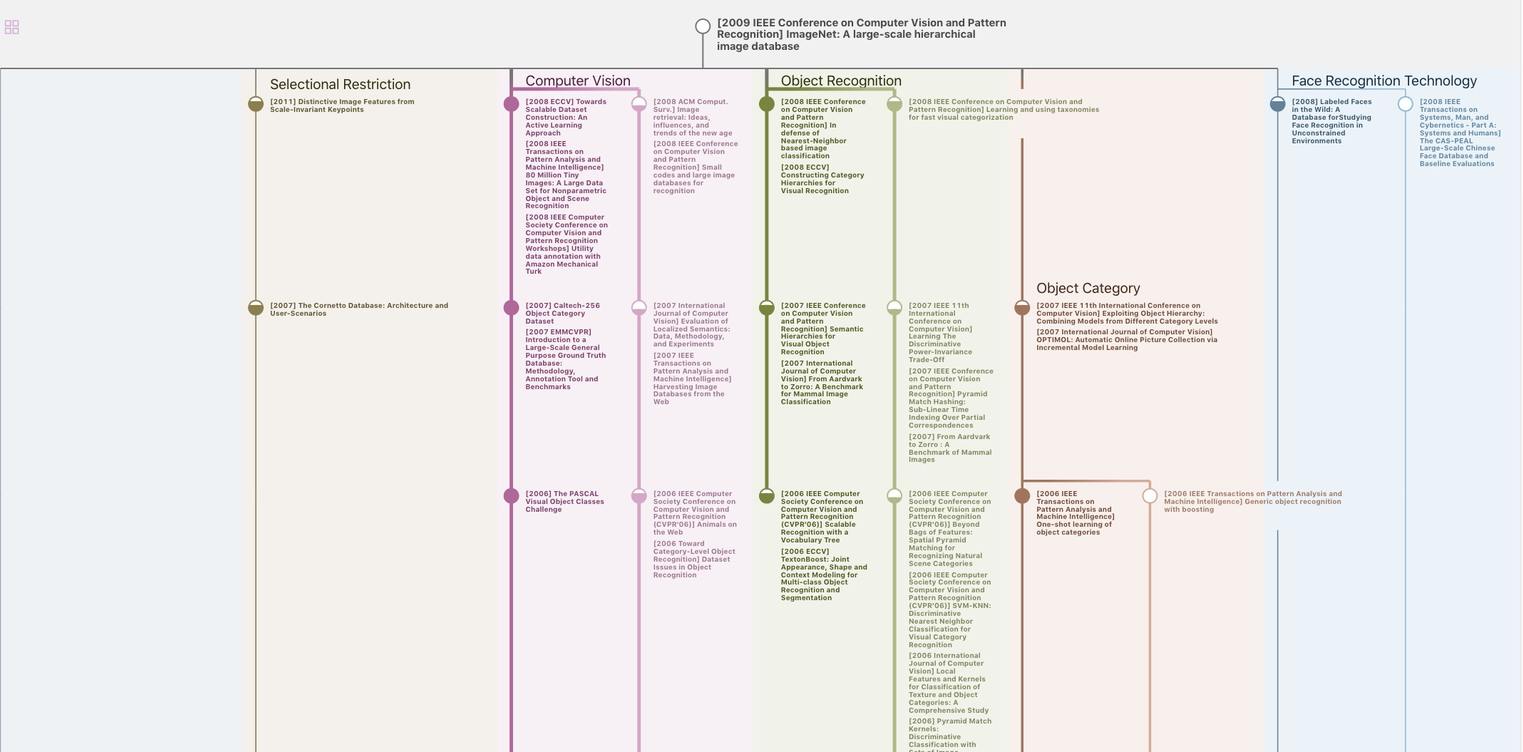
生成溯源树,研究论文发展脉络
Chat Paper
正在生成论文摘要