Fatigue life prediction driven by mesoscopic defect data
ENGINEERING APPLICATIONS OF ARTIFICIAL INTELLIGENCE(2024)
摘要
The research of predicting fatigue life through defect features is somewhat limited. In order to further study the influence of defect characteristics on fatigue life, a modification of Murakami model was proposed to calculate relative stress intensity factor, which is related with the influence of location, size, length-diameter ratio, flattening rate and adjacent interaction of defects comprehensively. A physics-informed neural network (PINN) was constructed with a physical information loss function transformed based on the relative stress intensity factor. Recognizing the challenges posed by inadequate training data, a mega trend diffusion technique based Gaussian distribution (G-MTD) is proposed to augment the dataset and maintain the distribution of the original data. By merging the G-MTD technique with the PINN, a comprehensive machine learning framework is established for the fatigue life prediction. The research findings demonstrate that this framework yields higher prediction accuracy and efficiency than the purely data-driven methods.
更多查看译文
关键词
Fatigue life,Mesoscopic defect,Augment dataset,Physics-informed neural network (PINN)
AI 理解论文
溯源树
样例
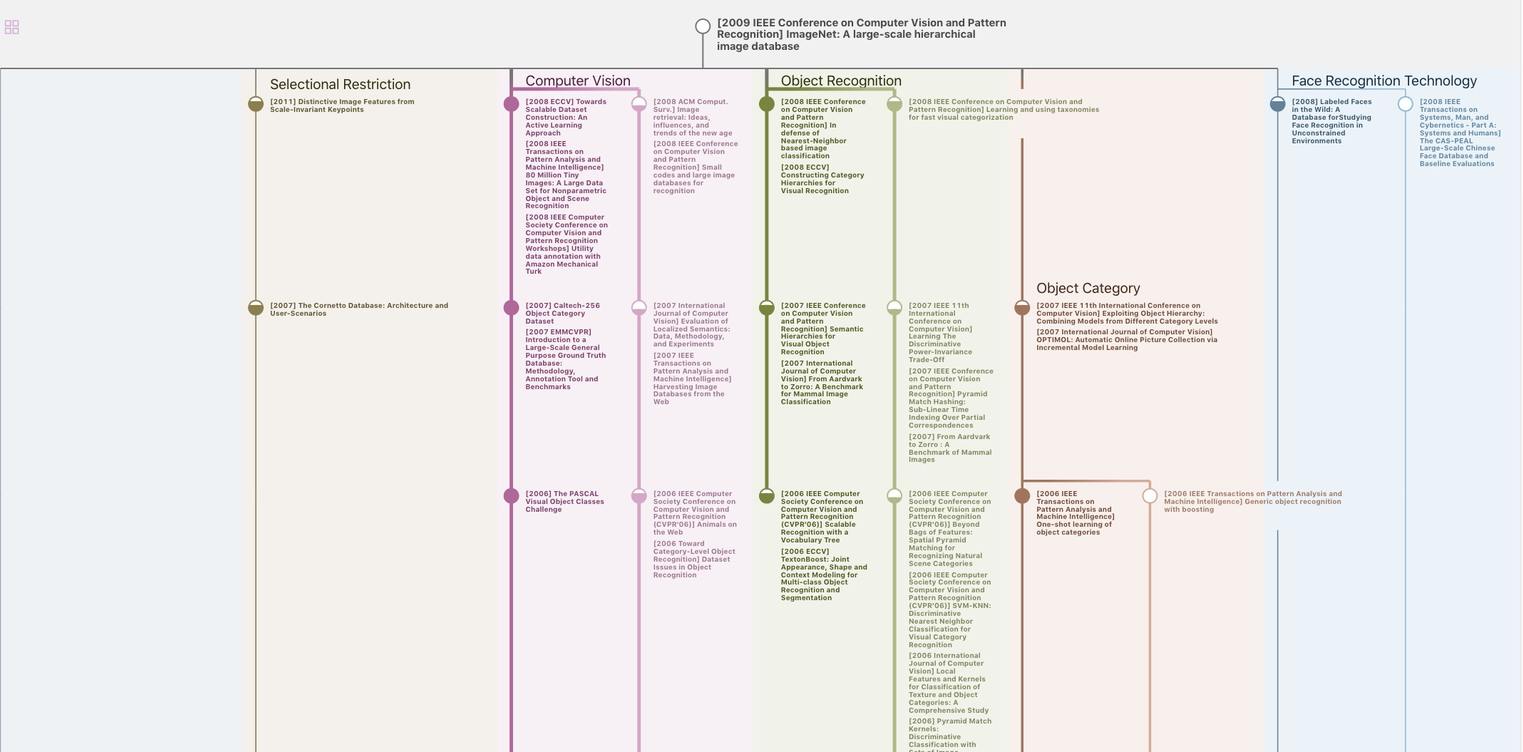
生成溯源树,研究论文发展脉络
Chat Paper
正在生成论文摘要