Hyperdimensional Computing Versus Convolutional Neural Network: Architecture, Performance Analysis, and Hardware Complexity.
2023 International Conference on Microelectronics (ICM)(2023)
摘要
The interest in brain-inspired computing architectures has been growing, particularly in the context of edge devices with constrained resources for executing cognitive functions. One such approach is hyperdimensional computing (HDC), a novel concept that draws inspiration from the large representation of human neuronal activity. HDC has demonstrated effectiveness in one-dimensional tasks, like text identification and activity recognition, offering advantages in power consumption and response time over convolutional neural networks (CNNs). This paper compares HDC and CNN regarding architecture, accuracy, and hardware complexity, explicitly focusing on image classification tasks. Our findings indicate that CNNs generally outperform HDC in two-dimensional tasks but require significantly more computational resources. In contrast, HDC offers adequate results using just 16% of the data needed for training. Additionally, experiments conducted using a Raspberry Pi 4 show that HDC can enhance inference speed and energy efficiency by approximately 2.5 times relative to CNNs.
更多查看译文
关键词
Hyperdimensional computing,Convolutional neural networks,Encoding and learning,Image classification
AI 理解论文
溯源树
样例
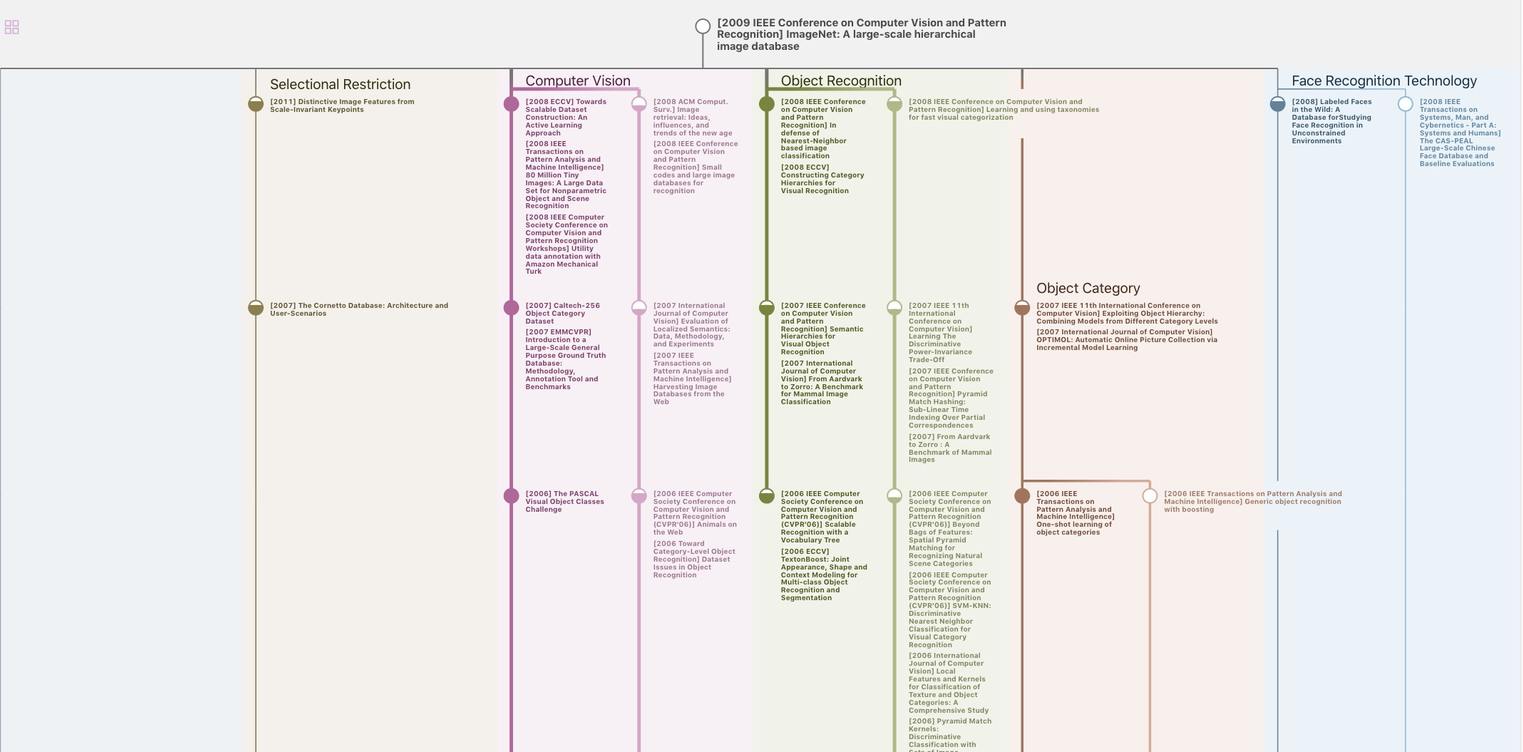
生成溯源树,研究论文发展脉络
Chat Paper
正在生成论文摘要