Involving Distinguished Temporal Graph Convolutional Networks for Skeleton-Based Temporal Action Segmentation
IEEE TRANSACTIONS ON CIRCUITS AND SYSTEMS FOR VIDEO TECHNOLOGY(2024)
摘要
For RGB-based temporal action segmentation (TAS), excellent methods that capture frame-level features have achieved remarkable performance. However, for motion-centered TAS, it is still challenging for existing methods that ignore the extraction of spatial features of joints. In addition, inaccurate action boundaries caused by the frames of similar motion destroy the integrity of the action segments. To alleviate the issues, an end-to-end Involving Distinguished Temporal Graph Convolutional Networks called IDT-GCN is proposed. First, we construct an enhanced spatial graph structure that adaptively captures the similar and differential dependencies between joints in a single topology through learning two independent correlation modeling functions. Then, the proposed Involving Distinguished Graph Convolutional (ID-GC) models the spatial correlations of different actions in a video by using multiple enhanced topologies on the corresponding channels. Furthermore, we design a generic modeling temporal action regression network, termed Temporal Segment Regression (TSR), to extract segmented encoding features and action boundary representations by modeling action sequences. Combining them with label smoothing modules, we develop powerful spatial-temporal graph convolutional networks (IDT-GCN) for fine-grained TAS, which notably outperforms state-of-the-art methods on the MCFS-22 and MCFS-130 datasets. Adding TSR to TCN-based baseline methods achieves competitive performance compared with the state-of-the-art transformer-based methods on RGB-based datasets, i.e., Breakfast and 50Salads. Further experimental results on the action recognition task verify the superiority of the enhanced spatial graph structure over the previous graph convolutional networks.
更多查看译文
关键词
Feature extraction,Motion segmentation,Correlation,Convolution,Topology,Convolutional neural networks,Solid modeling,Skeleton-based temporal action segmentation,enhanced spatial graph structure,segmented encoding
AI 理解论文
溯源树
样例
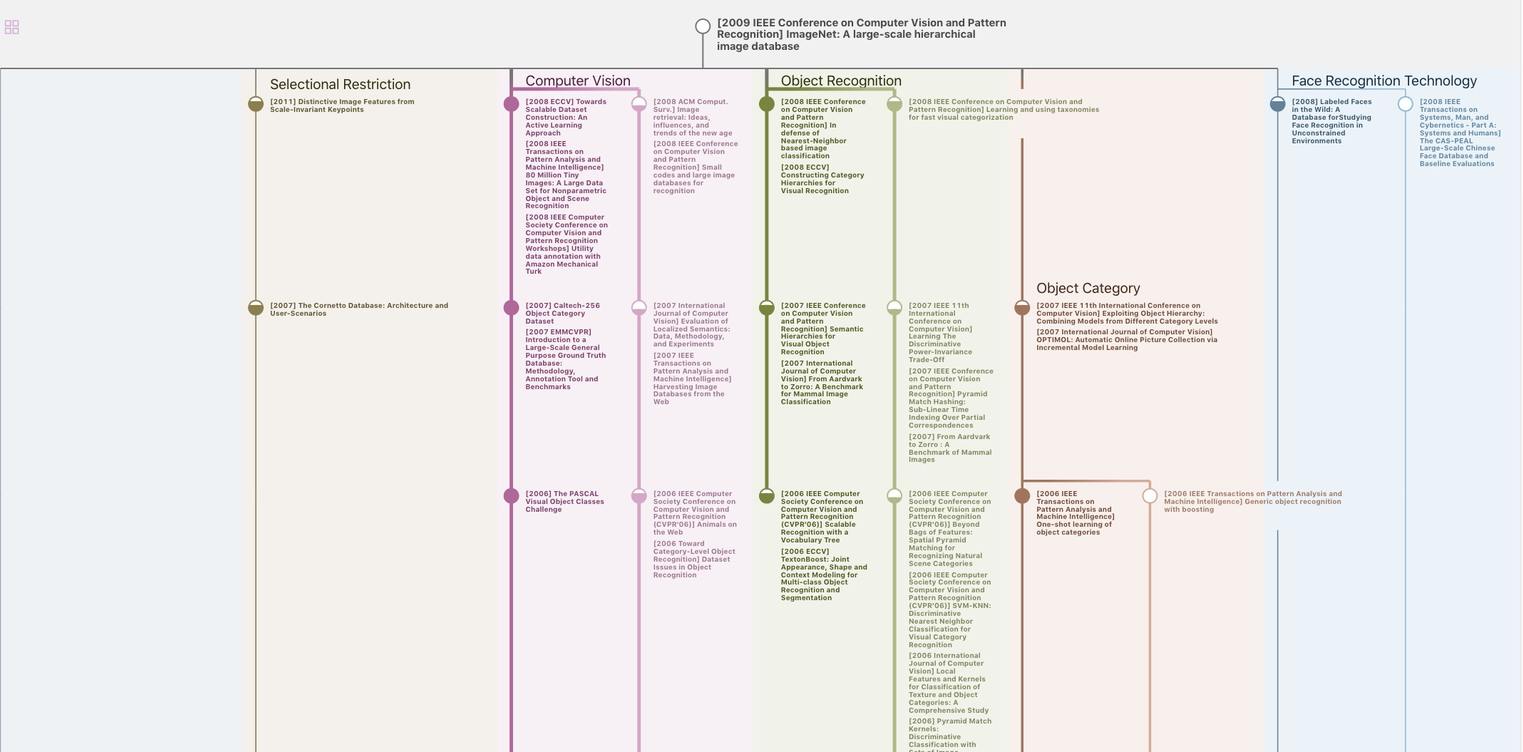
生成溯源树,研究论文发展脉络
Chat Paper
正在生成论文摘要