FPCB Surface Defect Detection Using Multiscale Spectral-Spatial Features Fusion
IEEE TRANSACTIONS ON COMPONENTS PACKAGING AND MANUFACTURING TECHNOLOGY(2023)
摘要
Recently, with the widespread application of flexible printed circuit boards (FPCBs) in smart electronic devices, FPCB surface defect detection has become an increasingly critical issue. In this study, a novel convolutional neural network framework based on transfer learning and multiscale spectral-spatial feature fusion (FPCB-Det) is proposed to detect FPCB surface defects. The proposed framework comprises a classification network (FPCB-ClaNet) and a localization network (FPCB-LocNet). Specifically, data augmentation (DA), class-balanced (CB) sampling, weight decay, and an attention mechanism are applied to enhance the performance of FPCB-ClaNet, and FPCB-LocNet uses 3-D convolution kernels with different sizes to jointly extract spectral-spatial features and achieves pixel-level segmentation of FPCB hyperspectral images (HSIs). The experimental results show that the classification accuracy of FPCB-ClaNet is as high as 97.84%, and the segmentation accuracy of FPCB-LocNet is as high as 97.86% compared with traditional image segmentation methods. The proposed FPCB-Det network can be applied to the actual production of FPCBs.
更多查看译文
关键词
Convolutional neural network,data imbalance,flexible printed circuit board (FPCB),hyperspectral image (HSI),surface defect detection
AI 理解论文
溯源树
样例
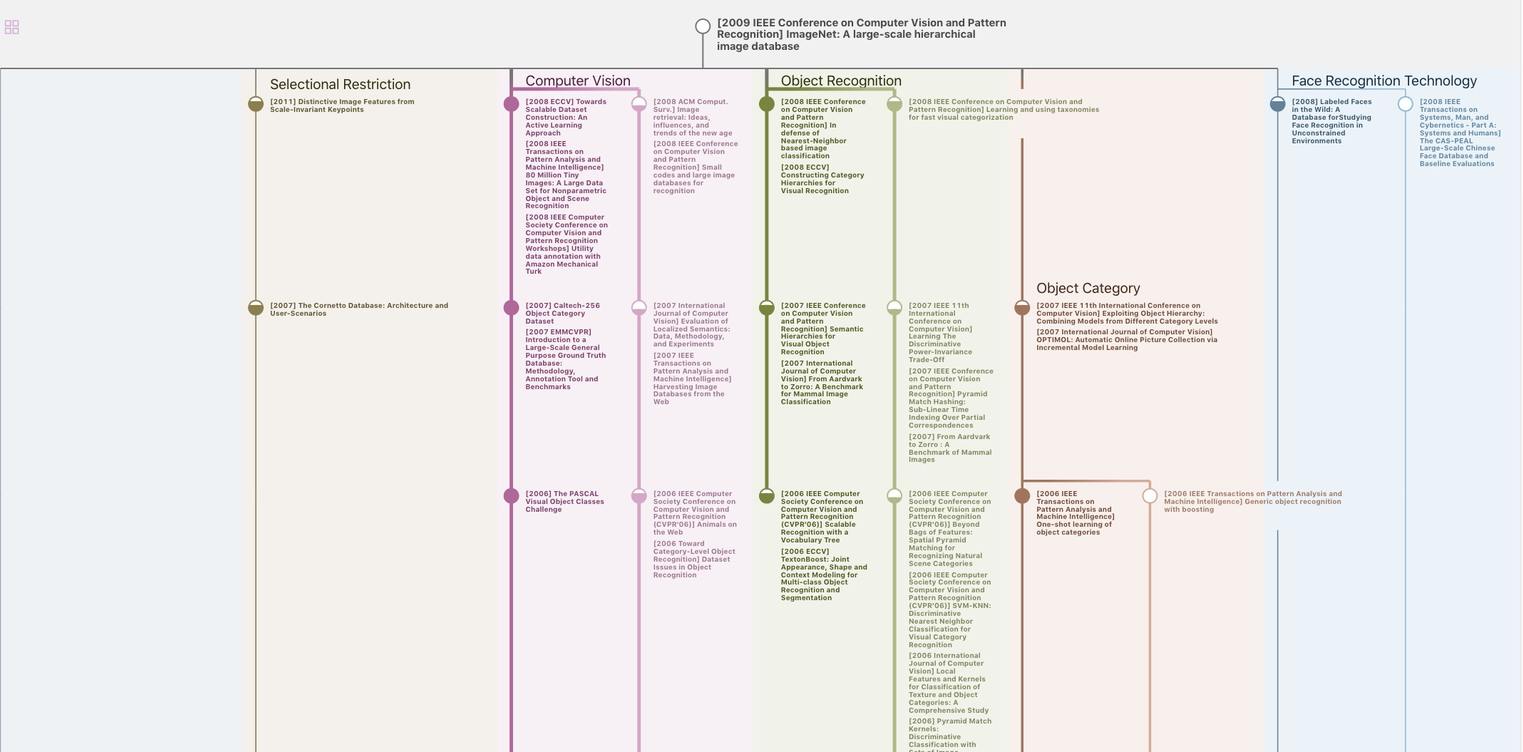
生成溯源树,研究论文发展脉络
Chat Paper
正在生成论文摘要