A deep-neural network potential to study transformation-induced plasticity in zirconia
JOURNAL OF THE EUROPEAN CERAMIC SOCIETY(2024)
摘要
Zirconia (ZrO2) ceramics uniquely exhibit transformation-induced plasticity, allowing plastic deformation prior to failure, setting them apart from most other ceramics. However, our understanding of ZrO2 plasticity is hindered by the challenge of simulating stress-induced atomic-scale phase transformations, owing to lack of an efficient interatomic potential accurately representing polymorphism and phase changes in ZrO2. In this work, we introduce a novel deep neural network interatomic potential, DP-ZrO2, constructed using a concurrent-learning approach. DP-ZrO2 reproduces properties of various ZrO2 phases, matching their phase diagrams as well as transformation pathways with accuracy comparable to ab initio density functional theory. Leveraging DP-ZrO2, we conducted molecular dynamics simulations of temperature-induced interphase boundary migration and nanocompression. These simulations demonstrate the potential's efficiency and applicability in studying deformation microstructures involving phase transformations in ZrO2. Our approach opens the door to large-scale simulations under complex loading conditions, which will shed light on the conditions favouring ZrO2 transformation-induced plasticity.
更多查看译文
关键词
Zirconia,Machine learning potential,Phase transformation,Molecular dynamics,Plasticity
AI 理解论文
溯源树
样例
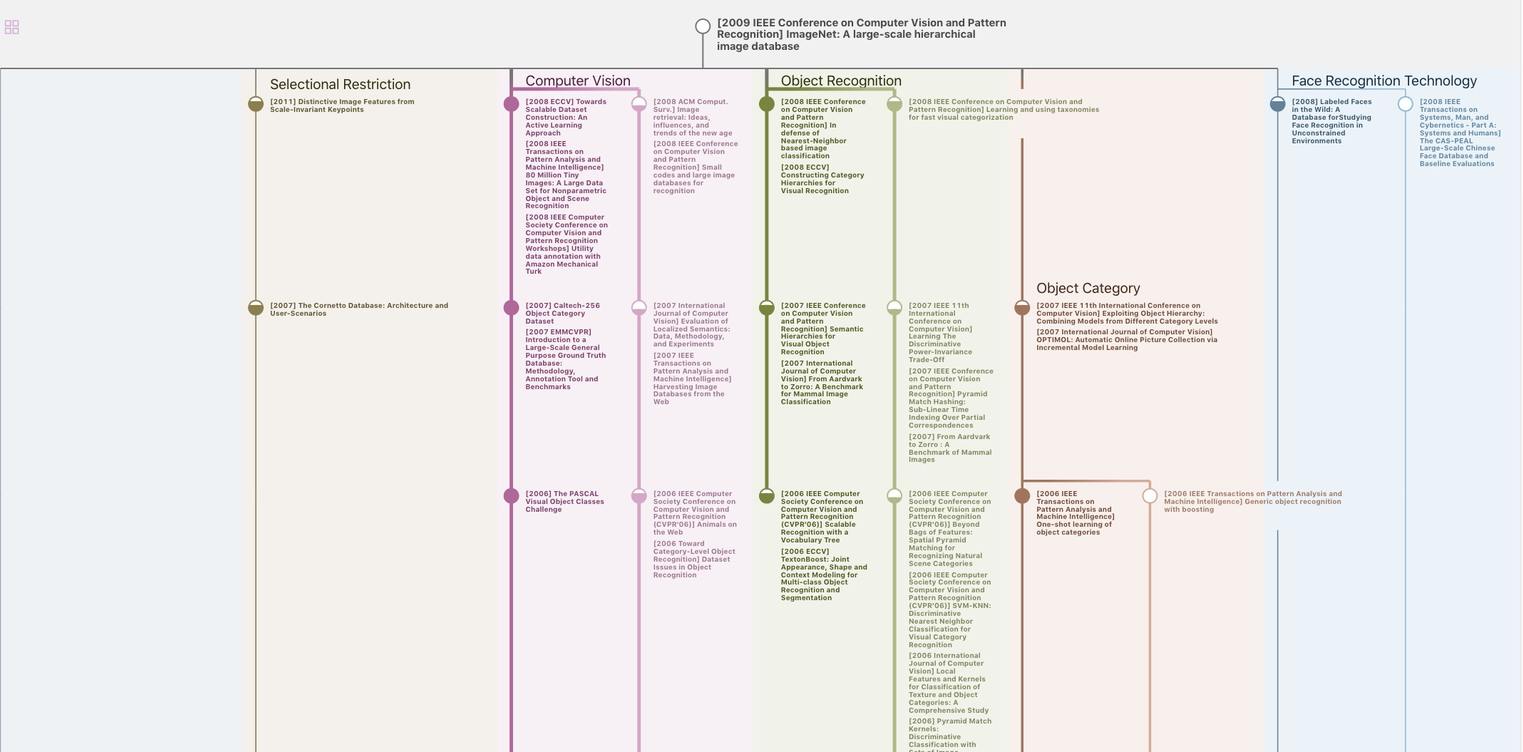
生成溯源树,研究论文发展脉络
Chat Paper
正在生成论文摘要