Simulation-Trained Neural Networks for Automatable Crack Detection in Magnetic Field Images
Journal of Nondestructive Evaluation(2024)
摘要
Magnetic field measurements play a vital role in various industries, particularly in the detection of cracks using magnetic field images, also known as magnetic field leakage testing. This paper presents an approach to automate the extraction of crack signals in magnetic field imaging by using neural networks. The proposed method relies on simulation-based training using the lightweight Python library Magpylib to calculate the three-dimensional static magnetic field of permanent magnets with surface defects. This approach has numerous advantages. It allows control of training data set variance by tuning simulation input parameters such as sample magnetization, measurement parameters, and defect properties to cover a wide range of cracks in size and position. Starting data acquisition before system operation allows investigating potential changes in sample shape or measurement parameters. Importantly, simulation-based data generation eliminates the need for physical measurements, leading to significant time savings. The study presents and discusses results obtained on two different ferromagnetic samples with surface cracks, a hollow cylinder and a steel sheet.
更多查看译文
关键词
Crack detection,Image processing,Magnetic field imaging,Neural networks,Object detection
AI 理解论文
溯源树
样例
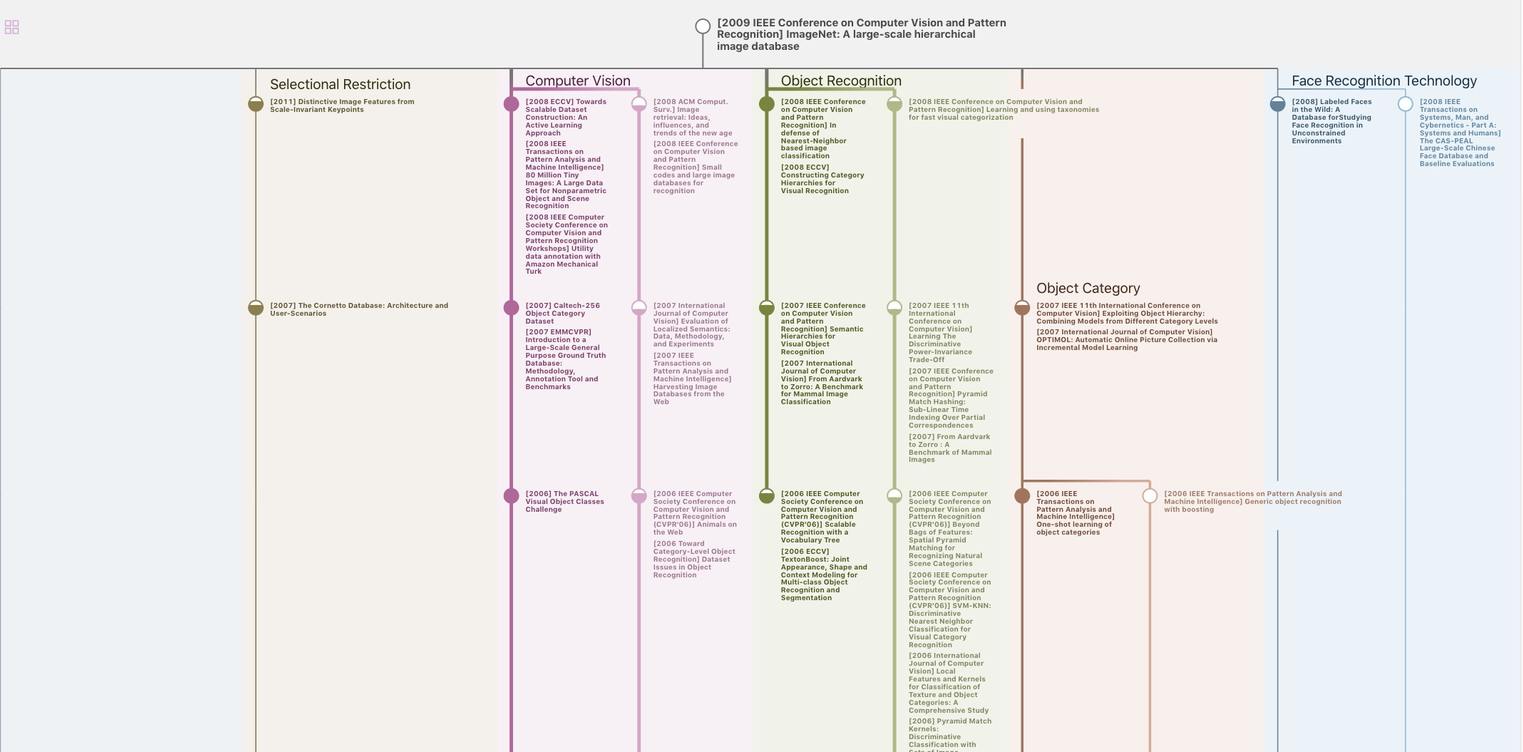
生成溯源树,研究论文发展脉络
Chat Paper
正在生成论文摘要