3D vision technologies for a self-developed structural external crack damage recognition robot
AUTOMATION IN CONSTRUCTION(2024)
摘要
Persistent cracking and progressive damage can weaken the operational performance of structures such as bridges, dams, and concrete buildings. Consequently, research into automated, high-precision crack detection methods remains pivotal within the realm of structural health monitoring (SHM). Presently, scholars predominantly rely on two-dimensional (2D) image-based algorithms for crack detection. However, these methods commonly struggle to accurately locate the three-dimensional (3D) coordinates of cracks on large structures and to extract the 3D contours of cracks. To address this challenge, this study proposes an automated 3D crack detection system for structures based on high-precision Light Detection and Ranging (LiDAR) and camera fusion. Firstly, precise registration of images and LiDAR point clouds was achieved through accurate extrinsic calibration of the sensors. Secondly, the lightweight MobileNetV2_DeepLabV3 crack semantic segmentation network was employed to detect and locate cracks. Finally, by automatically guiding the robotic arm, an industry-standard depth camera was able to capture high-precision 3D information about the crack at close observation points. Compared with the existing studies, this study emphasizes the extraction of high-precision 3D crack features and verifies the validity of the method by comparing the measurement results with those of the traditional method, demonstrating a remarkable measurement accuracy reaching sub-millimeter levels (0.1 mm). Moreover, the study introduces a comprehensive hardware platform and algorithmic framework, offering pioneering theoretical methodologies and replicable equipment references for automated surveillance and monitoring systems dedicated to structural health.
更多查看译文
关键词
Computer vision,3D crack,Path planning,Submillimeter-level precision,LiDAR and depth camera fusion
AI 理解论文
溯源树
样例
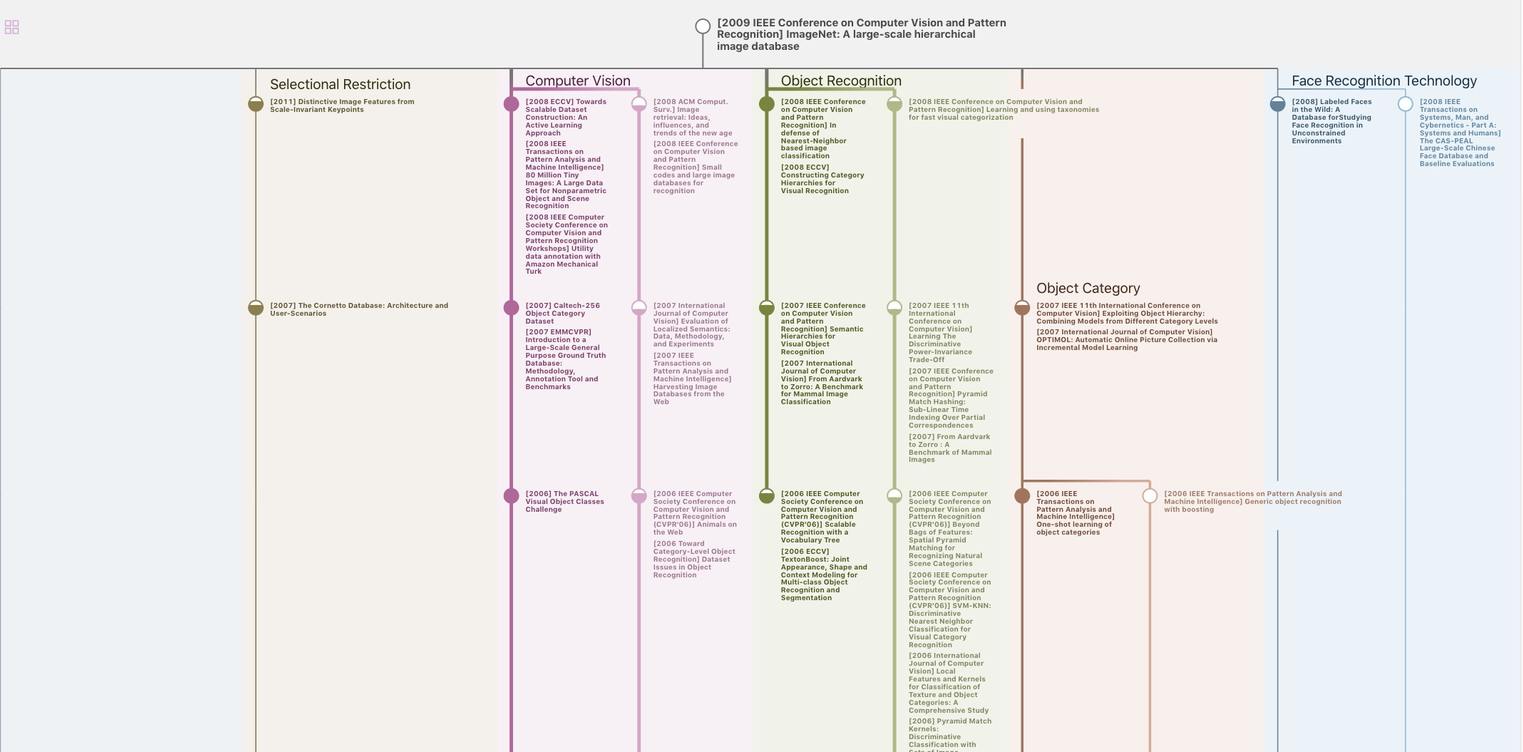
生成溯源树,研究论文发展脉络
Chat Paper
正在生成论文摘要