Learning dual disentangled representation with self-supervision for temporal knowledge graph reasoning
INFORMATION PROCESSING & MANAGEMENT(2024)
摘要
Temporal knowledge graph (TKG) reasoning aims to infer the missing links from the massive historical facts. One of the big issues is that how to model the entity evolution from both the local and especially global perspectives. The primary temporal dependency models often fail to disentangle both perspectives due to the lack explicit annotations to distinguish the boundary of these two representations. To address these limitations, we propose a contrastive learning framework to Disentangle Local and Global perspectives for TKG Reasoning with selfsupervision framework (DLGR). Our proposed DLGR can jointly utilize the local and global perspectives on two separate graphs and disentangle them in a self -supervised manner. Firstly, we construct a temporal subgraph and a temporal unified graph to effectively learn the local and global perspective representations, respectively. Second, we extract proxies regarding the different neighbors as pseudo labels to supervise the local and global disentanglement in a contrastive manner. Finally, we adaptively fuse the learned two perspective representations for TKG reasoning. The empirical results show that our DLGR significantly outperforms other baselines (e.g., compared to the strong baseline HGLS, our DLGR achieves 4.3%, 3.4%, 1.6% and 1.1% improvements on ICEWS14, ICEWS18, YAGO and WIKI using MRR).
更多查看译文
关键词
Temporal knowledge graph,Knowledge graph reasoning,Disentangled representation,Self-supervised learning
AI 理解论文
溯源树
样例
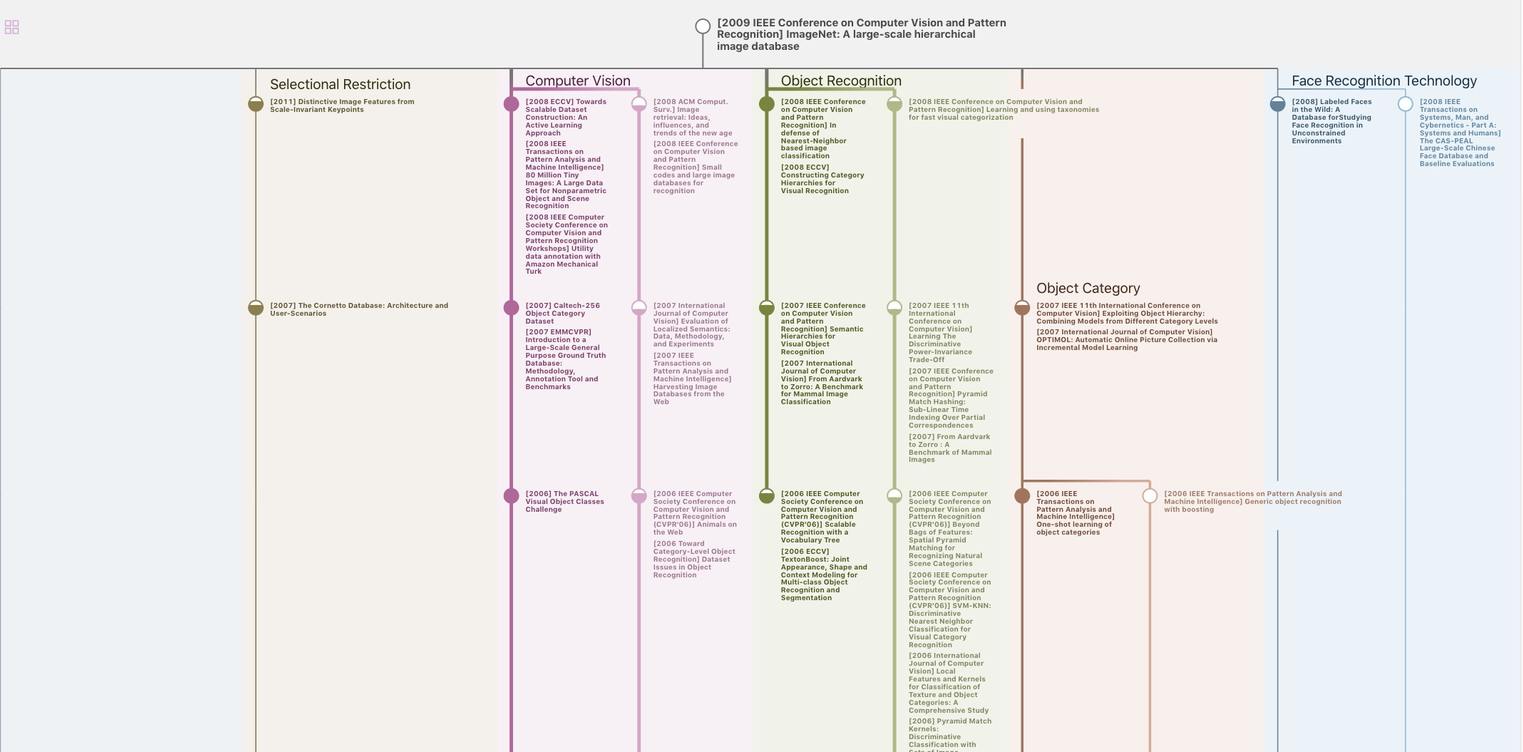
生成溯源树,研究论文发展脉络
Chat Paper
正在生成论文摘要