A predictive model for daylight performance based on multimodal generative adversarial networks at the early design stage
ENERGY AND BUILDINGS(2024)
摘要
In the early building design stage, machine learning-based surrogate models demonstrate significant advantages in rapidly evaluating design performance compared to conventional simulation software. However, due to network limitations, current models struggle to incorporate comprehensive building information, leading to undesired generalizability in adapting to design variations. This study proposes a multimodal Generative Adversarial Network (GAN)-based surrogate model, representing building features with combined multimodal data of images and vectors. Geometric features on the plan and facade are translated into grayscale maps, indicating the potential for daylight reception from each viewpoint, while material properties and weather features are extracted as vectors, such as reflectance, transmittance, sun position and sky conditions. To satisfy the demands of multimodal inputs, the multimodal feature fusion block, the vector-based feature encoding block, and the feature reinforcement block are introduced. These components aim to promote the deep integration of information from different modalities, balance the influences of features across different dimensional scales, and ensure the integrity of the information. The model's validity was verified on the RPLAN database, predicting illumination distribution at the whole-building hierarchy instead of the single-room hierarchy. The results indicate that the multimodal GAN has a Mean Squared Error of 8.129, a Mean Absolute Percentage Error of 0.135, and a Structural Similarity Index of 0.907 on the test set. Meanwhile, this approach saved 73.03% of computing time compared to simulation methods. Boasting strengths in generalizability, speed, and accuracy, the model provides effective support for daylight organization in the early stage of building design, without being confined by variable design scenarios.
更多查看译文
关键词
Daylight prediction,Surrogate model,Generative Adversarial Networks,Multimodal model
AI 理解论文
溯源树
样例
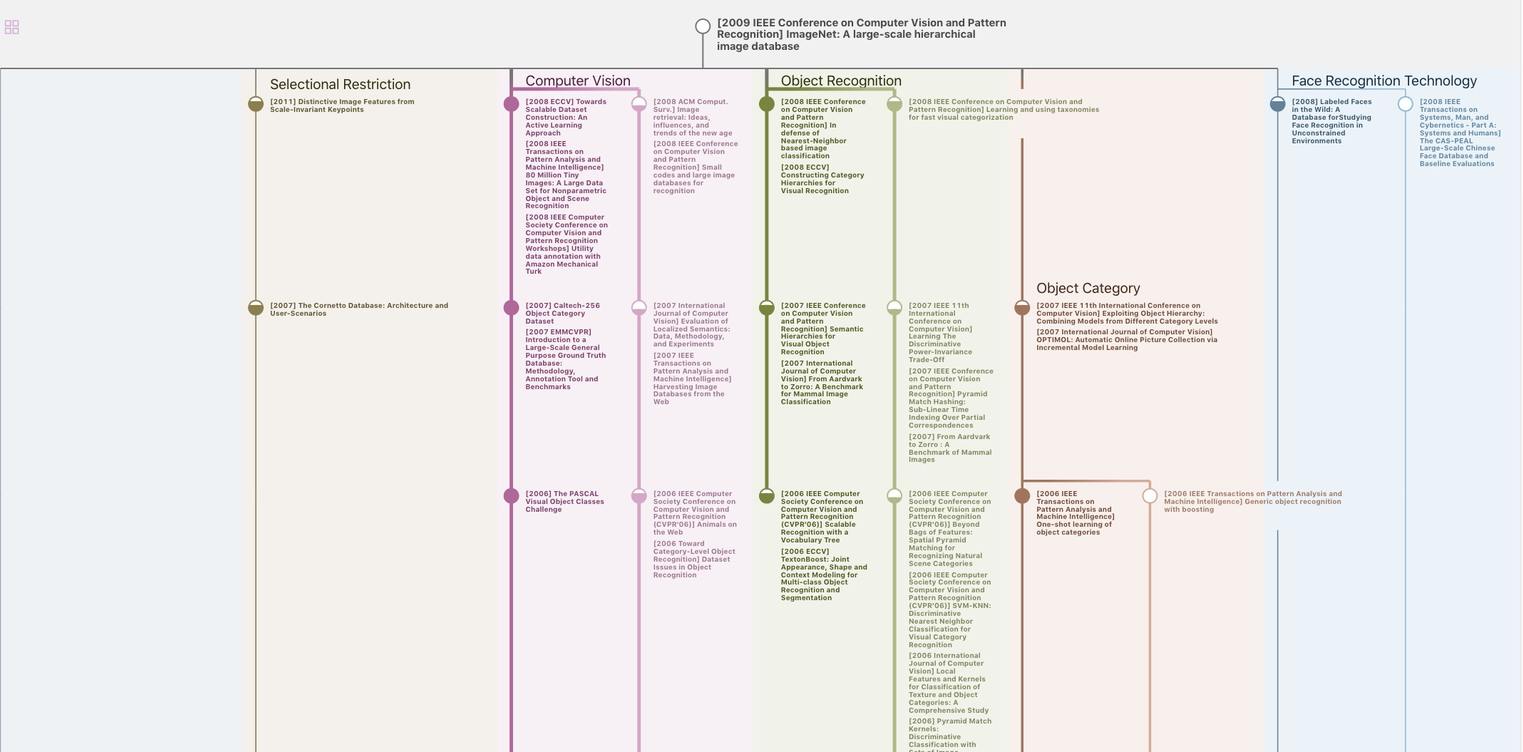
生成溯源树,研究论文发展脉络
Chat Paper
正在生成论文摘要