MFFDNet: Single Image Deraining via Dual-Channel Mixed Feature Fusion.
IEEE Transactions on Instrumentation and Measurement(2024)
摘要
Images captured by vision-based measurement tools often suffer from detail blurring, color distortion, and visibility degradation due to rain streaks in rainy weather. As a potential remedy, we develop a hybrid image deraining network called mixed feature fusion network for single-image deraining (MFFDNet), which artfully integrates local and global image features for better rain removal. The proposed MFFDNet makes three significant contributions. First, MFFDNet takes full advantage of convolutional neural network (CNN) and transformer to produce more discriminative features, improving the model’s deraining capacity. Second, we propose a novel local feature extraction module called the channel-spatial attention block (CSAB), which can reduce the interference of rain streaks by separating high-frequency and low-frequency information. Also, this module leverages the spatial attention mechanism to obtain better local features. Finally, we develop a prospective feature fusion module, which can produce local features with global characteristics and global features with local characteristics by fusing these two features. Comprehensive evaluations on four synthetic and two real-world datasets demonstrate that MFFDNet performs well in peak signal-to-noise ratio (PSNR), structural similarity (SSIM), natural image quality evaluator (NIQE), and blind/referenceless image spatial quality evaluator (BRISQUE) metrics. The source code is available at
https://github.com/taowenyin/MFFDNet
.
更多查看译文
关键词
Feature fusion,hybrid network,image deraining,joint learning,mixed feature fusion network for single-image deraining (MFFDNet)
AI 理解论文
溯源树
样例
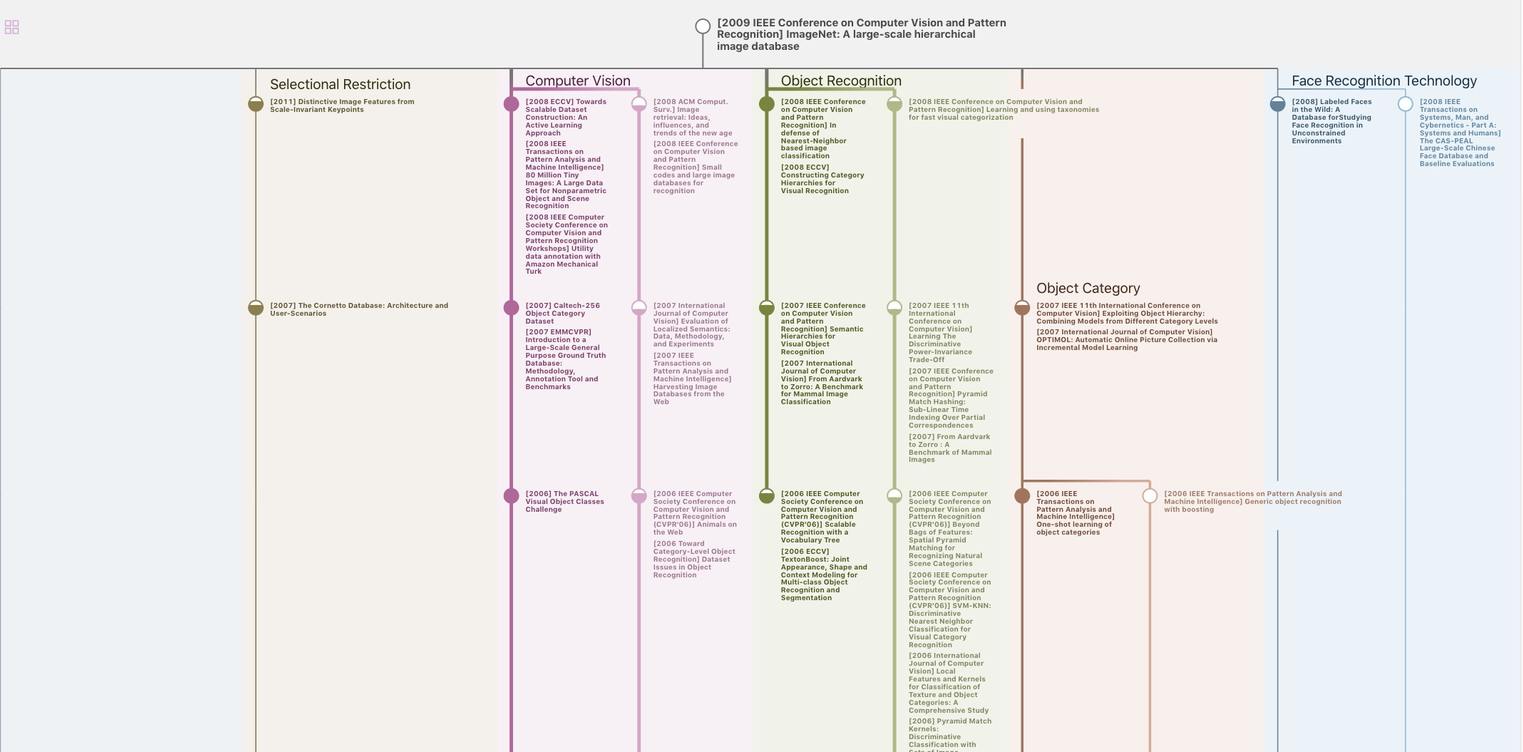
生成溯源树,研究论文发展脉络
Chat Paper
正在生成论文摘要