Digital twin-driven framework for fatigue life prediction of welded structures considering residual stress
INTERNATIONAL JOURNAL OF FATIGUE(2024)
摘要
The welding of steel generates substantial welding residual stress (WRS), which exerts a significant impact on the fatigue life of steel bridges. In this study, a physical model for calculating the fatigue crack growth (FCG) life of welded specimens in the WRS field is established based on the weight function method. Experimental data validates the reliability and precision of the proposed physical model. The impact of WRS on the fatigue life of structural components is scrutinized and analyzed. On this basis, a digital twin (DT) framework driven by a physical-data model is proposed to consider the inherent parameter uncertainty in FCG behavior within the WRS domain. A dynamic Bayesian network (DBN) is used to characterize the evolution characteristics of fatigue crack states over time in the digital space. Particle filter algorithm is used as DBN inference method. The results show that the calculation of the physical model is in good agreement with the experimental values. Neglecting the influence of WRS distribution may lead to an overestimation of fatigue life in welded structures. The DT framework can update uncertain parameters online and realize the accurate prediction of the FCG life in the WRS field.
更多查看译文
关键词
Fatigue crack growth,Welding residual stress,Weight function method,Digital twin,Dynamic Bayesian network
AI 理解论文
溯源树
样例
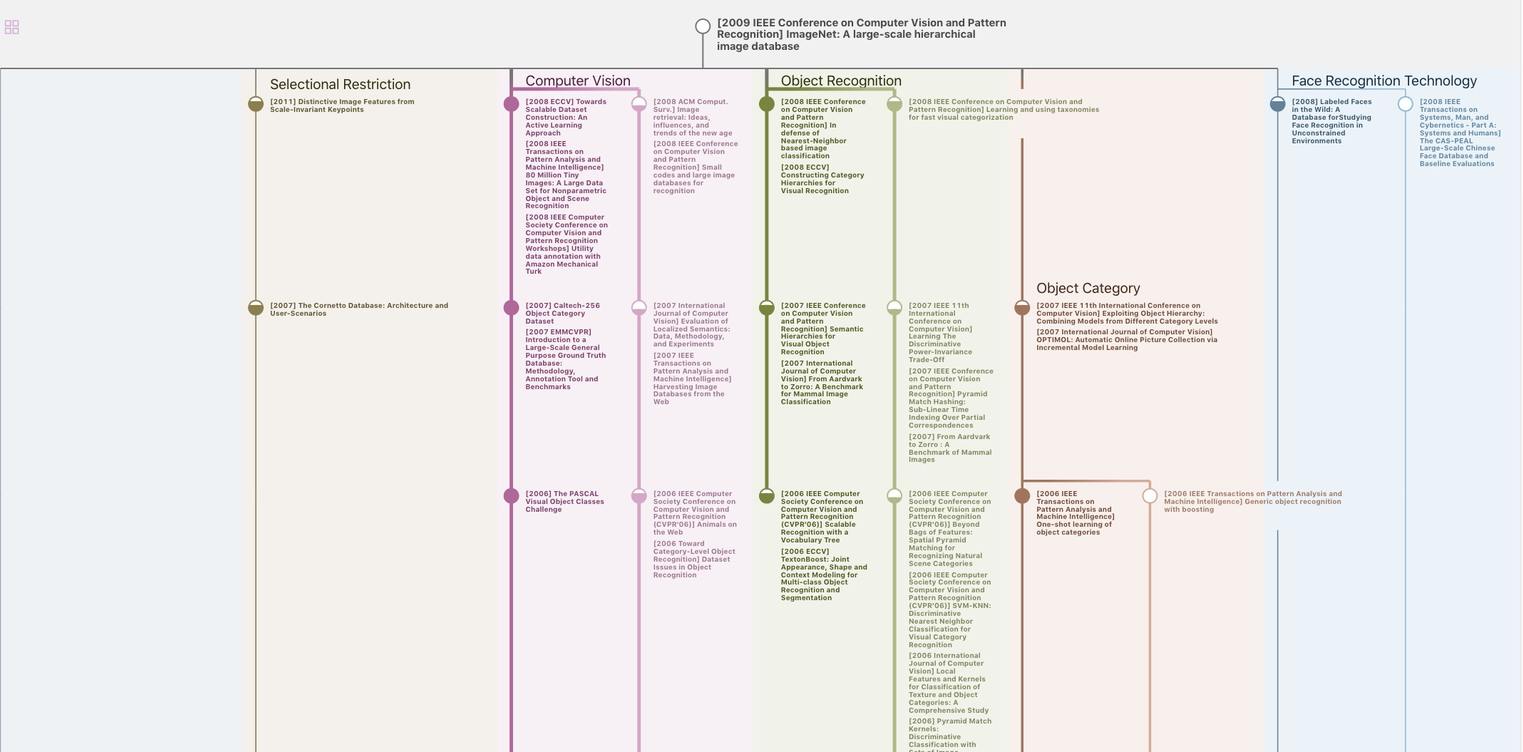
生成溯源树,研究论文发展脉络
Chat Paper
正在生成论文摘要