Enhancing the Quality and Reliability of Machine Learning Interatomic Potentials through Better Reporting Practices
JOURNAL OF PHYSICAL CHEMISTRY C(2024)
摘要
Recent developments in machine learning interatomic potentials (MLIPs) have empowered even nonexperts in machine learning to train MLIPs for accelerating materials simulations. However, reproducibility and independent evaluation of presented MLIP results is hindered by a lack of clear standards in current literature. In this Perspective, we aim to provide guidance on best practices for documenting MLIP use while walking the reader through the development and deployment of MLIPs including hardware and software requirements, generating training data, training models, validating predictions, and MLIP inference. We also suggest useful plotting practices and analyses to validate and boost confidence in the deployed models. Finally, we provide a step-by-step checklist for practitioners to use directly before publication to standardize the information to be reported. Overall, we hope that our work will encourage the reliable and reproducible use of these MLIPs, which will accelerate their ability to make a positive impact in various disciplines including materials science, chemistry, and biology, among others.
更多查看译文
AI 理解论文
溯源树
样例
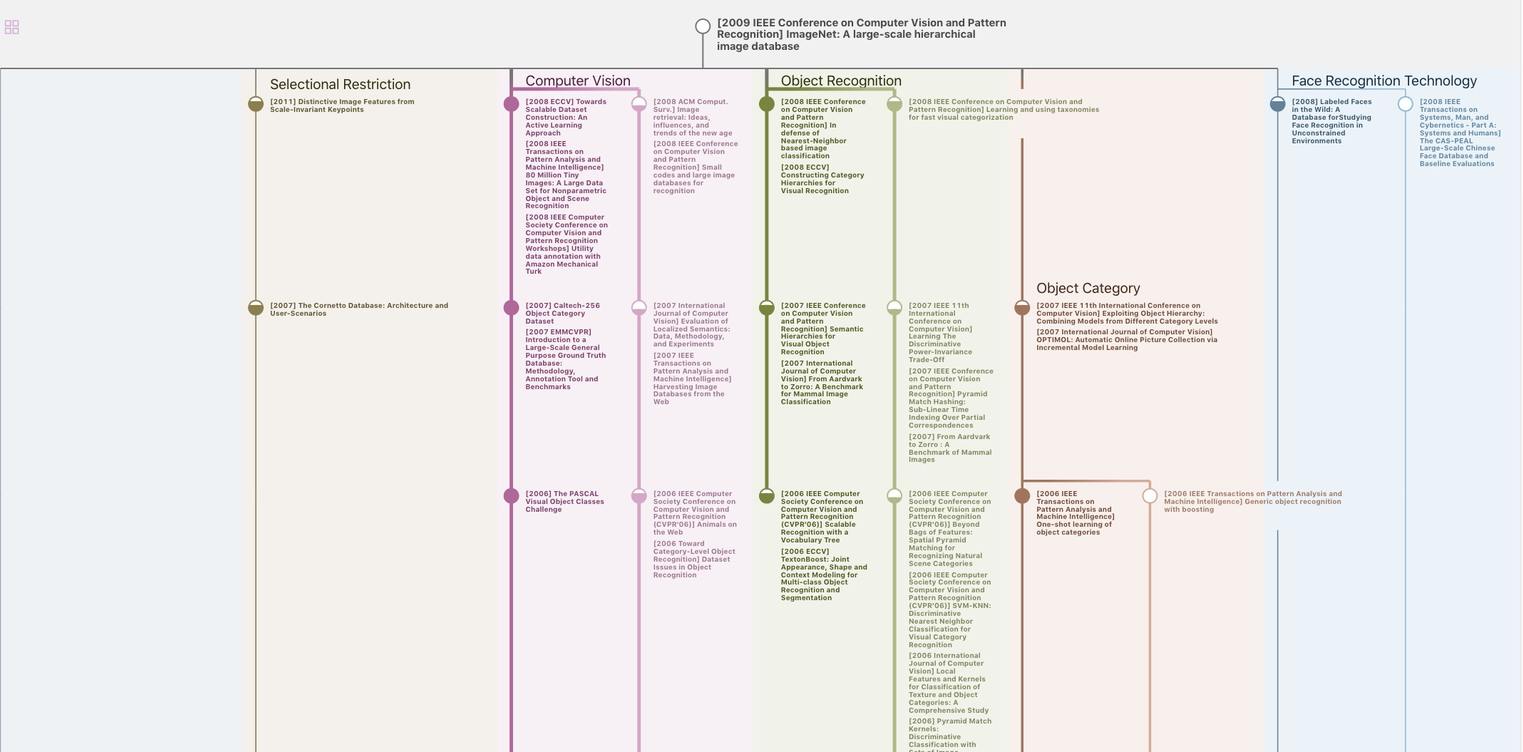
生成溯源树,研究论文发展脉络
Chat Paper
正在生成论文摘要