Preserving Image Properties Through Initializations in Diffusion Models
CoRR(2024)
摘要
Retail photography imposes specific requirements on images. For instance,
images may need uniform background colors, consistent model poses, centered
products, and consistent lighting. Minor deviations from these standards impact
a site's aesthetic appeal, making the images unsuitable for use. We show that
Stable Diffusion methods, as currently applied, do not respect these
requirements. The usual practice of training the denoiser with a very noisy
image and starting inference with a sample of pure noise leads to inconsistent
generated images during inference. This inconsistency occurs because it is easy
to tell the difference between samples of the training and inference
distributions. As a result, a network trained with centered retail product
images with uniform backgrounds generates images with erratic backgrounds. The
problem is easily fixed by initializing inference with samples from an
approximation of noisy images. However, in using such an approximation, the
joint distribution of text and noisy image at inference time still slightly
differs from that at training time. This discrepancy is corrected by training
the network with samples from the approximate noisy image distribution.
Extensive experiments on real application data show significant qualitative and
quantitative improvements in performance from adopting these procedures.
Finally, our procedure can interact well with other control-based methods to
further enhance the controllability of diffusion-based methods.
更多查看译文
关键词
Algorithms,Generative models for image,video,3D,etc.,Applications,Commercial / retail
AI 理解论文
溯源树
样例
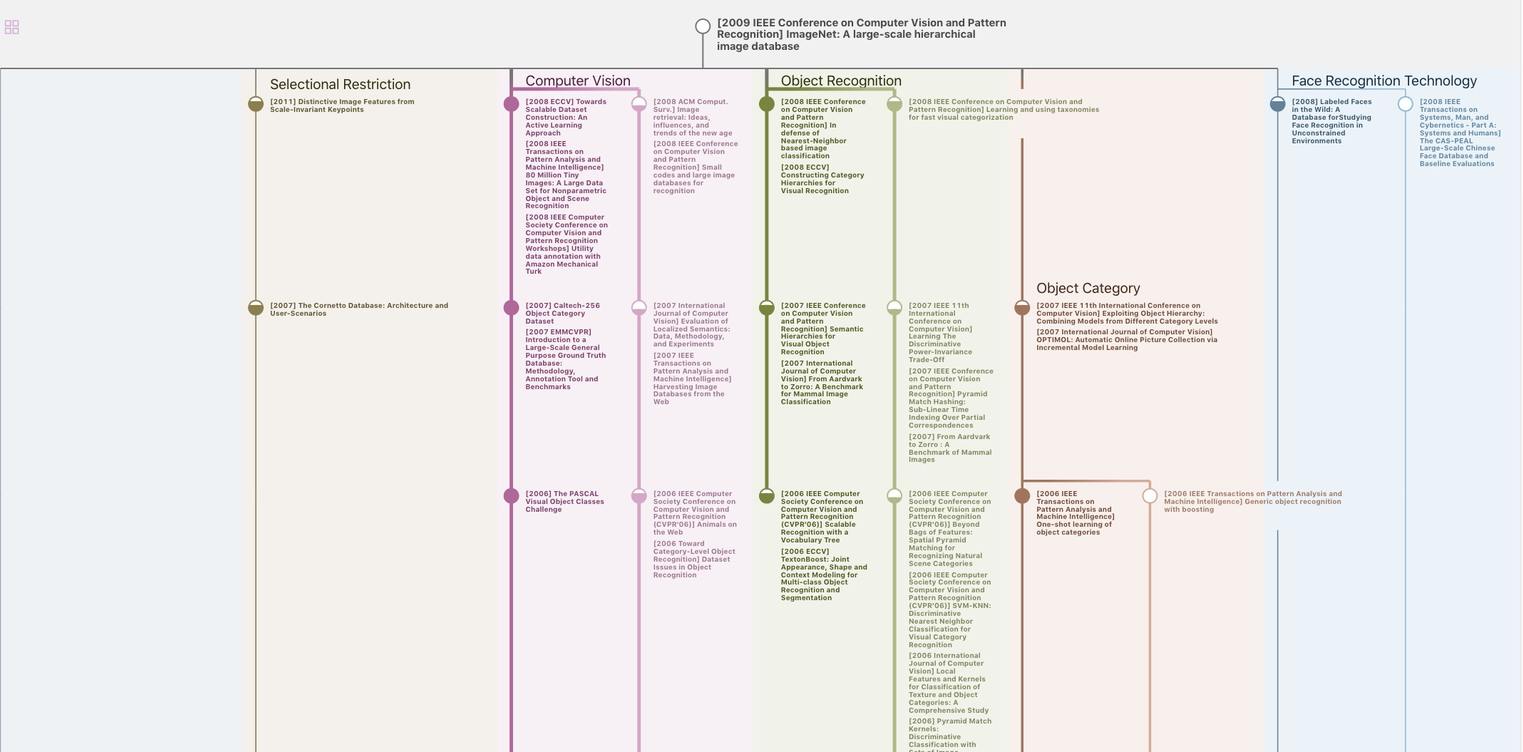
生成溯源树,研究论文发展脉络
Chat Paper
正在生成论文摘要