Unsupervised Object-Centric Learning From Multiple Unspecified Viewpoints.
IEEE transactions on pattern analysis and machine intelligence(2024)
摘要
Visual scenes are extremely diverse, not only because there are infinite possible combinations of objects and backgrounds but also because the observations of the same scene may vary greatly with the change of viewpoints. When observing a multi-object visual scene from multiple viewpoints, humans can perceive the scene compositionally from each viewpoint while achieving the so-called "object constancy" across different viewpoints, even though the exact viewpoints are untold. This ability is essential for humans to identify the same object while moving and to learn from vision efficiently. It is intriguing to design models that have a similar ability. In this article, we consider a novel problem of learning compositional scene representations from multiple unspecified (i.e., unknown and unrelated) viewpoints without using any supervision and propose a deep generative model which separates latent representations into a viewpoint-independent part and a viewpoint-dependent part to solve this problem. During the inference, latent representations are randomly initialized and iteratively updated by integrating the information in different viewpoints with neural networks. Experiments on several specifically designed synthetic datasets have shown that the proposed method can effectively learn from multiple unspecified viewpoints.
更多查看译文
关键词
Compositional scene representations,deep generative models,object constancy,object-centric learning,unsupervised learning,variational inference
AI 理解论文
溯源树
样例
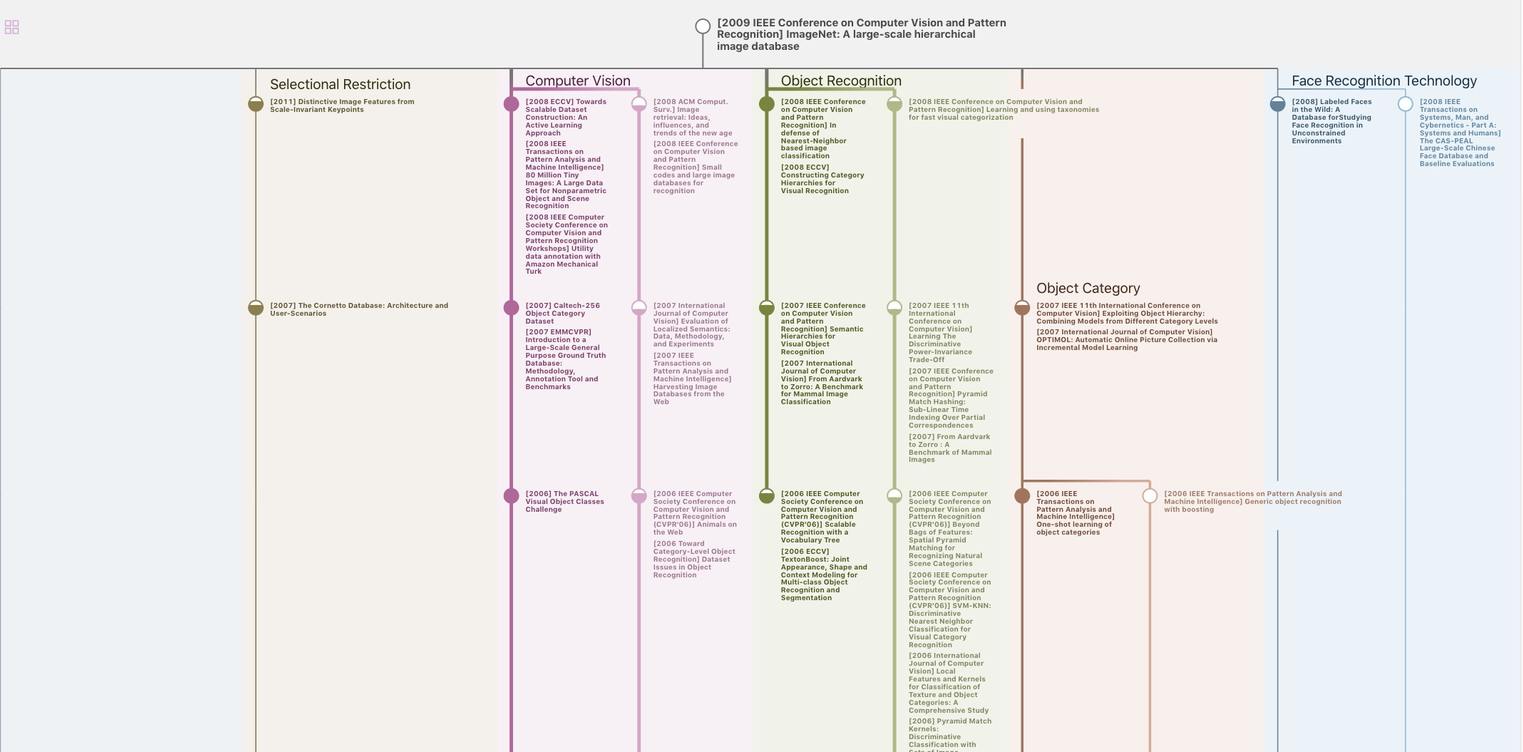
生成溯源树,研究论文发展脉络
Chat Paper
正在生成论文摘要