Multi-label feature selection with high-sparse personalized and low-redundancy shared common features
INFORMATION PROCESSING & MANAGEMENT(2024)
摘要
Prevalent multi-label feature selection (MLFS) approaches to obtain the most suitable feature subset by dealing with two issues, namely sparsity and redundancy. In this paper, we design an efficient Elastic net based high Sparse personalized and low Redundancy Feature Selection approach for multi-label data named ESRFS to address the two obstacles, i.e., low-sparse LASSOnorm leads to personalized features for each label while high redundancy I21 -norm explore shared common features for all labels in multi-label learning. These two problems impede the selection of high-quality features for classification. In comparison with previous MLFS approaches, ESRFS has two main superiority. First, ESRFS achieves higher sparse personalized features than LASSO -norm. Second, ESRFS can identify low redundancy shared common features with strong discrimination by introducing a novel regularization term. To effectively and efficiently identify the most optimal feature subset, an alternating-multiplier-based rule is introduced to optimize ESRFS. Experimental results on fifteen multi-label data sets show that ESRFS can achieve obvious superior performance compared to eight state -of -the -art MLFS approaches in 80%, 80%, 73.3%, 80%, 86.7%, 80% cases based on Hamming Loss, Zero-One Loss using MLkNN, Micro-F1 and Macro-F1 using SVM as well as Micro-F1 and Macro-F1 using 3NN perspectives.
更多查看译文
关键词
Multi-label learning,Feature selection,Sparse learning,Classification
AI 理解论文
溯源树
样例
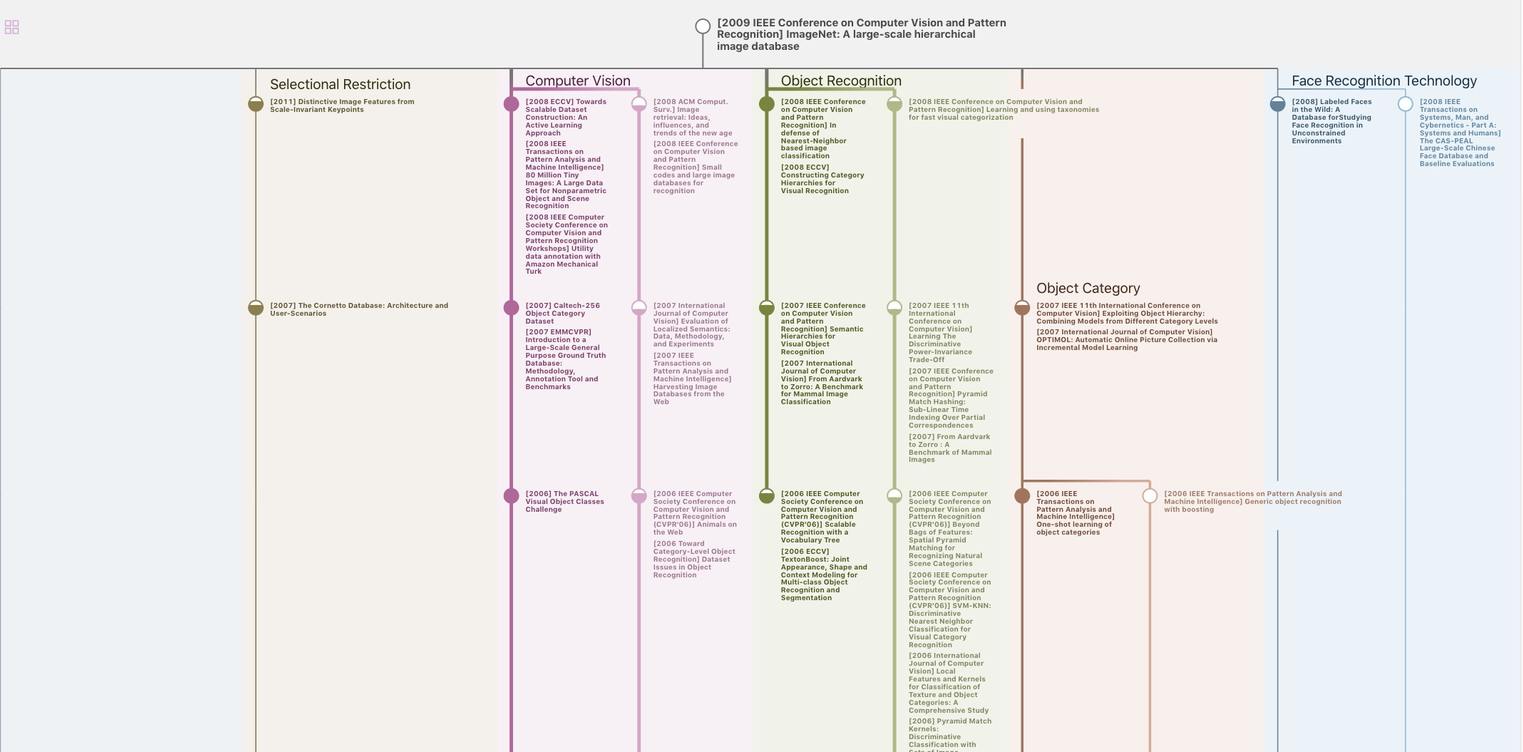
生成溯源树,研究论文发展脉络
Chat Paper
正在生成论文摘要